The Effects of Aberrant Responding on Model-Fit Assuming Different Underlying Response Processes
APPLIED PSYCHOLOGICAL MEASUREMENT(2023)
摘要
Aberrant responding on tests and surveys has been shown to affect the psychometric properties of scales and the statistical analyses from the use of those scales in cumulative model contexts. This study extends prior research by comparing the effects of four types of aberrant responding on model fit in both cumulative and ideal point model contexts using graded partial credit (GPCM) and generalized graded unfolding (GGUM) models. When fitting models to data, model misfit can be both a function of misspecification and aberrant responding. Results demonstrate how varying levels of aberrant data can severely impact model fit for both cumulative and ideal point data. Specifically, longstring responses have a stronger impact on dimensionality for both ideal point and cumulative data, while random responding tends to have the most negative impact on data model fit according to information criteria (AIC, BIC). The results also indicate that ideal point data models such as GGUM may be able to fit cumulative data as well as the cumulative model itself (GPCM), whereas cumulative data models may not provide sufficient model fit for data simulated using an ideal point model.
更多查看译文
关键词
response,effects,model-fit
AI 理解论文
溯源树
样例
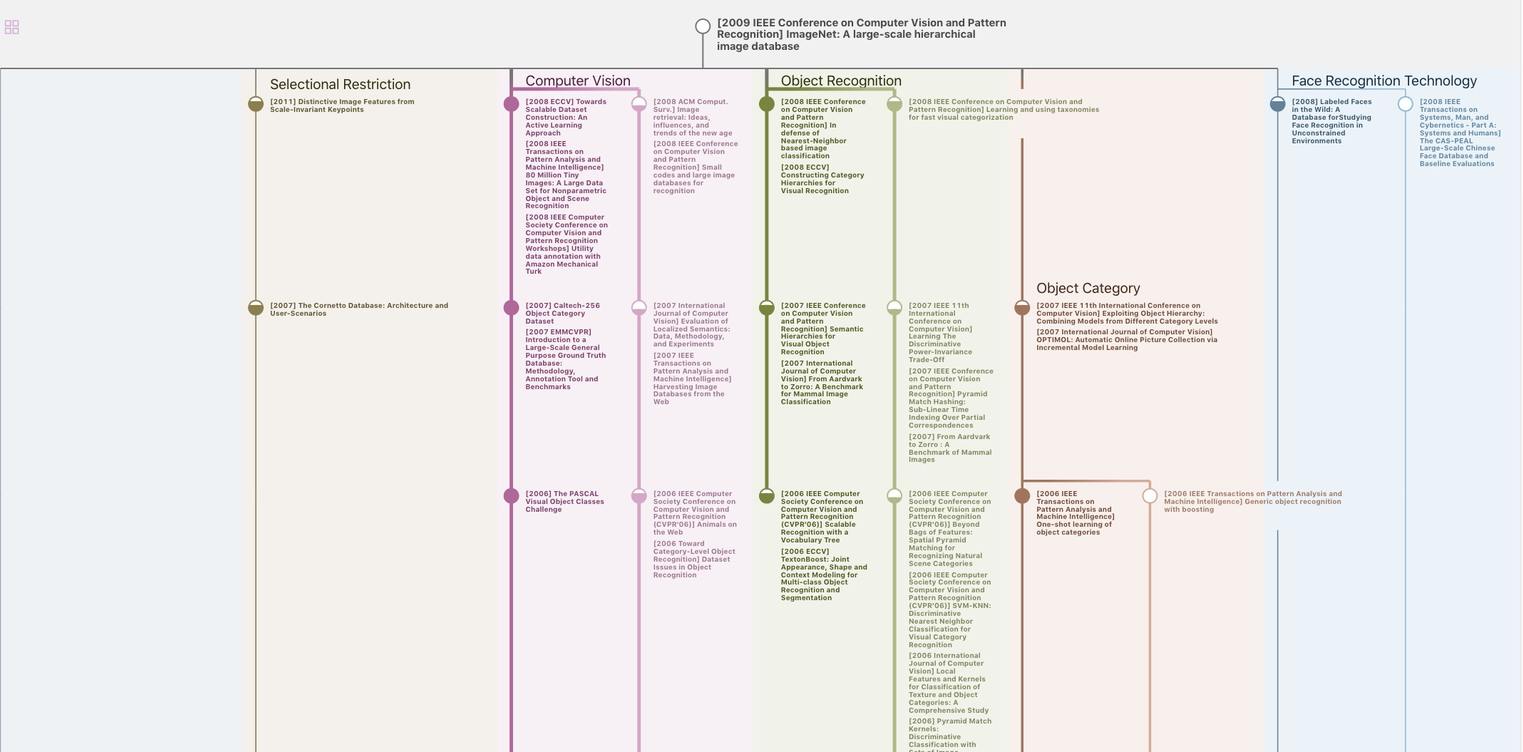
生成溯源树,研究论文发展脉络
Chat Paper
正在生成论文摘要