Unlearning with Fisher Masking
CoRR(2023)
摘要
Machine unlearning aims to revoke some training data after learning in response to requests from users, model developers, and administrators. Most previous methods are based on direct fine-tuning, which may neither remove data completely nor retain full performances on the remain data. In this work, we find that, by first masking some important parameters before fine-tuning, the performances of unlearning could be significantly improved. We propose a new masking strategy tailored to unlearning based on Fisher information. Experiments on various datasets and network structures show the effectiveness of the method: without any fine-tuning, the proposed Fisher masking could unlearn almost completely while maintaining most of the performance on the remain data. It also exhibits stronger stability compared to other unlearning baselines
更多查看译文
关键词
fisher masking
AI 理解论文
溯源树
样例
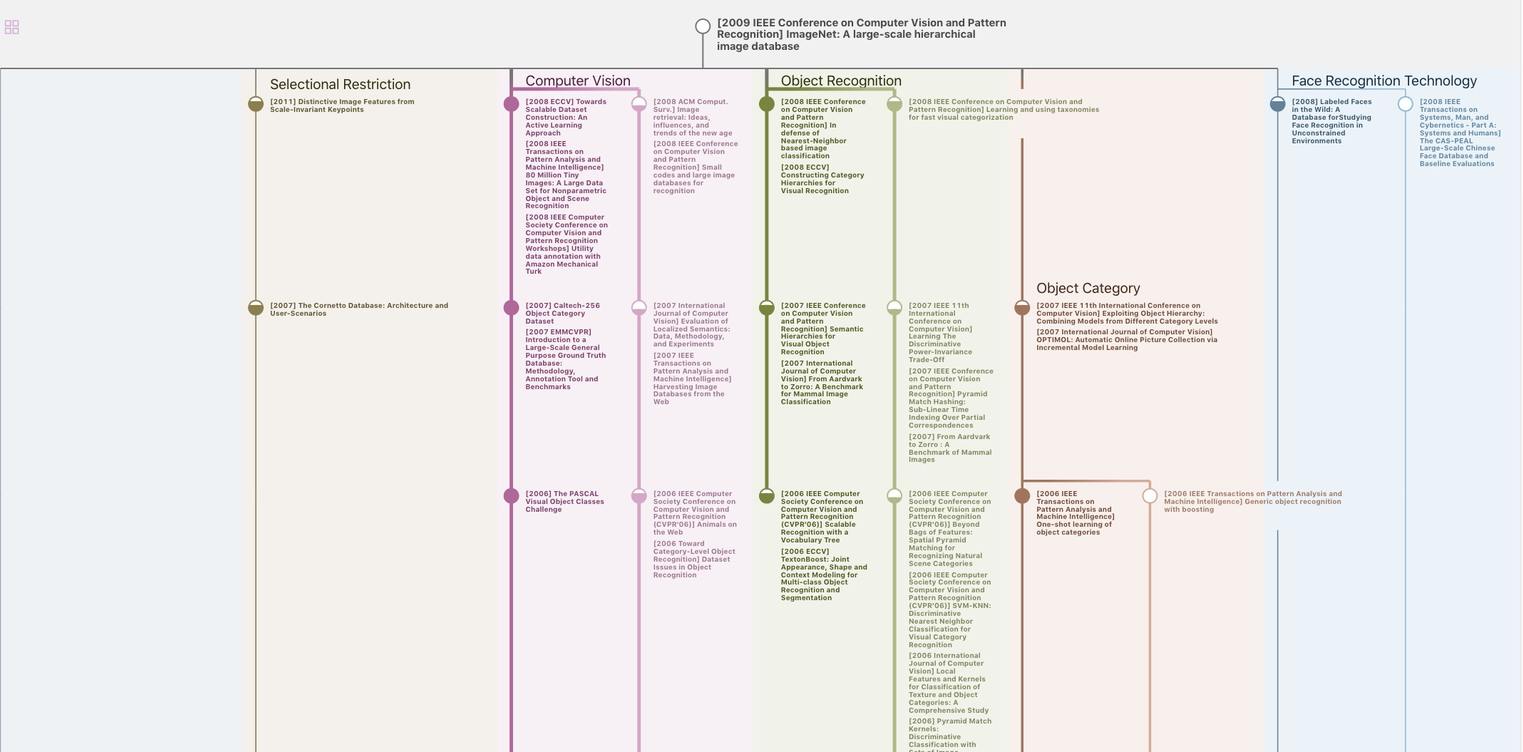
生成溯源树,研究论文发展脉络
Chat Paper
正在生成论文摘要