HalluciDet: Hallucinating RGB Modality for Person Detection Through Privileged Information
2024 IEEE/CVF Winter Conference on Applications of Computer Vision (WACV)(2023)
摘要
A powerful way to adapt a visual recognition model to a new domain is through
image translation. However, common image translation approaches only focus on
generating data from the same distribution as the target domain. Given a
cross-modal application, such as pedestrian detection from aerial images, with
a considerable shift in data distribution between infrared (IR) to visible
(RGB) images, a translation focused on generation might lead to poor
performance as the loss focuses on irrelevant details for the task. In this
paper, we propose HalluciDet, an IR-RGB image translation model for object
detection. Instead of focusing on reconstructing the original image on the IR
modality, it seeks to reduce the detection loss of an RGB detector, and
therefore avoids the need to access RGB data. This model produces a new image
representation that enhances objects of interest in the scene and greatly
improves detection performance. We empirically compare our approach against
state-of-the-art methods for image translation and for fine-tuning on IR, and
show that our HalluciDet improves detection accuracy in most cases by
exploiting the privileged information encoded in a pre-trained RGB detector.
Code: https://github.com/heitorrapela/HalluciDet
更多查看译文
关键词
hallucinating rgb modality,person detection through
AI 理解论文
溯源树
样例
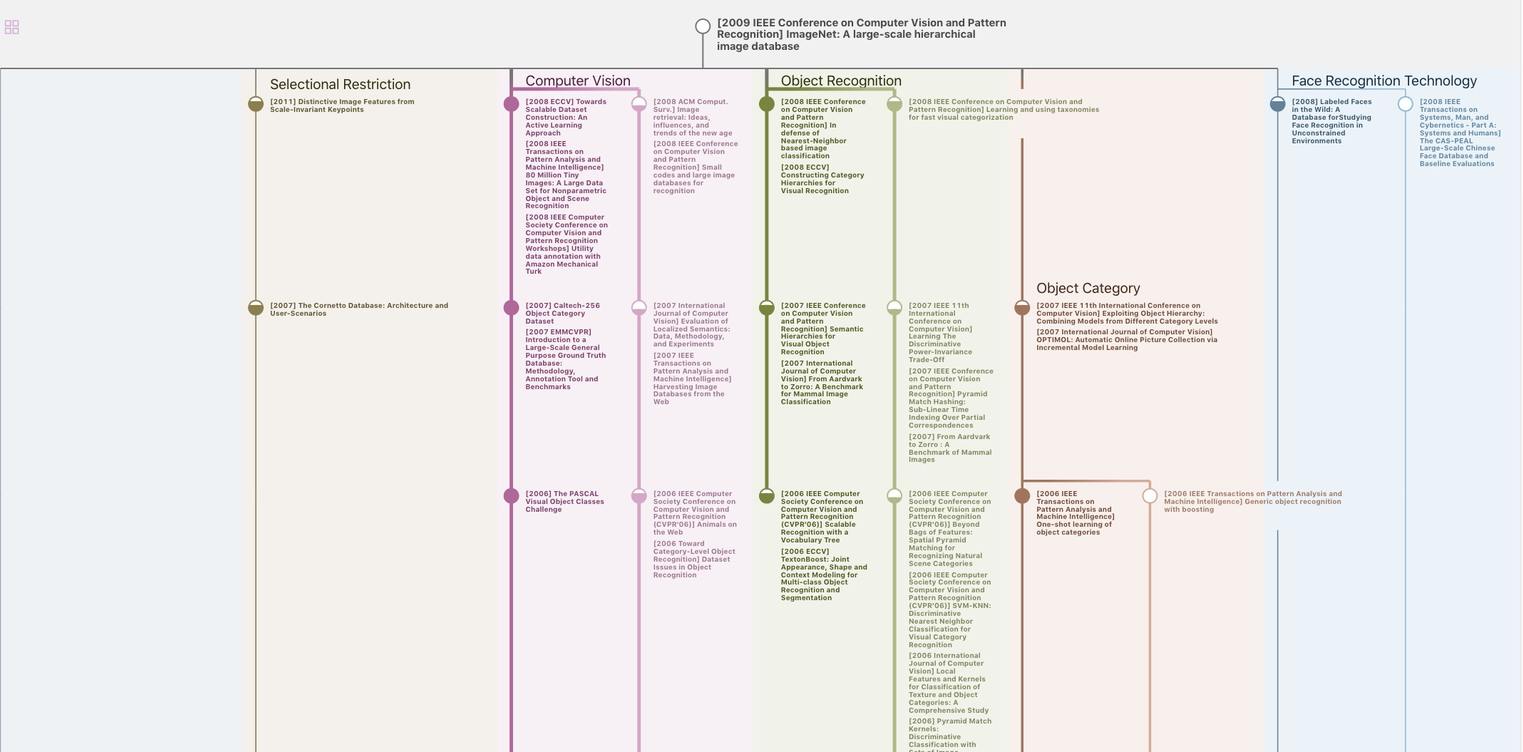
生成溯源树,研究论文发展脉络
Chat Paper
正在生成论文摘要