Water Pressure Optimisation for Leakage Management L sing Q Learning
2023 IEEE CONFERENCE ON ARTIFICIAL INTELLIGENCE, CAI(2023)
摘要
The recent global urbanization problem has set the industry and researchers sights to the importance of safe, effective water distribution due to the unprecedent demand placed on our aging water networks. Our current water practices often increase the degradation of assets through heightened pressures causing more failures and leakage. Whilst the higher network pressures ensure customer demands are met; they cause detrimental failures to the system, long-term expenses, higher carbon emissions and energy consumption. This paper uses a baseline reinforcement learning algorithm to optimize valve set point for active pressure control. Using optimized Q-learning in an EPANET-Python environment, the agent learns to modify valve set points to decrease the average pressures whilst remaining within the OFWAT mandated pressure limits of Him. This code is tested on the d-town test network. The agent shows continuous improvement finding an optimized set point of 26m and dropping the average system pressure by 2% by making simple changes to two pressure reducing valves. The agent learns the optimal actions to take for different states however further improvements can be made through the use of deep neural networks.
更多查看译文
关键词
Reinforcement Learning, Urban Water, Pressure Optimization
AI 理解论文
溯源树
样例
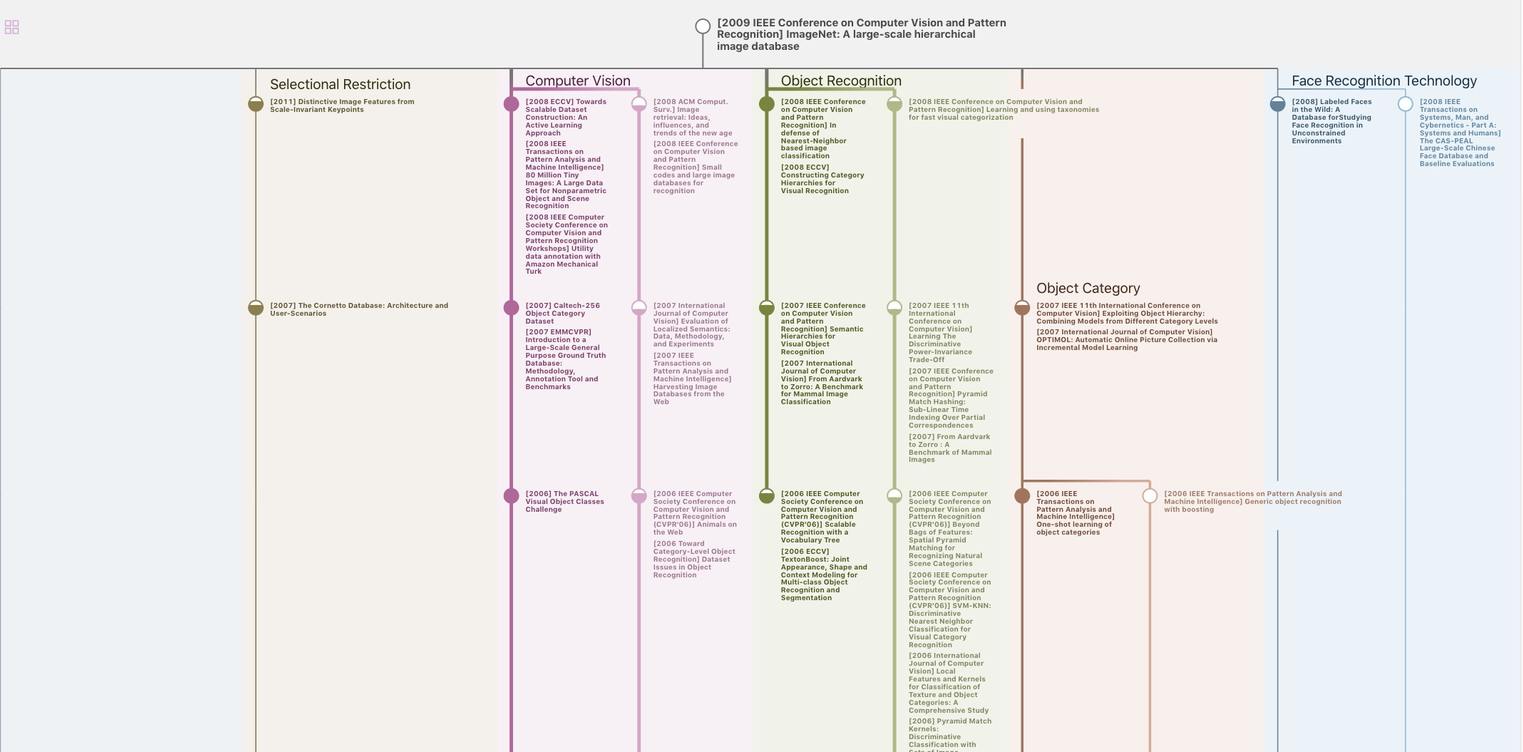
生成溯源树,研究论文发展脉络
Chat Paper
正在生成论文摘要