ML Models for Detecting QoE Degradation in Low-Latency Applications: A Cloud-Gaming Case Study
IEEE TRANSACTIONS ON NETWORK AND SERVICE MANAGEMENT(2023)
摘要
Detecting abnormal network events is an important activity of Internet Service Providers particularly when running critical applications (e.g., ultra low-latency applications in mobile wireless networks). Abnormal events can stress the infrastructure and lead to severe degradation of user experience. Machine Learning (ML) models have demonstrated their relevance in many tasks including Anomaly Detection (AD). While promising remarkable performance compared to manual or threshold-based detection, applying ML-based AD methods is challenging for operators due to the proliferation of ML models and the lack of well-established methodology and metrics to evaluate them and select the most appropriate one. This paper presents a comprehensive evaluation of eight unsupervised ML models selected from different classes of ML algorithms and applied to AD in the context of cloud gaming applications. We collect cloud gaming Key Performance Indicators (KPIs) time-series datasets in real-world network conditions, and we evaluate and compare the selected ML models using the same methodology, and assess their robustness to data contamination, their efficiency and computational complexity. In addition to the traditional F1-score performance metric used in anomaly detection, we use Matthews Coefficient Correlation (MCC) to better differentiate between models' efficiencies. Our proposed methodology relies on window-based anomaly detection techniques as they are more useful for network operators compared to single point detection approaches. However, we found most existing window-based approaches to lack in accuracy and may under or over-estimate a model's performance. Therefore, in this paper, we propose a novel Window Anomaly Decision (WAD) approach that overcomes these drawbacks. We leverage our experimental results to provide insights about the most relevant models for detecting QoE degradation and offer recommendations on their suitability for different application requirements.
更多查看译文
关键词
Anomaly detection,Quality of experience,Degradation,Data models,Computational modeling,Low latency communication,Prediction algorithms,Low-latency,anomaly detection,unsupervised learning,QoE,4G networks,metrics
AI 理解论文
溯源树
样例
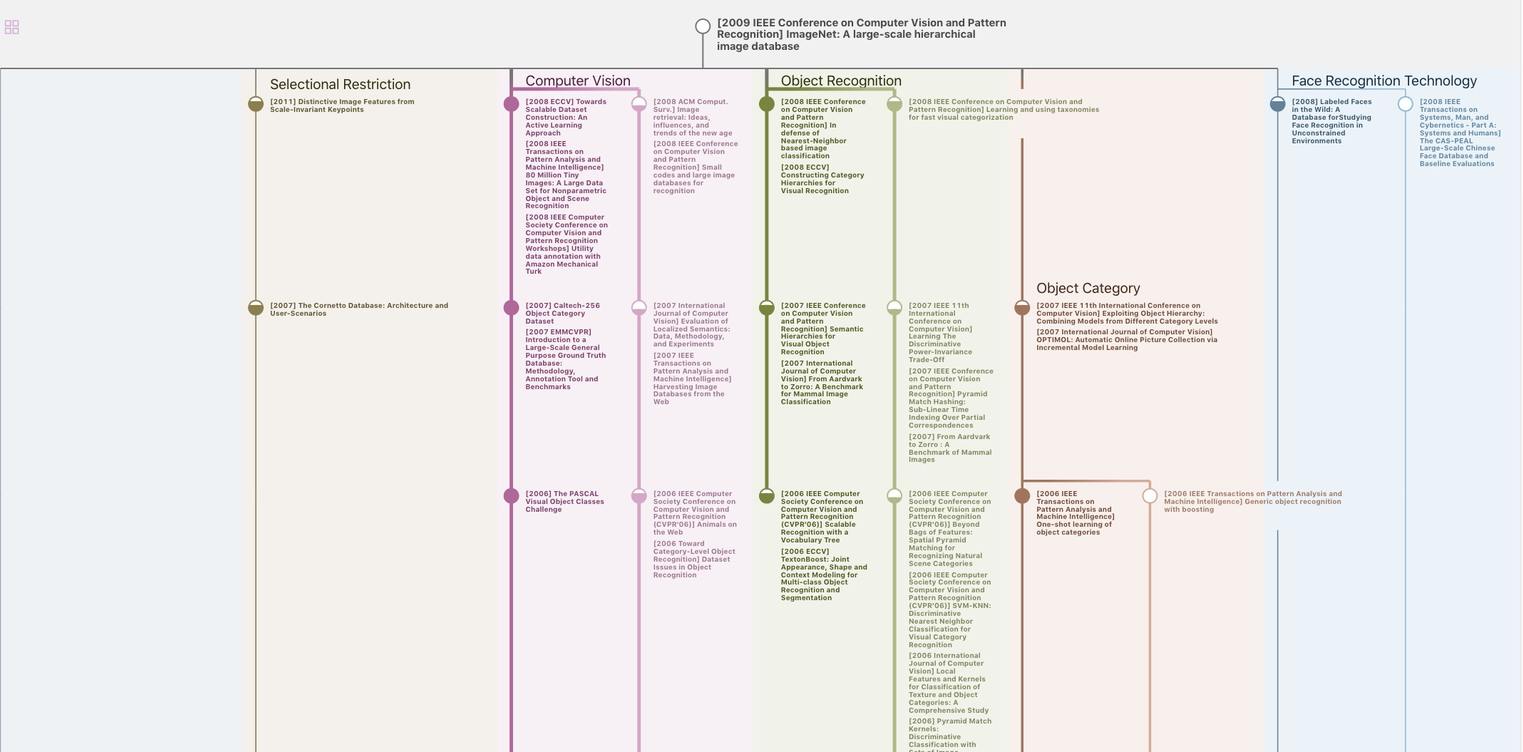
生成溯源树,研究论文发展脉络
Chat Paper
正在生成论文摘要