Toward Effective Personalized Service QoS Prediction From the Perspective of Multi-Task Learning
IEEE TRANSACTIONS ON NETWORK AND SERVICE MANAGEMENT(2023)
摘要
End-to-end QoS measurement plays an indispensable role in the decision-making of cloud services and IoT services. Many efforts have paid on developing QoS prediction approaches in the past decade leveraging the principle of collaborative filtering. But there remain many challenging issues concerning multi-task prediction requirements, feature selection for heterogeneous prediction tasks, and model training. To this end, we propose an effective personalized service QoS prediction method from the perspective of multi-task learning, named PMT. PMT consists of specially-designed feature selection components and a multi-step model training strategy. The feature selection method leverages the principle of multi-expert decision-making and self-attention mechanism. The multi-step model training enables a weight-free configuration for parallel prediction tasks. Experimental results on a large dataset with two tasks and a small dataset with three tasks demonstrate that PMT is superior to the state-of-the-art QoS prediction methods.
更多查看译文
关键词
Personalized QoS prediction,deep learning,multi-task learning,feature selection,attention mechanism
AI 理解论文
溯源树
样例
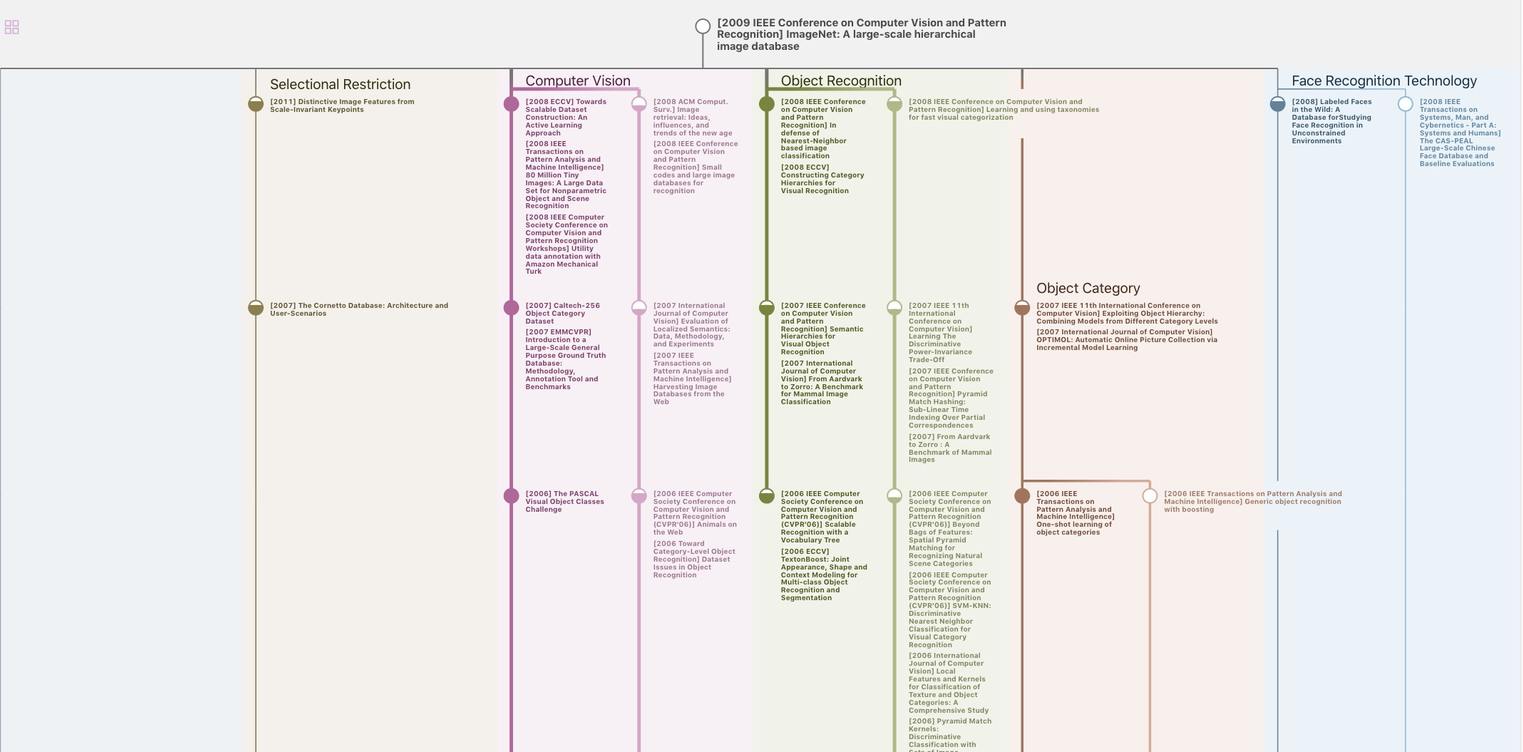
生成溯源树,研究论文发展脉络
Chat Paper
正在生成论文摘要