Crystal-GFN: sampling crystals with desirable properties and constraints
CoRR(2023)
摘要
Accelerating material discovery holds the potential to greatly help mitigate the climate crisis. Discovering new solid-state crystals such as electrocatalysts, ionic conductors or photovoltaics can have a crucial impact, for instance, in improving the efficiency of renewable energy production and storage. In this paper, we introduce Crystal-GFlowNet, a generative model of crystal structures that sequentially samples a crystal's composition, space group and lattice parameters. This domain-inspired approach enables the flexible incorporation of physical and geometrical constraints, as well as the use of any available predictive model of a desired property as an objective function. We evaluate the capabilities of Crystal-GFlowNet by using as objective the formation energy of a crystal structure, as predicted by a new proxy model trained on MatBench. The results demonstrate that Crystal-GFlowNet is able to sample diverse crystals with low formation energy.
更多查看译文
关键词
crystals,crystal-gfn
AI 理解论文
溯源树
样例
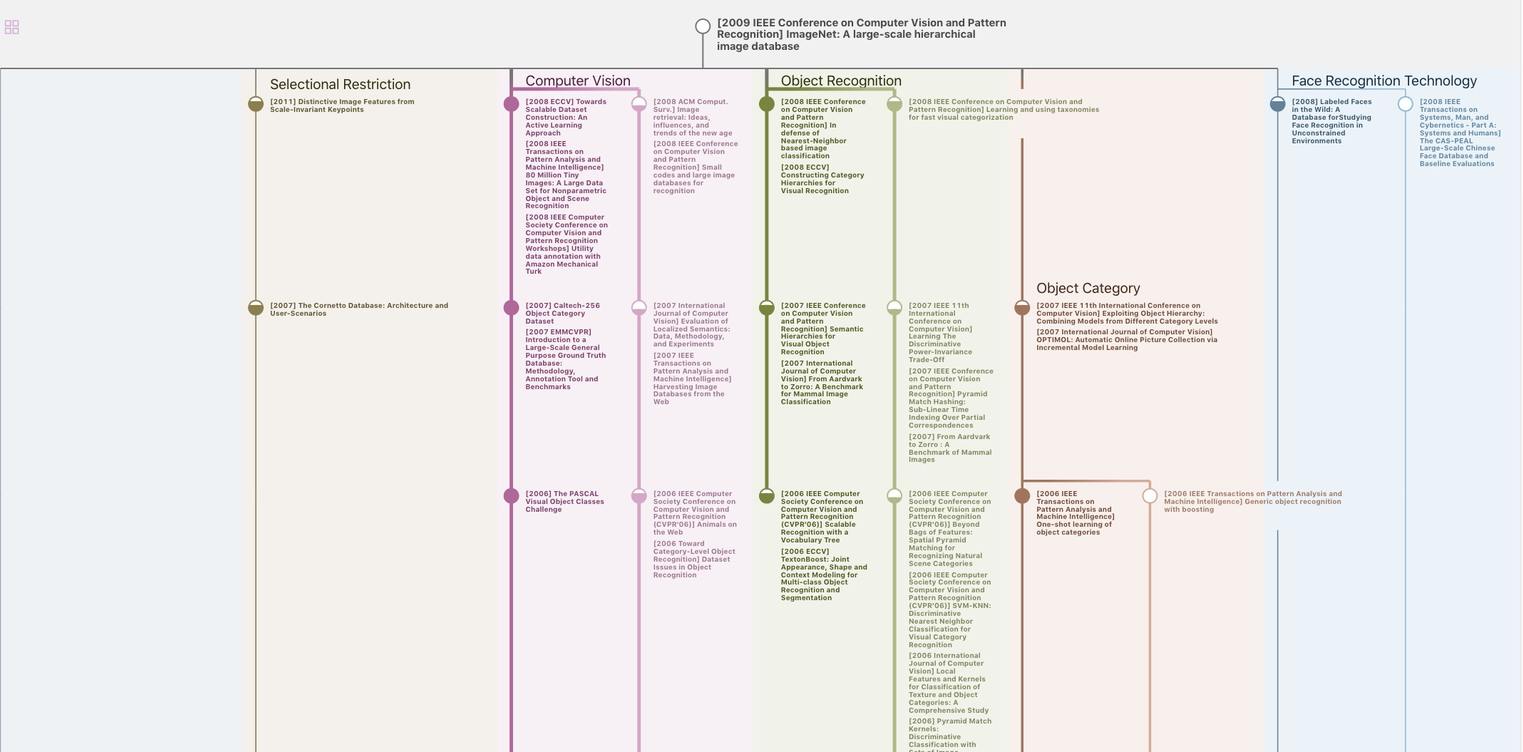
生成溯源树,研究论文发展脉络
Chat Paper
正在生成论文摘要