Visual Abductive Reasoning Meets Driving Hazard Prediction
CoRR(2023)
摘要
This paper addresses the problem of predicting hazards that drivers may
encounter while driving a car. We formulate it as a task of anticipating
impending accidents using a single input image captured by car dashcams. Unlike
existing approaches to driving hazard prediction that rely on computational
simulations or anomaly detection from videos, this study focuses on high-level
inference from static images. The problem needs predicting and reasoning about
future events based on uncertain observations, which falls under visual
abductive reasoning. To enable research in this understudied area, a new
dataset named the DHPR (Driving Hazard Prediction and Reasoning) dataset is
created. The dataset consists of 15K dashcam images of street scenes, and each
image is associated with a tuple containing car speed, a hypothesized hazard
description, and visual entities present in the scene. These are annotated by
human annotators, who identify risky scenes and provide descriptions of
potential accidents that could occur a few seconds later. We present several
baseline methods and evaluate their performance on our dataset, identifying
remaining issues and discussing future directions. This study contributes to
the field by introducing a novel problem formulation and dataset, enabling
researchers to explore the potential of multi-modal AI for driving hazard
prediction.
更多查看译文
关键词
visual abductive reasoning,driving hazard prediction
AI 理解论文
溯源树
样例
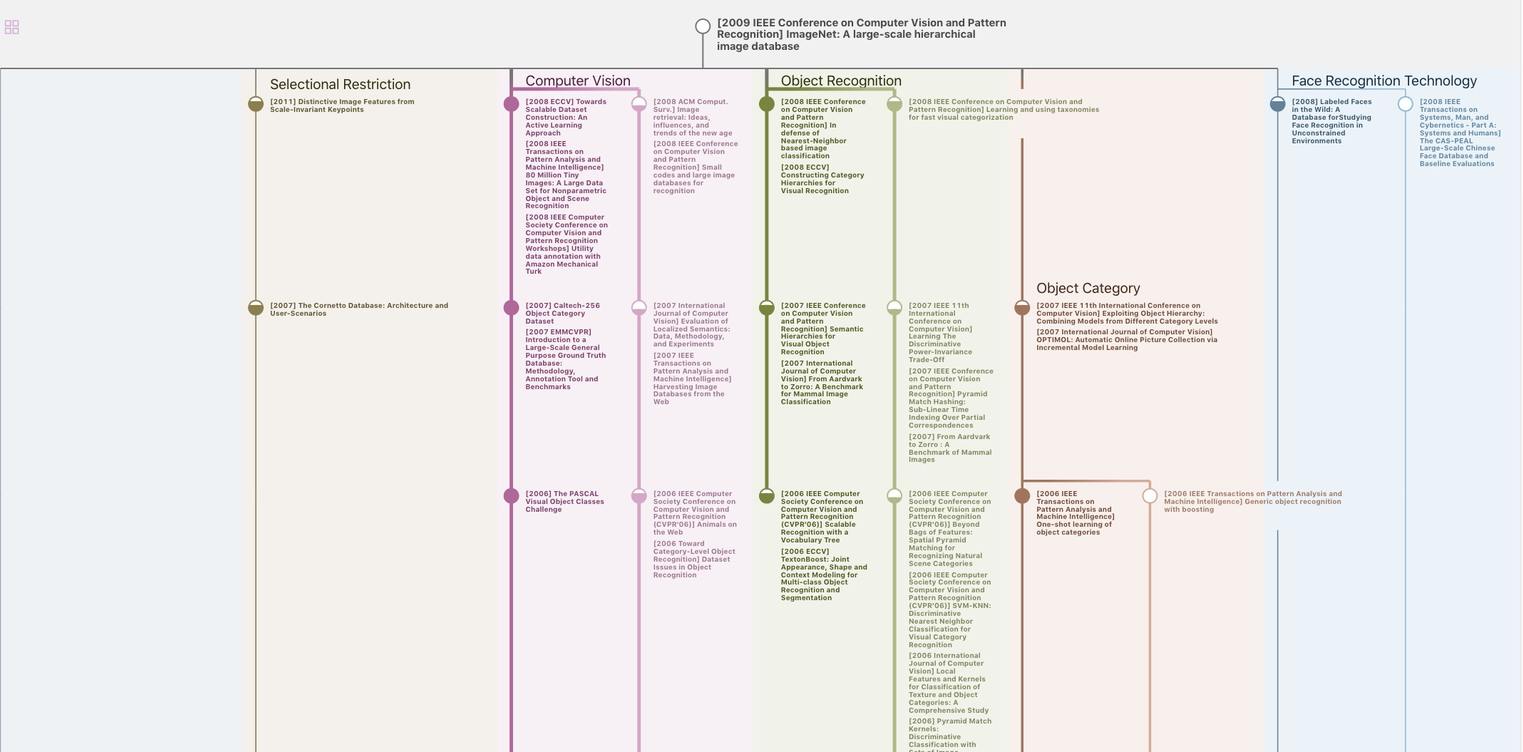
生成溯源树,研究论文发展脉络
Chat Paper
正在生成论文摘要