Lie Neurons: Adjoint-Equivariant Neural Networks for Semisimple Lie Algebras
CoRR(2023)
摘要
This paper proposes an equivariant neural network that takes data in any
semi-simple Lie algebra as input. The corresponding group acts on the Lie
algebra as adjoint operations, making our proposed network adjoint-equivariant.
Our framework generalizes the Vector Neurons, a simple
SO(3)-equivariant network, from 3-D Euclidean space to Lie algebra
spaces, building upon the invariance property of the Killing form. Furthermore,
we propose novel Lie bracket layers and geometric channel mixing layers that
extend the modeling capacity. Experiments are conducted for the
𝔰𝔬(3) and 𝔰𝔩(3) Lie algebras on various tasks,
including fitting equivariant and invariant functions, learning system
dynamics, point cloud registration, and homography-based shape classification.
Our proposed equivariant network shows wide applicability and competitive
performance in various domains.
更多查看译文
关键词
semisimple lie,algebras,networks,adjoint-equivariant
AI 理解论文
溯源树
样例
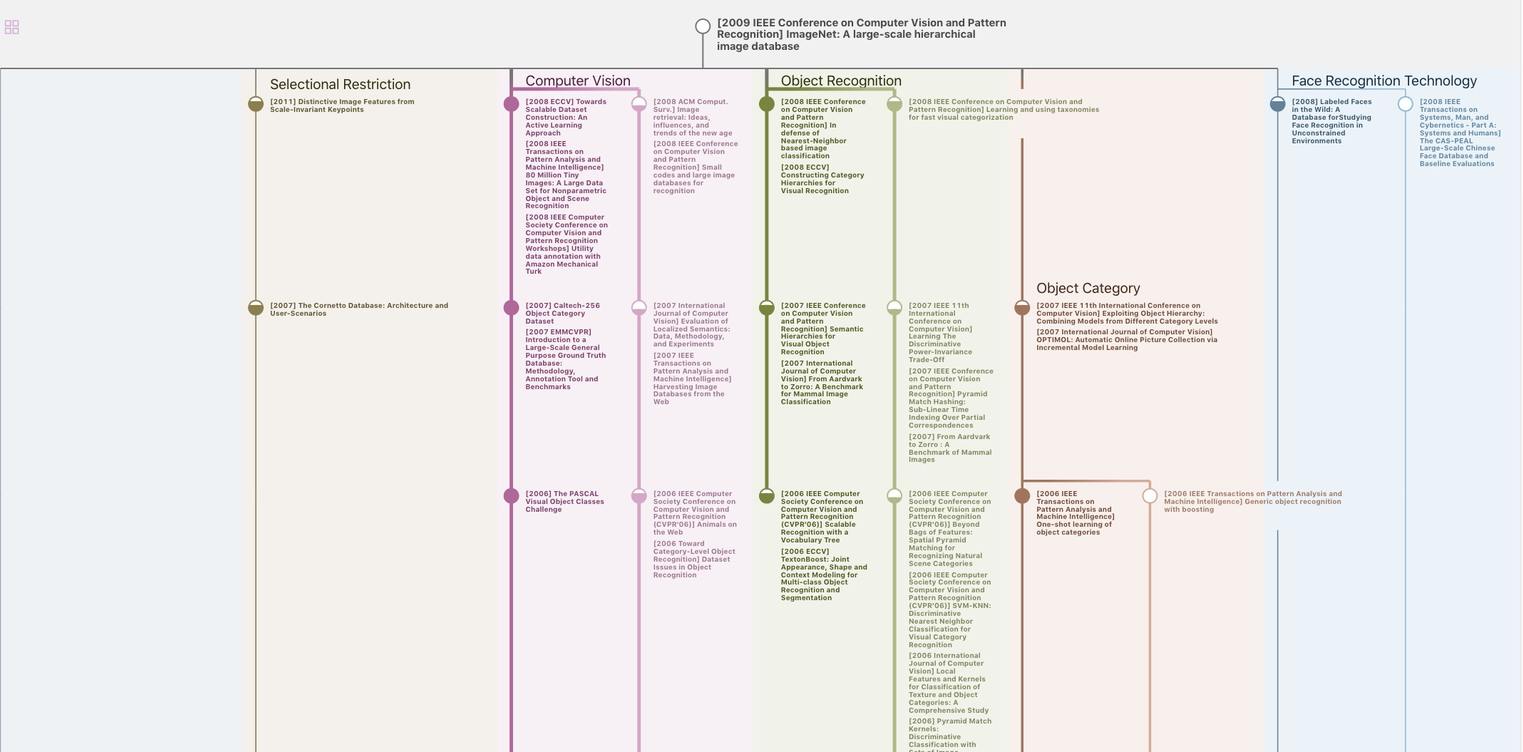
生成溯源树,研究论文发展脉络
Chat Paper
正在生成论文摘要