Domain Adaptive Recognition of Defects for Ground Penetrating Radar Images
2023 8th International Conference on Signal and Image Processing (ICSIP)(2023)
摘要
GPR technology is of significant importance for road maintenance authorities to detect road defect under the road surface, for it helps to monitor the construction process and detect defects early, thus reducing maintenance costs. The high cost of data collection and core verification for underground defects makes the dataset very valuable. In practical applications, different radar types and imaging methods often lead to heterogeneous generated radar images. In addition, there are significant differences between training data and real-world test data, which may lead to ambiguous predictions. This is because as the discrepance in different data domains increase, the transferability of the features extracted by neural networks significantly decreases at higher levels. Therefore, it is important to reduce dataset bias and enhance transferability in specific task layers. This article specifically designs a feature transfer enhancement algorithm for heterogeneous ground penetrating radar data based on deep domain adaptation. Compared with other deep domain adaptation algorithms such as DAN, DANN, and DeepCoral, the proposed method has the best recognition performance on underground defect data of ground penetrating radar.
更多查看译文
关键词
ground penetrating radar,domain adaptation,defect recognition
AI 理解论文
溯源树
样例
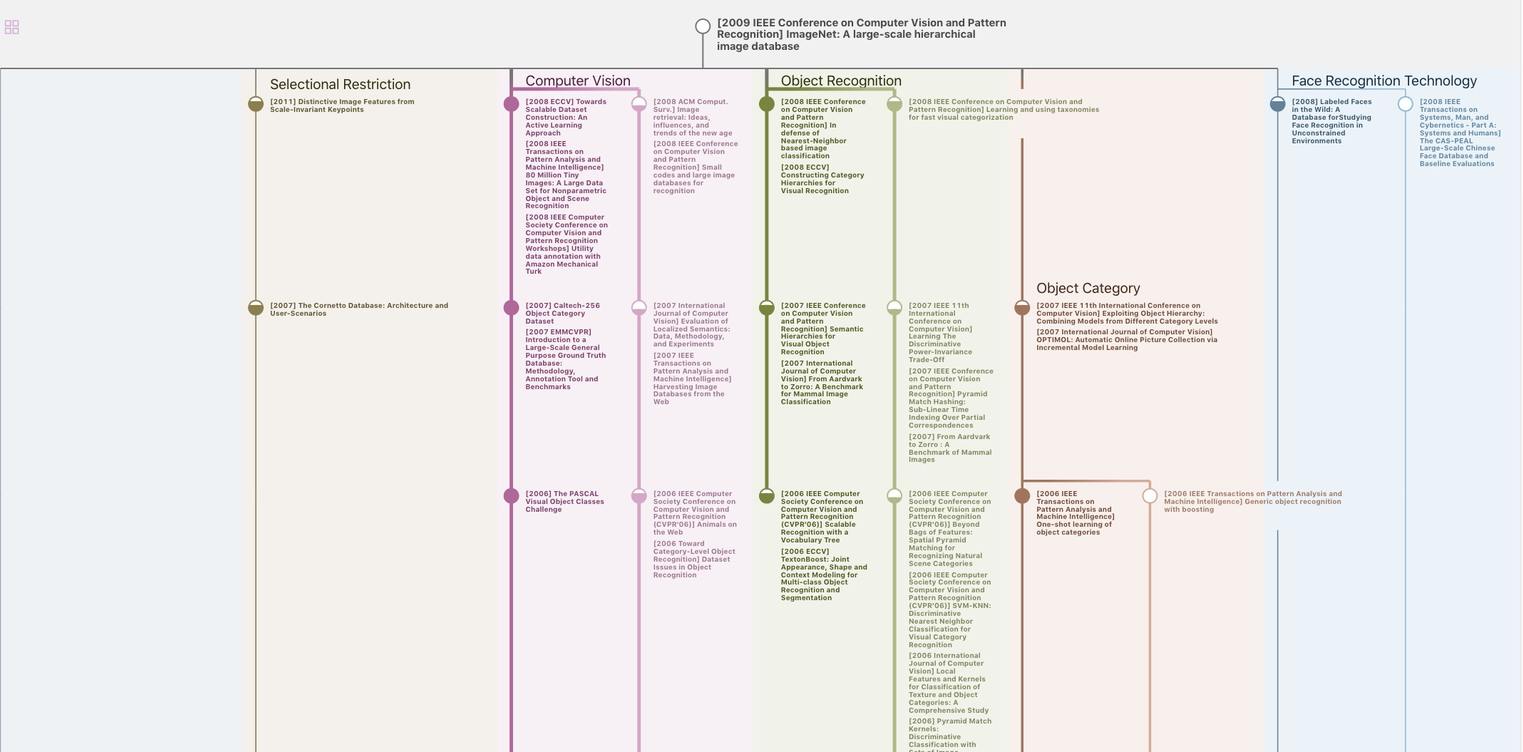
生成溯源树,研究论文发展脉络
Chat Paper
正在生成论文摘要