Robust cosmological inference from non-linear scales with k-th nearest neighbor statistics
Monthly Notices of the Royal Astronomical Society(2023)
摘要
We present the methodology for deriving accurate and reliable cosmological constraints from non-linear scales (<50Mpc/h) with k-th nearest neighbor (kNN) statistics. We detail our methods for choosing robust minimum scale cuts and validating galaxy-halo connection models. Using cross-validation, we identify the galaxy-halo model that ensures both good fits and unbiased predictions across diverse summary statistics. We demonstrate that we can model kNNs effectively down to transverse scales of rp ~ 3Mpc/h and achieve precise and unbiased constraints on the matter density and clustering amplitude, leading to a 2% constraint on sigma_8. Our simulation-based model pipeline is resilient to varied model systematics, spanning simulation codes, halo finding, and cosmology priors. We demonstrate the effectiveness of this approach through an application to the Beyond-2p mock challenge. We propose further explorations to test more complex galaxy-halo connection models and tackle potential observational systematics.
更多查看译文
关键词
robust cosmological inference,nearest
AI 理解论文
溯源树
样例
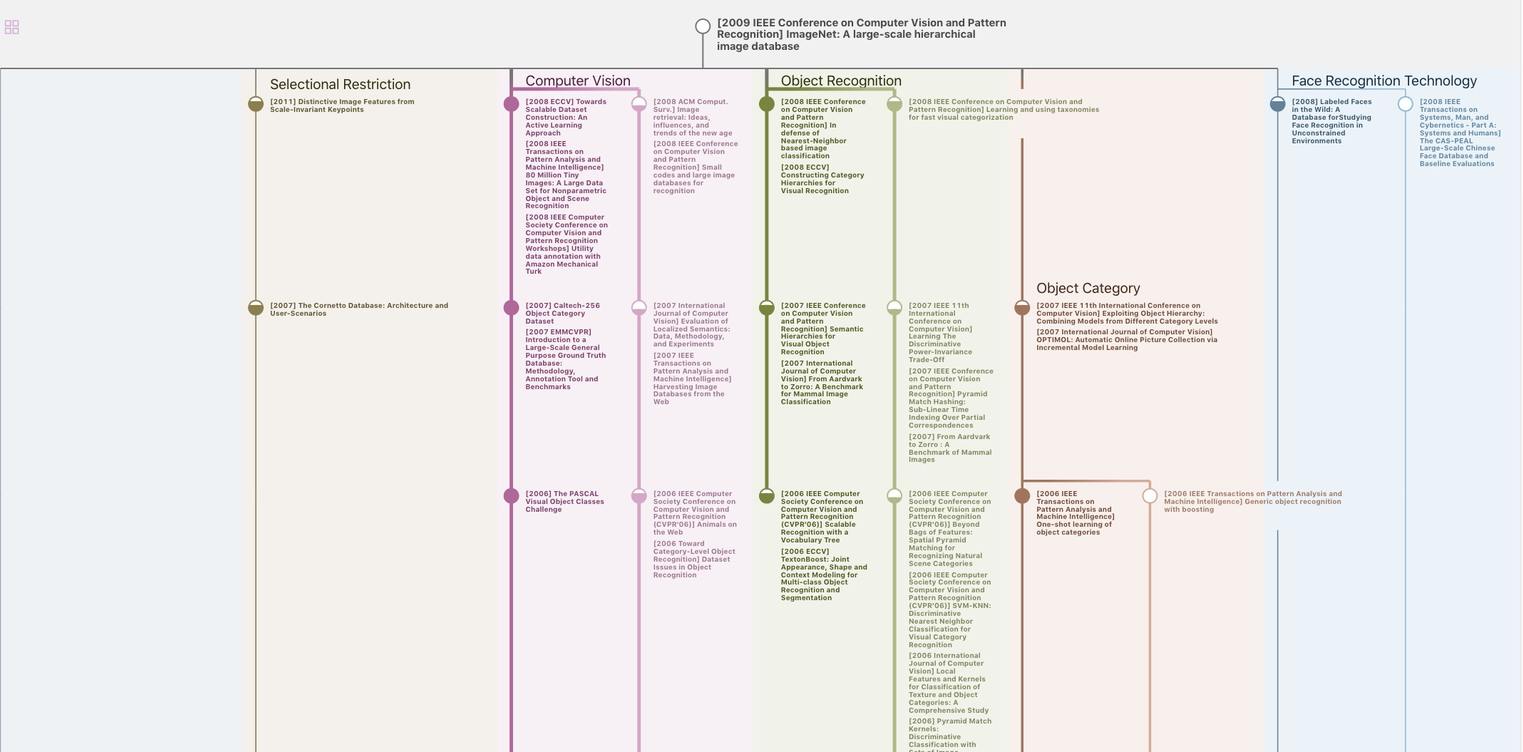
生成溯源树,研究论文发展脉络
Chat Paper
正在生成论文摘要