Reconstructing Centennial-Scale Water Level of Large Pan-Arctic Lakes Using Machine Learning Methods
JOURNAL OF EARTH SCIENCE(2023)
摘要
The pan-Arctic region has the largest number of lakes in the world, which is rather sensitive to changing climate. It is urgently needed to understand how these lakes were changing in the long term. However, there are few lakes with long-term historical monitoring of water level, understanding the hydrologic changes of pan-Arctic lakes over the past century requires the data reconstruction by state-of-art techniques. This study used machine learning algorithms to reconstruct the water level of pan-Arctic lakes on a centennial scale. It further investigated their relationship with long-term hydro-logical and climatic variables. Comparison of the reconstructed results by four different machine learning models shows that the extreme gradient boosting tree (XGBoost) is better than other three models. Overall, the centennial-scale reconstruction using the XGBoost model performs best for most study lakes. Based on the reconstructed results, we can observe that water level changes of several North American lakes are correlated with potential evapotranspiration, followed by precipitation, while the Eurasian lakes are more strongly associated with temperature and wet day frequency. The water level dynamics of pan-Arctic lakes could be largely attributed to Arctic Oscillation and Atlantic Multi-decadal Oscillation. This study is expected to advance our understanding of the pan-Arctic lake water level changes in the past century and to provide a feasible method for reconstructing the regional lake water level in the long term.
更多查看译文
关键词
machine learning,centennial-scale,pan-arctic
AI 理解论文
溯源树
样例
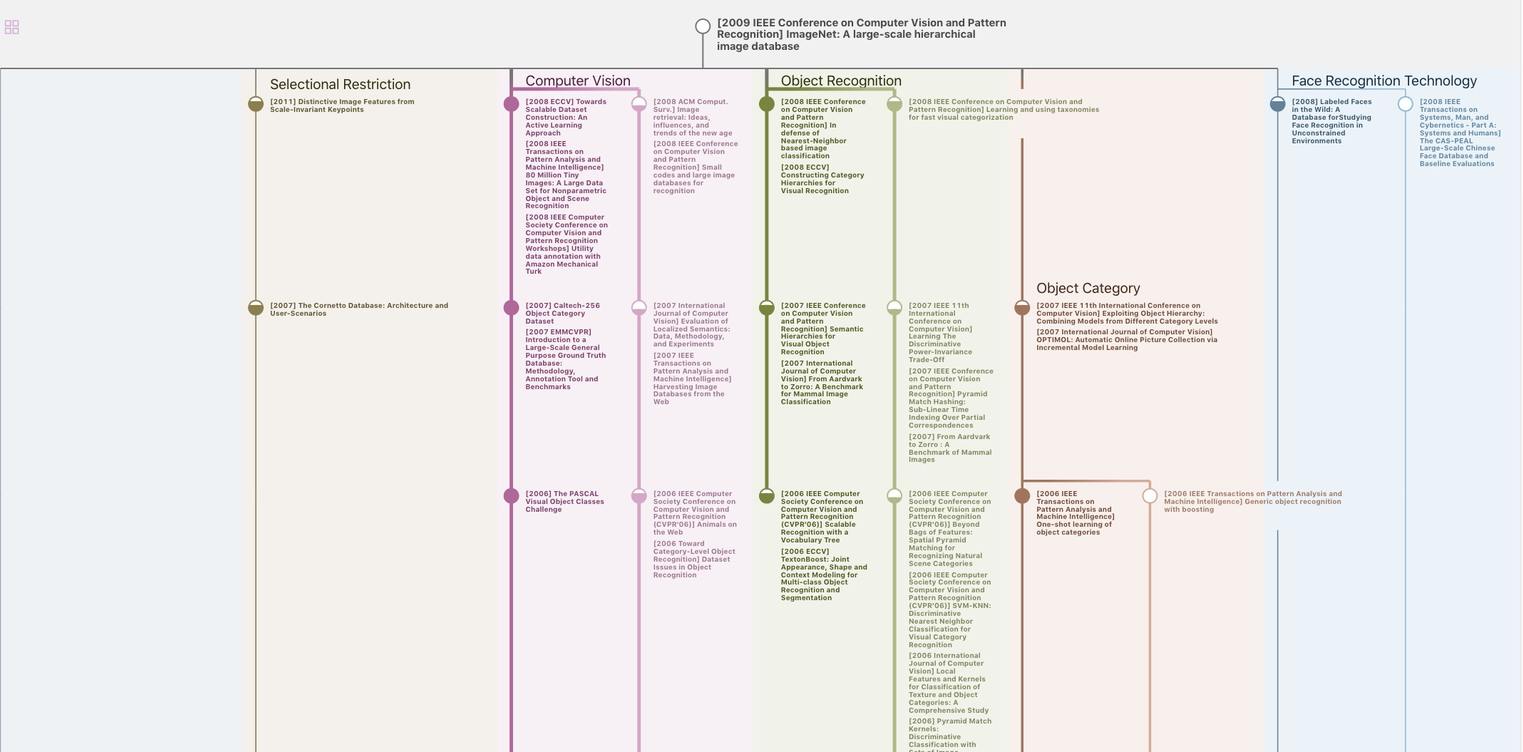
生成溯源树,研究论文发展脉络
Chat Paper
正在生成论文摘要