URLOST: Unsupervised Representation Learning without Stationarity or Topology
CoRR(2023)
摘要
Unsupervised representation learning has seen tremendous progress but is constrained by its reliance on data modality-specific stationarity and topology, a limitation not found in biological intelligence systems. For instance, human vision processes visual signals derived from irregular and non-stationary sampling lattices yet accurately perceives the geometry of the world. We introduce a novel framework that learns from high-dimensional data lacking stationarity and topology. Our model combines a learnable self-organizing layer, density adjusted spectral clustering, and masked autoencoders. We evaluate its effectiveness on simulated biological vision data, neural recordings from the primary visual cortex, and gene expression datasets. Compared to state-of-the-art unsupervised learning methods like SimCLR and MAE, our model excels at learning meaningful representations across diverse modalities without depending on stationarity or topology. It also outperforms other methods not dependent on these factors, setting a new benchmark in the field. This work represents a step toward unsupervised learning methods that can generalize across diverse high-dimensional data modalities.
更多查看译文
关键词
unsupervised representation learning
AI 理解论文
溯源树
样例
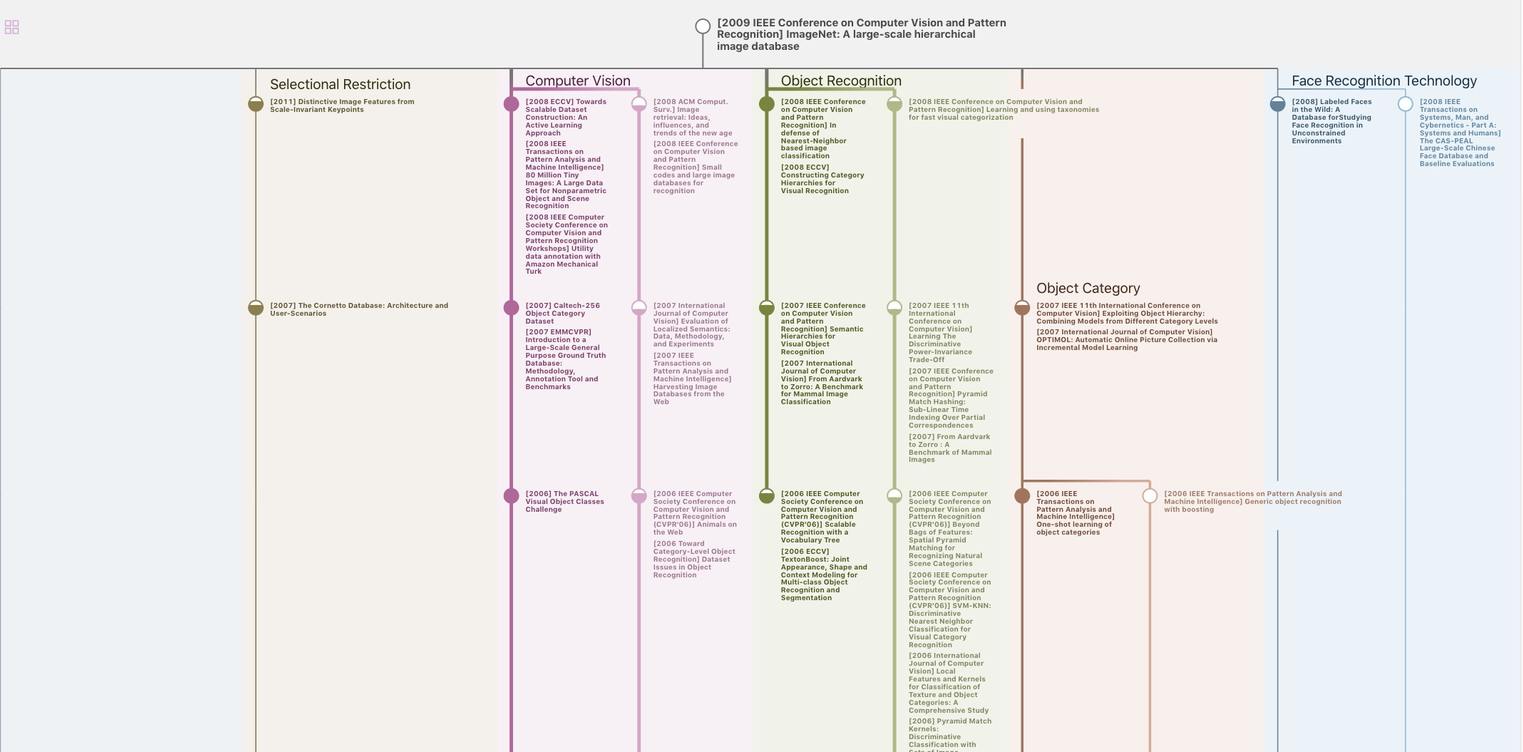
生成溯源树,研究论文发展脉络
Chat Paper
正在生成论文摘要