A Multi-Behavior Recommendation Method for Users Based on Graph Neural Networks
APPLIED SCIENCES-BASEL(2023)
摘要
Most existing recommendation models only consider single user-item interaction information, which leads to serious cold-start or data sparsity problems. In practical applications, a user's behavior is multi-type, and different types of user behavior show different semantic information. To achieve more accurate recommendations, a major challenge comes from being able to handle heterogeneous behavior data from users more finely. To address this problem, this paper proposes a multi-behavior recommendation framework based on a graph neural network, which captures personalized semantics of specific behavior and thus distinguishes the importance of different behaviors for predicting the target behavior. Meanwhile, this model establishes dependency relationships among different types of interaction behaviors under the graph-based information transfer network, and the graph convolutional network is further used to capture the high-order complexity of interaction graphs. The experimental results of three benchmark datasets show that the proposed graph-based multi-behavior recommendation model displays significant improvements in recommendation accuracy compared to the baseline method.
更多查看译文
关键词
graph,networks,multi-behavior
AI 理解论文
溯源树
样例
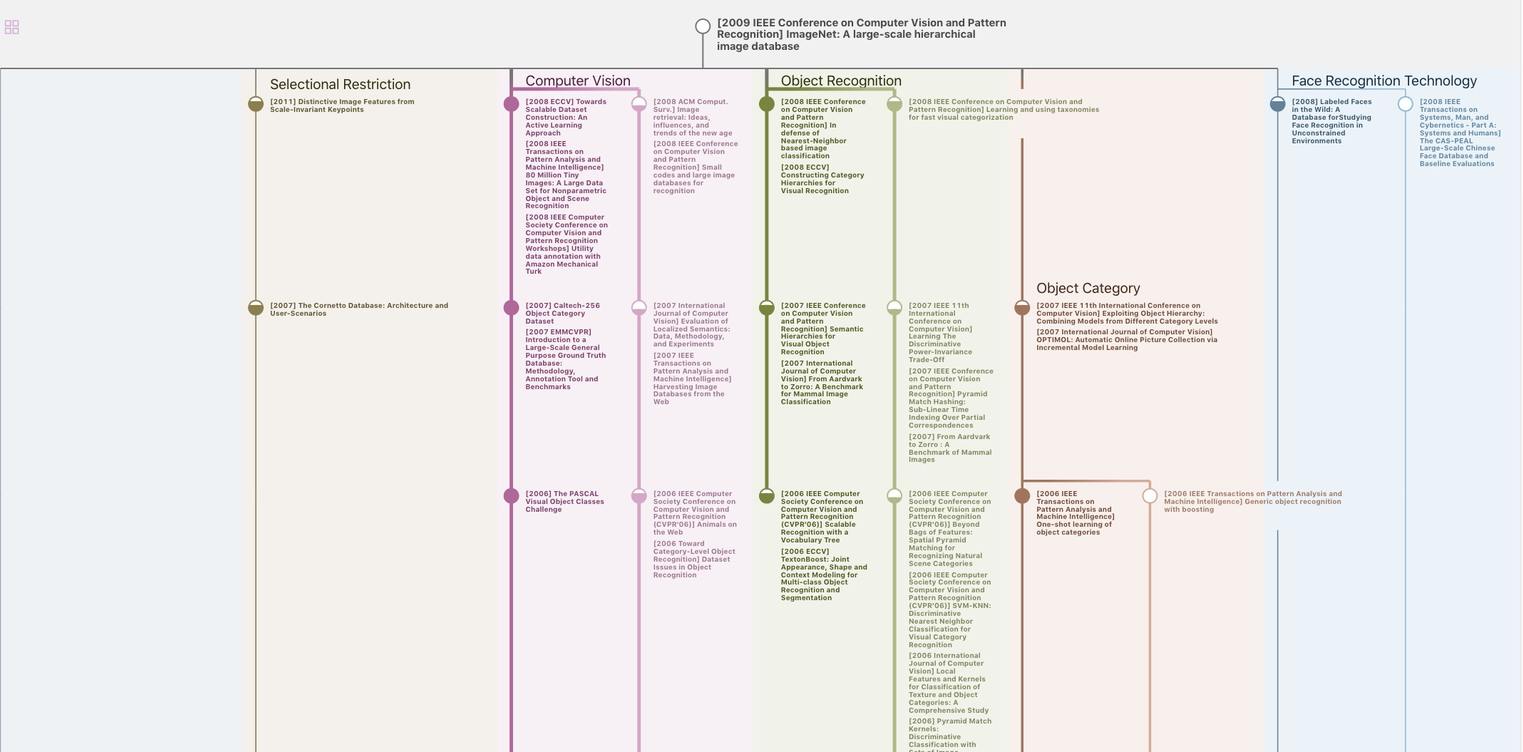
生成溯源树,研究论文发展脉络
Chat Paper
正在生成论文摘要