Neural network variational Monte Carlo for positronic chemistry
arXiv (Cornell University)(2023)
摘要
Gamma rays produced by positron annihilation are used as a sensitive probe of matter at atomic length scales. Technologies for manipulating positrons and studying their interactions with ordinary matter are rapidly progressing. This motivates the development of accurate ab initio methods for modelling positronic interactions with molecular matter. Here, we apply the recently developed Fermionic neural network (FermiNet) wavefunction ansatz to the problem of finding the ground-state properties of mixed positron-electron systems. We find that FermiNet produces highly accurate, in some cases state-of-the-art, ground-state energies across a range of atoms and small molecules with a wide range of qualitatively distinct positron binding characteristics. We highlight the capabilities of our method by calculating the positron binding energy of the challenging non-polar Benzene molecule.
更多查看译文
关键词
neural network,chemistry,monte
AI 理解论文
溯源树
样例
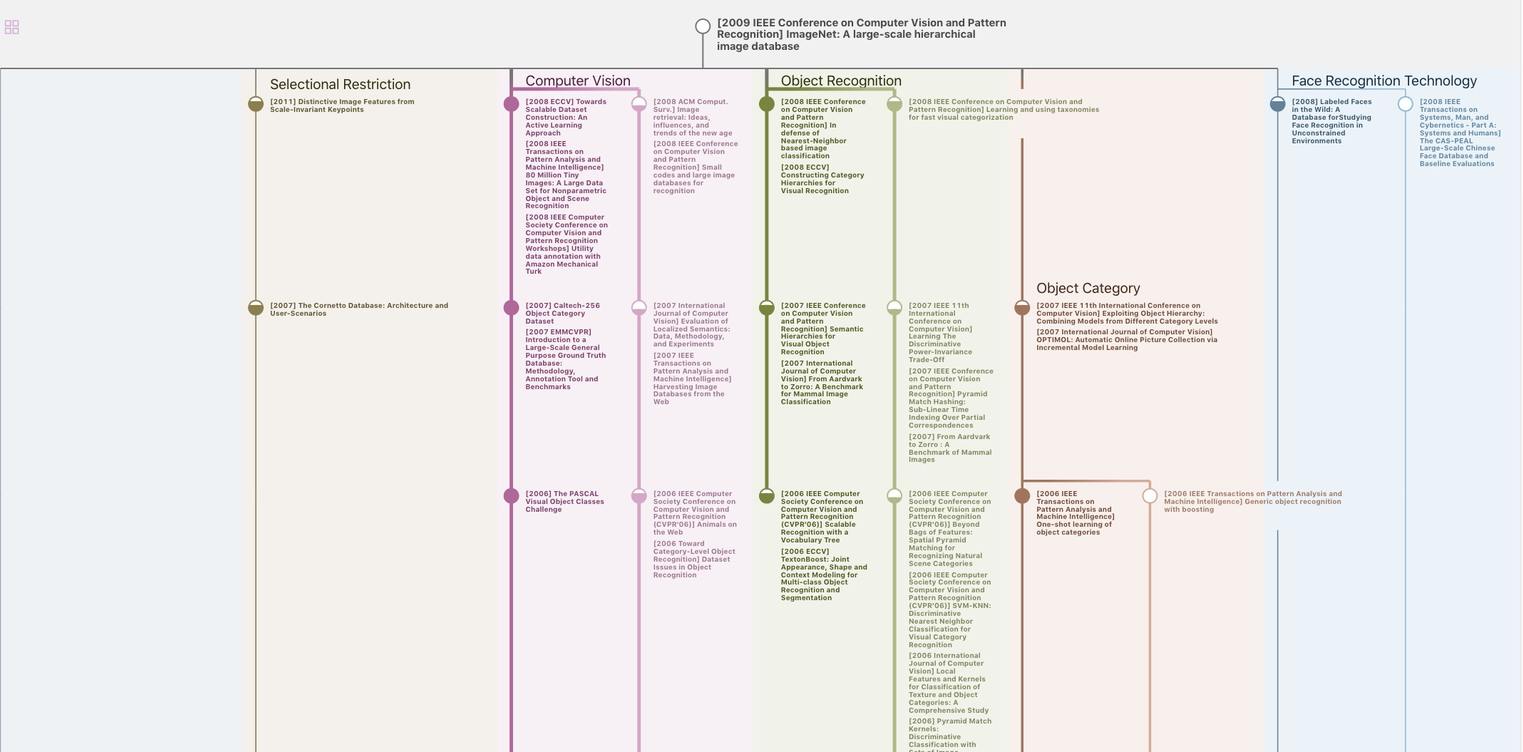
生成溯源树,研究论文发展脉络
Chat Paper
正在生成论文摘要