A Zipf's law-based text generation approach for addressing imbalance in entity extraction
JOURNAL OF INFORMETRICS(2023)
摘要
Entity extraction is critical in the intelligent advancement across diverse domains. Nevertheless, a challenge to its effectiveness arises from the data imbalance, where certain entities are common while others are scarce. To address this issue, this study proposes a novel text generation approach that harnesses Zipf's law, which is a powerful tool from informetrics for studying human language. By employing characteristics of Zipf's law, words within the documents are classified as common and rare ones. Subsequently, sentences are classified into common and rare ones, and are further processed by text generation models accordingly. Rare entities within the generated sentences are then labeled using human-designed rules, serving as a supplement to the raw dataset, thereby mitigating the imbalance problem. The study presents a case of extracting entities from technical documents, and the extensive experimental results on two datasets prove the effectiveness of the proposed method. Furthermore, the significance and potential of Zipf's law in driving the progress of artificial intelligence (AI) is discussed, broadening the scope and coverage of informetrics. By incorporating the foundational principles of informetrics into text generation, this study showcases the pivotal role of informetrics in shaping the design and developmental of AI systems.
更多查看译文
关键词
Zipf's law,Data imbalance,Text generation,Entity extraction
AI 理解论文
溯源树
样例
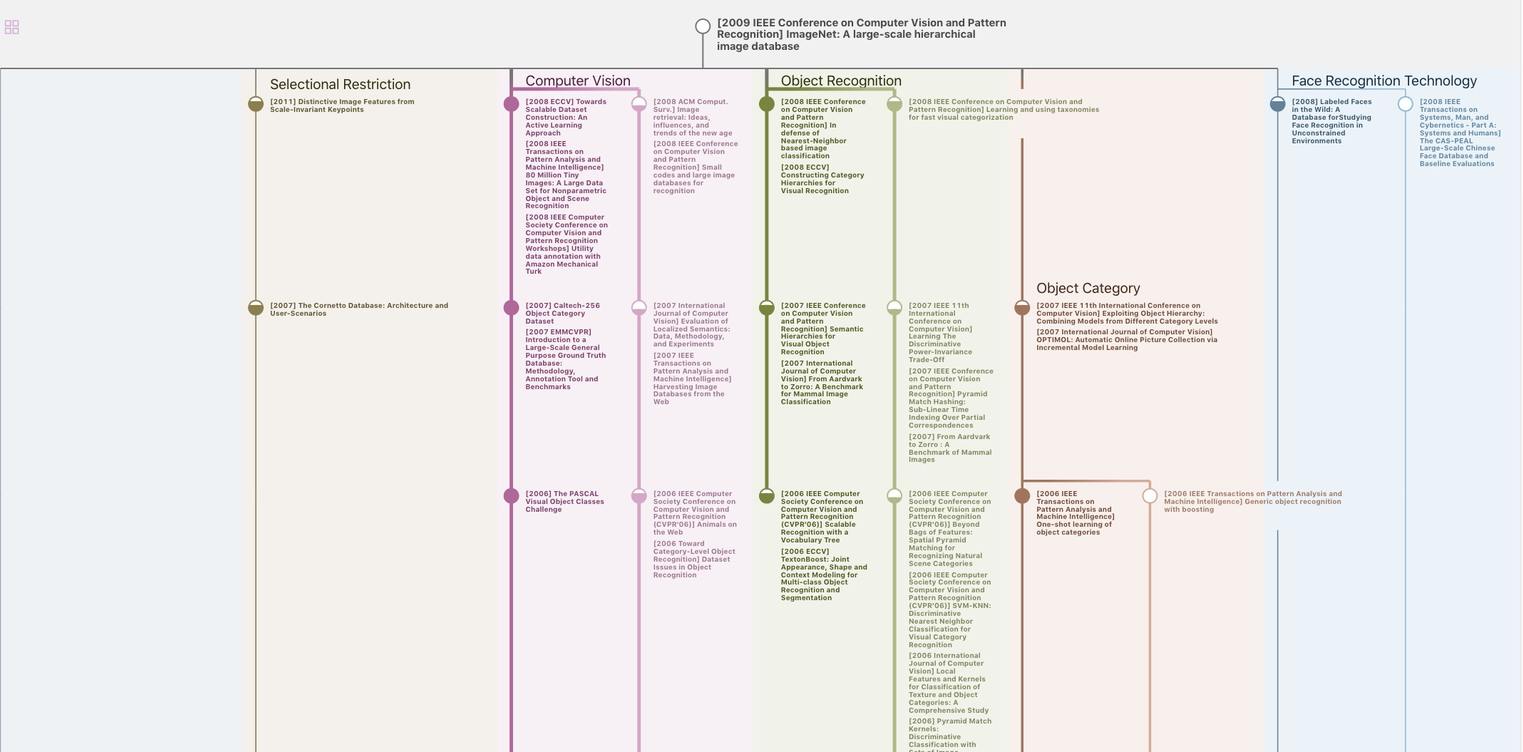
生成溯源树,研究论文发展脉络
Chat Paper
正在生成论文摘要