SEE-MCAM: Scalable Multi-bit FeFET Content Addressable Memories for Energy Efficient Associative Search
2023 IEEE/ACM INTERNATIONAL CONFERENCE ON COMPUTER AIDED DESIGN, ICCAD(2023)
摘要
Artificial intelligence has made remarkable advancements in recent years, leading to the development of algorithms and models capable of handling ever-increasing amounts of data. The computational demands of these algorithms necessitate circuit and architecture designs that go beyond the von-Neumann paradigm. Content addressable memories (CAMs), which implement parallel associative search functionality within memory blocks to overcome the memory wall bottleneck, have proven to be effective for data-intensive tasks. While current CAM designs have achieved higher storage density and energy efficiency than their CMOS-based counterparts by leveraging emerging non-volatile memories (NVM), most of these implementations are limited to binary storage cells. In this work, we propose SEE-MCAM, scalable and compact multi-bit CAM (MCAM) designs that utilize the three-terminal ferroelectric FET (FeFET) as the proxy. By exploiting the multi-level-cell characteristics of FeFETs, our proposed SEE-MCAM designs enable multi-bit associative search functions and achieve better energy efficiency and performance than existing FeFET-based CAM designs. We validated the functionality of our proposed designs by achieving 3 bits per cell CAM functionality, resulting in 3x improvement in storage density. The area per bit of the proposed SEE-MCAM cell is 8% of the conventional CMOS CAM. We thoroughly investigated the scalability and robustness of the proposed design. Evaluation results suggest that the proposed 2FeFET-1T SEE-MCAM achieves 9.8x more energy efficiency and 1.6x less search latency compared to the CMOS CAM, respectively. When compared to existing MCAM designs, the proposed SEE-MCAM can achieve 8.7x and 4.9x more energy efficiency than ReRAM-based and FeFET-based MCAMs, respectively. Benchmarking results show that our approach provides up to 3 orders of magnitude improvement in speedup and energy efficiency over a GPU implementation in accelerating a novel quantized hyperdimensional computing (HDC) application.
更多查看译文
AI 理解论文
溯源树
样例
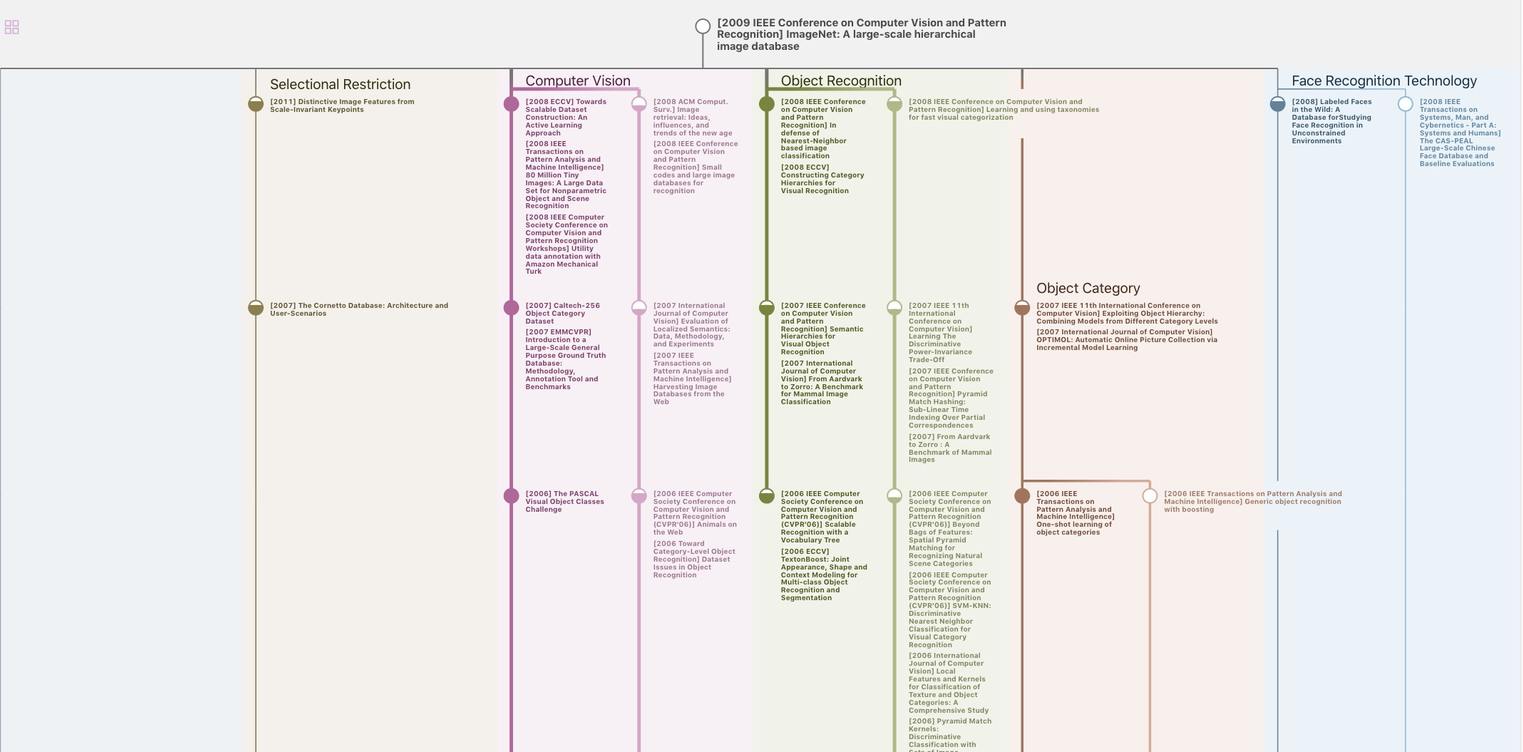
生成溯源树,研究论文发展脉络
Chat Paper
正在生成论文摘要