Faithful Knowledge Graph Explanations for Commonsense Reasoning
CoRR(2023)
摘要
While fusing language models and knowledge graphs has become common in
commonsense question answering research, enabling faithful chain-of-thought
explanations in these models remains an open problem. Our analysis reveals that
one major weakness of current KG-based explanation methodologies lies in
overlooking the faithfulness of path decoding during evaluation. This oversight
leads to the distribution of the graph encoder often diverging from the
original model predictions. To address this gap, we present two main
contributions: (1) We propose and validate Text-GNN Fidelity in this specific
context, to assess the reliability of the graph representation. (2) We
introduce TeGDA (Text-Graph Distribution-aware Alignment), a novel algorithm
that aligns the graph encoder with the target model to improve the faithfulness
of subsequent explanations and that can be easily integrated into existing
approaches. Our experiments and analysis show its potential to produce more
faithful systems. Concretely, our work emphasises the neglected distributional
misalignment problem in LM-KG reasoning models, which has been a latent source
of spurious explanations.
更多查看译文
关键词
faithful knowledge graph explanations
AI 理解论文
溯源树
样例
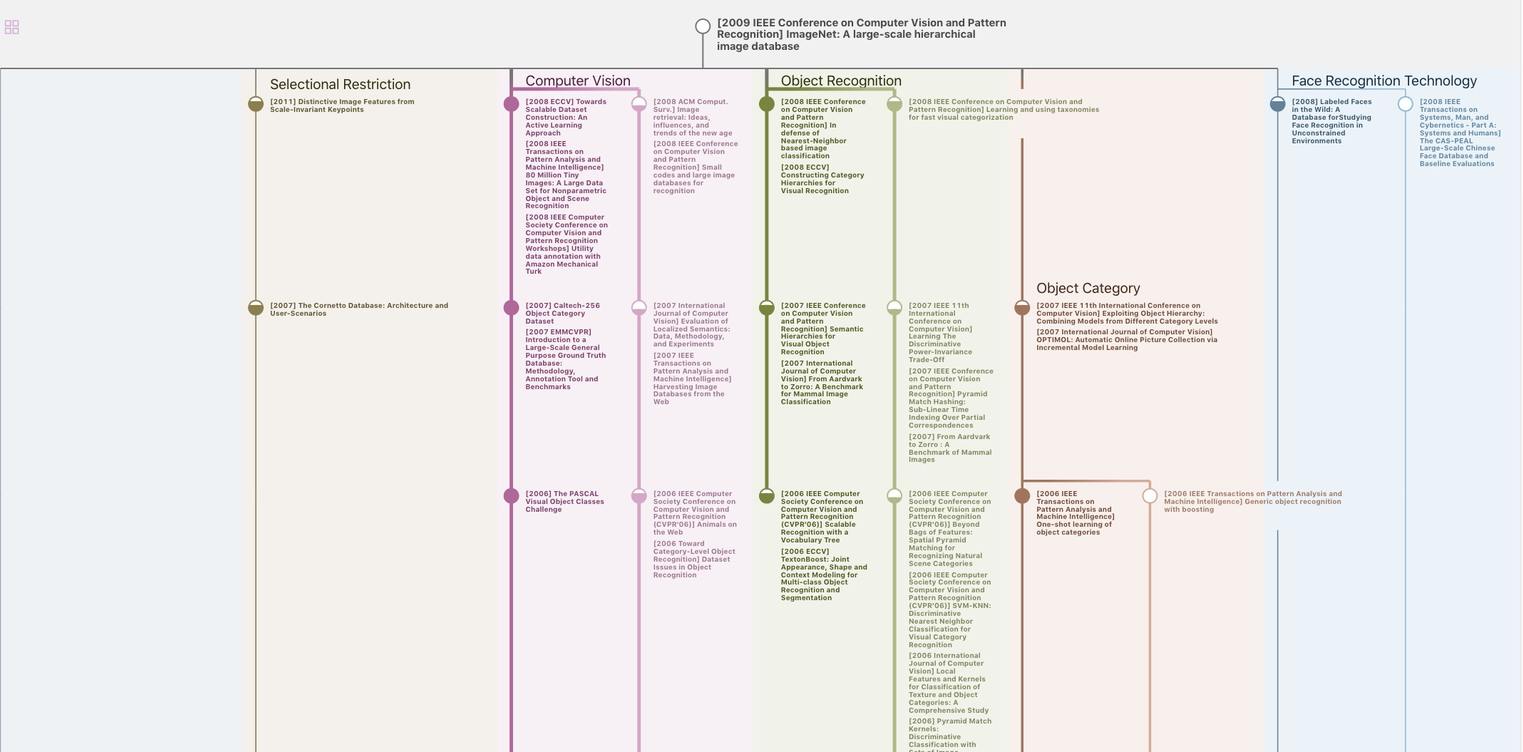
生成溯源树,研究论文发展脉络
Chat Paper
正在生成论文摘要