Enhancing building pattern recognition through multi-scale data and knowledge graph: a case study of C-shaped patterns
INTERNATIONAL JOURNAL OF DIGITAL EARTH(2023)
摘要
Building pattern recognition is important for understanding urban forms, automating map generalization, and visualizing 3D city models. However, current approaches based on object-independent methods have limitations in capturing all visually aware patterns due to the part-based nature of human vision. Moreover, these approaches also suffer from inefficiencies when applying proximity graph models. To address these limitations, we propose a framework that leverages multi-scale data and a knowledge graph, focusing on recognizing C-shaped building patterns. We first employ a specialized knowledge graph to represent the relationships between buildings within and across various scales. Subsequently, we convert the rules for C-shaped pattern recognition and enhancement into query conditions, where the enhancement refers to using patterns recognized at one scale to enhance pattern recognition at other scales. Finally, rule-based reasoning is applied within the constructed knowledge graph to recognize and enrich C-shaped building patterns. We verify the effectiveness of our method using multi-scale data with three levels of detail (LODs) collected from AMap, and our method achieves a higher recall rate of 26.4% for LOD1, 20.0% for LOD2, and 9.1% for LOD3 compared to existing methods with similar precision rates. We also achieve recognition efficiency improvements of 0.91, 1.37, and 9.35 times, respectively.
更多查看译文
AI 理解论文
溯源树
样例
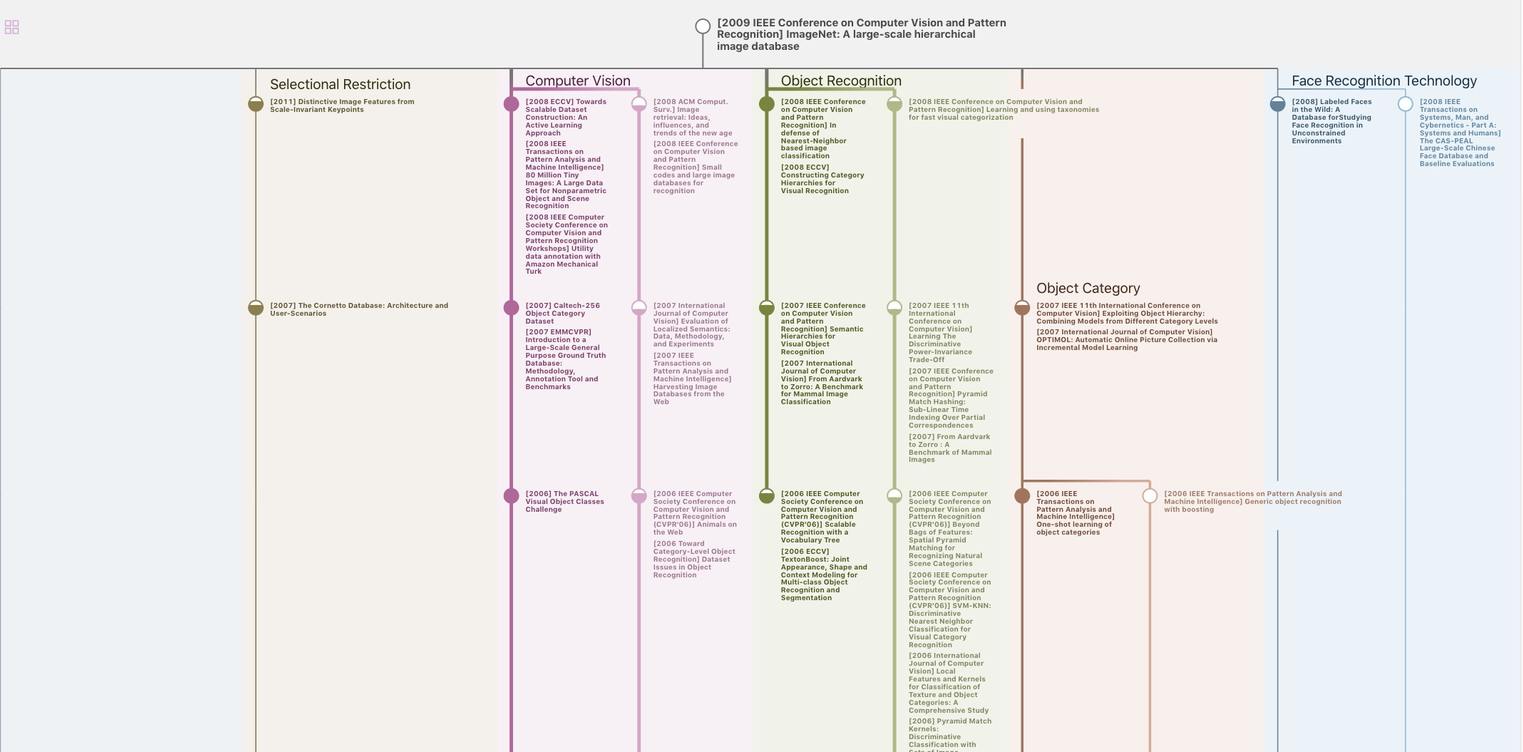
生成溯源树,研究论文发展脉络
Chat Paper
正在生成论文摘要