LogKG: Log Failure Diagnosis Through Knowledge Graph
IEEE Transactions on Services Computing(2023)
摘要
Logs are one of the most valuable data to describe the running state of services. Failure diagnosis through logs is crucial for service reliability and security. The current automatic log failure diagnosis methods cannot fully use the multiple fields of logs, which fail to capture the relation between them. In this article, we propose LogKG, a new framework for diagnosing failures based on knowledge graphs (KG) of logs. LogKG fully extracts entities and relations from logs to mine multi-field information and their relations through the KG. To fully use the information represented by KG, we propose a failure-oriented log representation (FOLR) method to extract the failure-related patterns. Utilizing the OPTICS clustering method, LogKG aggregates historical failure cases, labels typical failure cases, and trains a failure diagnosis model to identify the root cause. We evaluate the effectiveness of LogKG on a real-world log dataset and a public log dataset, respectively, showing that it outperforms existing methods. With the deployment in a top-tier global Internet Service Provider (ISP), we demonstrate the performance and practicability of LogKG.
更多查看译文
关键词
Semantics, Task analysis, Sun, Manuals, Security, Natural language processing, Knowledge graphs, Cluster, diagnosis, embedding, LogKG
AI 理解论文
溯源树
样例
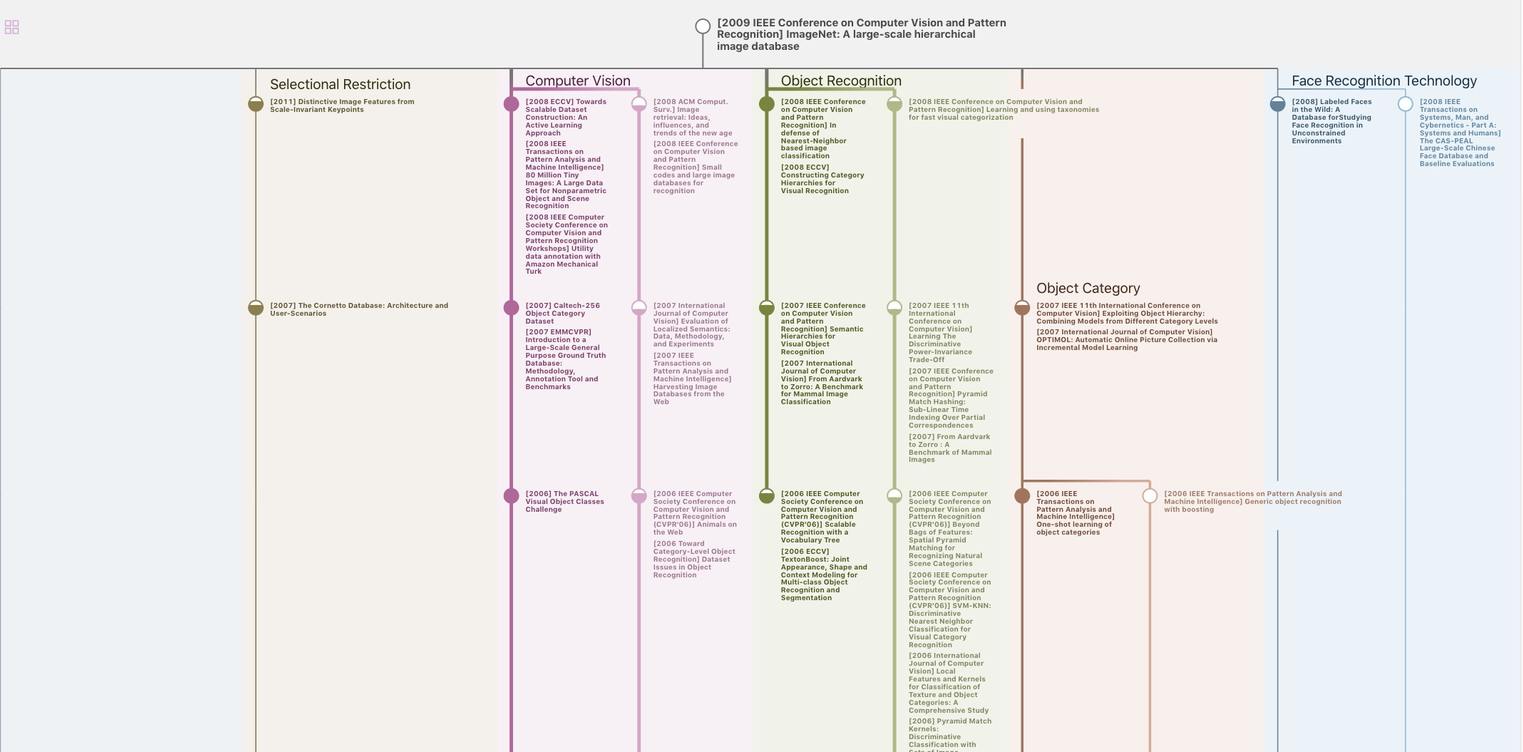
生成溯源树,研究论文发展脉络
Chat Paper
正在生成论文摘要