HNS: An efficient hermite neural solver for solving time-fractional partial differential equations
Chaos, Solitons & Fractals(2024)
摘要
Neural network solvers represent an innovative and promising approach for tackling time-fractional partial differential equations by utilizing deep learning techniques. L1 interpolation approximation serves as the standard method for addressing time-fractional derivatives within neural network solvers. However, we have discovered that neural network solvers based on L1 interpolation approximation are unable to fully exploit the benefits of neural networks, and the accuracy of these models is constrained to interpolation errors. In this paper, we present the high-precision Hermite Neural Solver (HNS) for solving time-fractional partial differential equations. Specifically, we first construct a high-order explicit approximation scheme for fractional derivatives using Hermite interpolation techniques, and rigorously analyze its approximation accuracy. Afterward, taking into account the infinitely differentiable properties of deep neural networks, we integrate the high-order Hermite interpolation explicit approximation scheme with deep neural networks to propose the HNS. The experimental results show that HNS achieves higher accuracy than methods based on the L1 scheme for both forward and inverse problems, as well as in high-dimensional scenarios. This indicates that HNS has significantly improved accuracy and flexibility compared to existing L1-based methods, and has overcome the limitations of explicit finite difference approximation methods that are often constrained to function value interpolation. As a result, the HNS is not a simple combination of numerical computing methods and neural networks, but rather achieves a complementary and mutually reinforcing advantages of both approaches. The data and code can be found at https://github.com/hsbhc/HNS.
更多查看译文
关键词
efficient hermite neural solver,differential equations,time-fractional
AI 理解论文
溯源树
样例
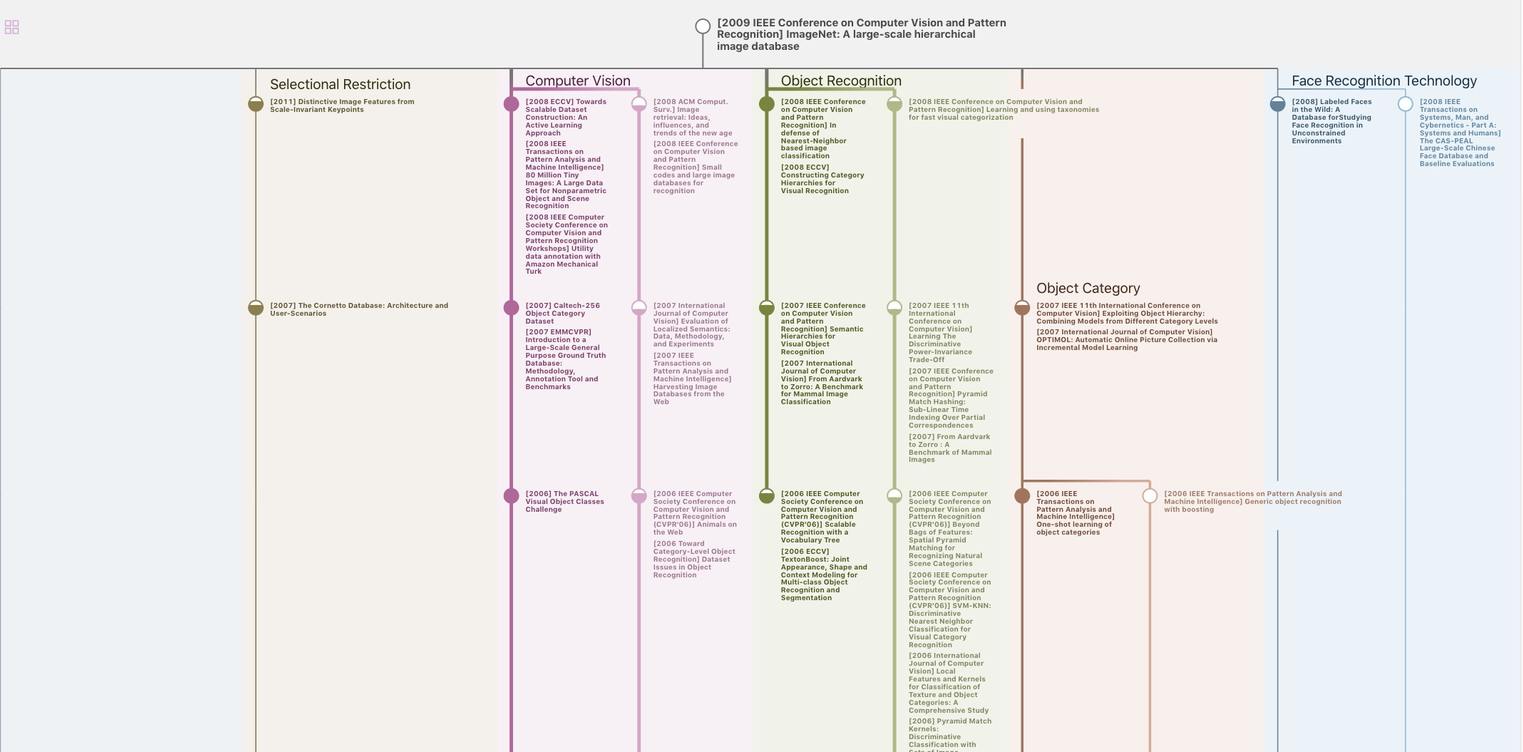
生成溯源树,研究论文发展脉络
Chat Paper
正在生成论文摘要