The co-effect of image resolution and crown size on deep learning for individual tree detection and delineation
INTERNATIONAL JOURNAL OF DIGITAL EARTH(2023)
摘要
Individual tree detection and delineation (ITDD) is an important subject in forestry and urban forestry. This study represents the first research to propose the concept of crown resolution to comprehensively evaluate the co-effect of image resolution and crown size on deep learning. Six images with different resolutions were derived from a DJI Unmanned Aerial Vehicle (UAV), and 1344 manually delineated Chinese fir (Cunninghamia lanceolata (Lamb) Hook) tree crowns were used for six training and validation mask region-based convolutional neural network (Mask R-CNN) models, while additional 476 delineated tree crowns were reserved for testing. The overall detection accuracy, the influence of different crown sizes, and crown resolutions were calculated to evaluate model performance accuracy with different image resolutions for ITDD. Results show that the highest accuracy was achieved when the crown resolution was between 800 and 12800 pixels/tree. The accuracy of ITDD was impacted by crown resolution, and it was unable to effectively identify Chinese fir when the crown resolution was less than 25 pixels/tree or higher than 12800 pixels/tree. The study highlights crown resolution as a critical factor affecting ITDD and suggests selecting the appropriate resolution based on the target detected crown size.
更多查看译文
关键词
Mask R-CNN,instance segmentation,UAV image resolution,crown-like characteristics
AI 理解论文
溯源树
样例
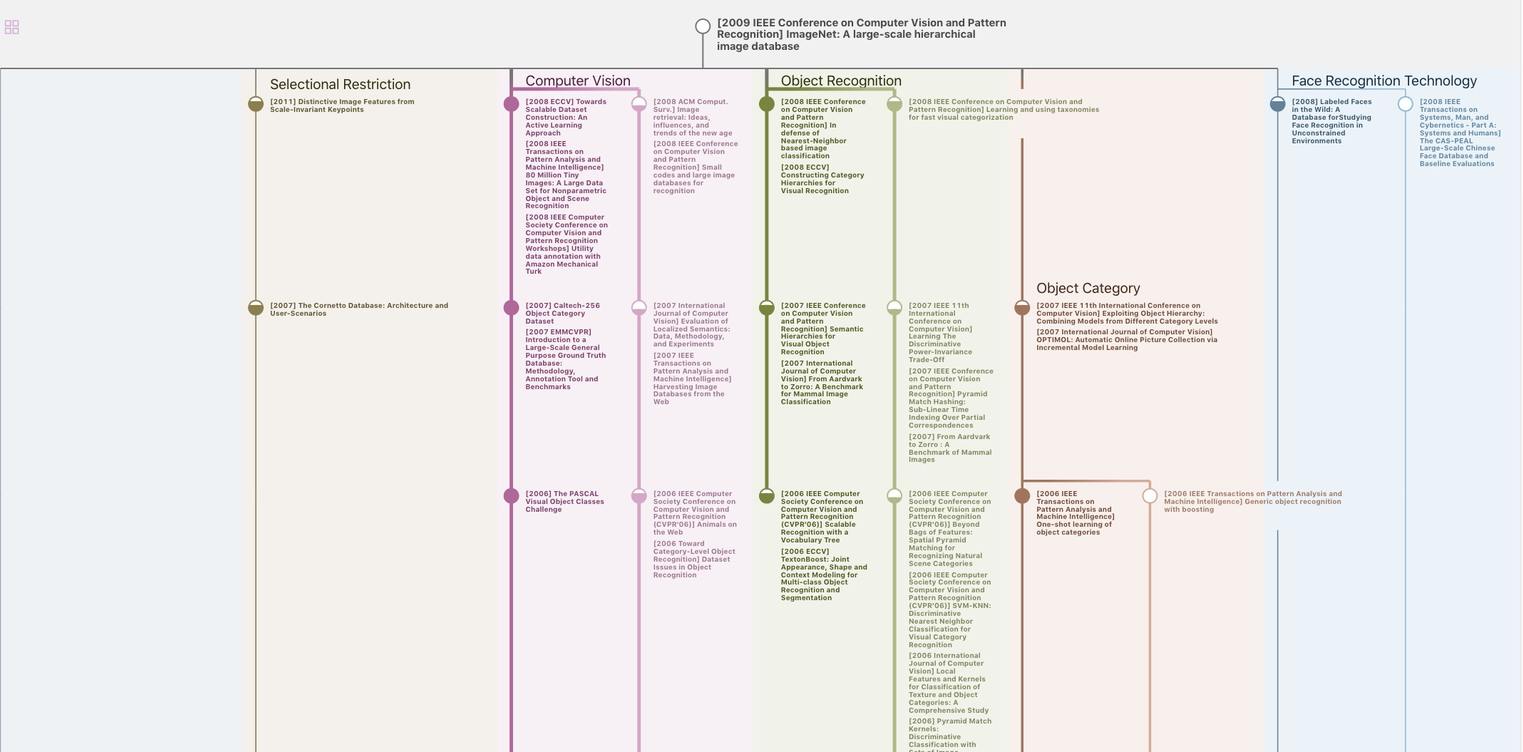
生成溯源树,研究论文发展脉络
Chat Paper
正在生成论文摘要