Beyond Text: A Deep Dive into Large Language Models' Ability on Understanding Graph Data
CoRR(2023)
摘要
Large language models (LLMs) have achieved impressive performance on many natural language processing tasks. However, their capabilities on graph-structured data remain relatively unexplored. In this paper, we conduct a series of experiments benchmarking leading LLMs on diverse graph prediction tasks spanning node, edge, and graph levels. We aim to assess whether LLMs can effectively process graph data and leverage topological structures to enhance performance, compared to specialized graph neural networks. Through varied prompt formatting and task/dataset selection, we analyze how well LLMs can interpret and utilize graph structures. By comparing LLMs' performance with specialized graph models, we offer insights into the strengths and limitations of employing LLMs for graph analytics. Our findings provide insights into LLMs' capabilities and suggest avenues for further exploration in applying them to graph analytics.
更多查看译文
关键词
large language models,graph data
AI 理解论文
溯源树
样例
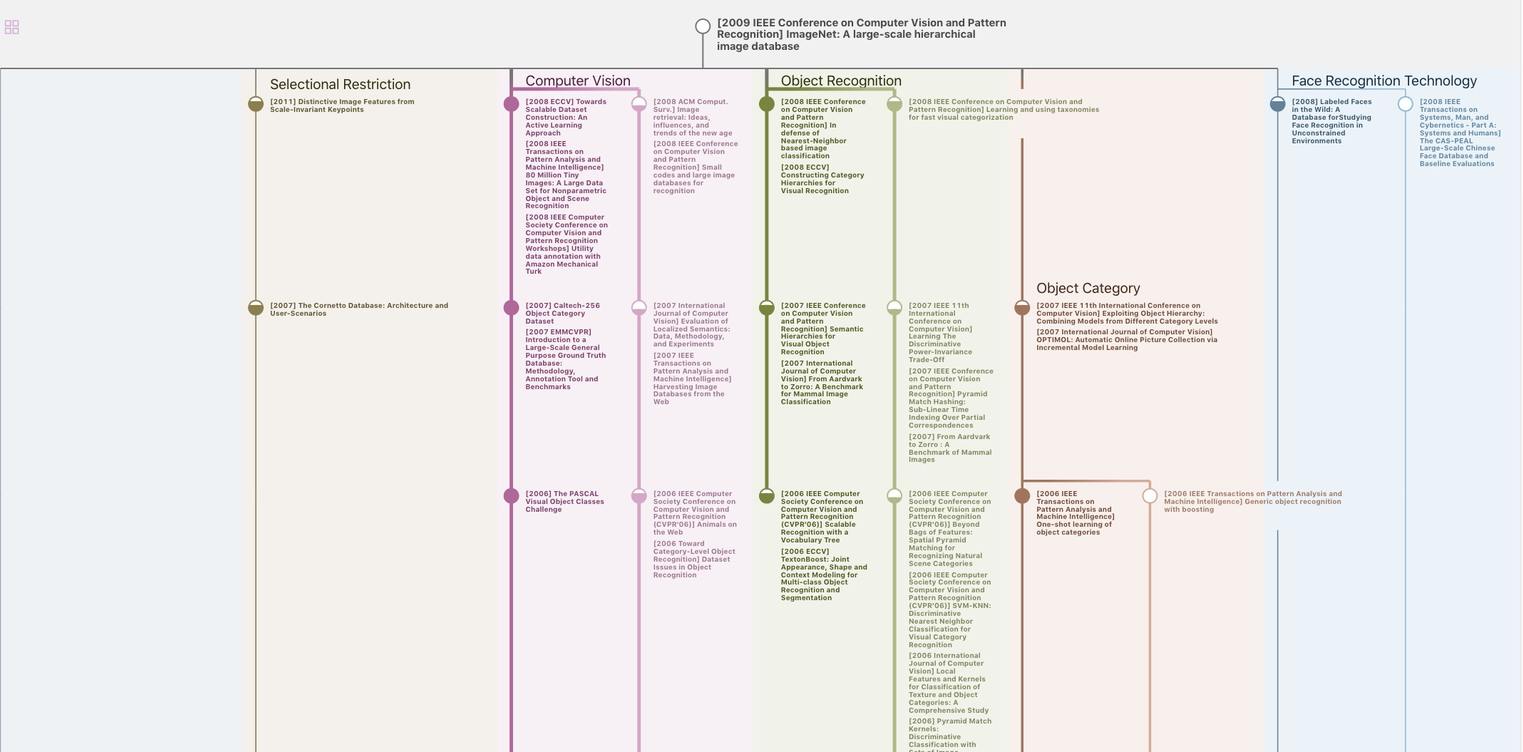
生成溯源树,研究论文发展脉络
Chat Paper
正在生成论文摘要