EUCLID: A New Approach to Constrain Nuclear Data via Optimized Validation Experiments using Machine Learning
15TH INTERNATIONAL CONFERENCE ON NUCLEAR DATA FOR SCIENCE AND TECHNOLOGY, ND2022(2023)
摘要
Compensating errors between several nuclear data observables in a library can adversely impact application simulations. The EUCLID project (Experiments Underpinned by Computational Learning for Improvements in Nuclear Data) set out to first identify where compensating errors could be hiding in our libraries, and then design validation experiments optimized to reduce compensating errors for a chosen set of nuclear data. Adjustment of nuclear data will be performed to assess whether the new experimental data spanning measurements from multiple responses successfully reduced compensating errors. The specific target nuclear data for EUCLID are Pu-239 fission, inelastic scattering, elastic scattering, capture, nu-bar, and prompt fission neutron spectrum (PENS). A new experiment has been designed, which will be performed at the National Criticality Experiments Research Center (NCERC).
更多查看译文
关键词
constrain nuclear data,machine learning,validation experiments
AI 理解论文
溯源树
样例
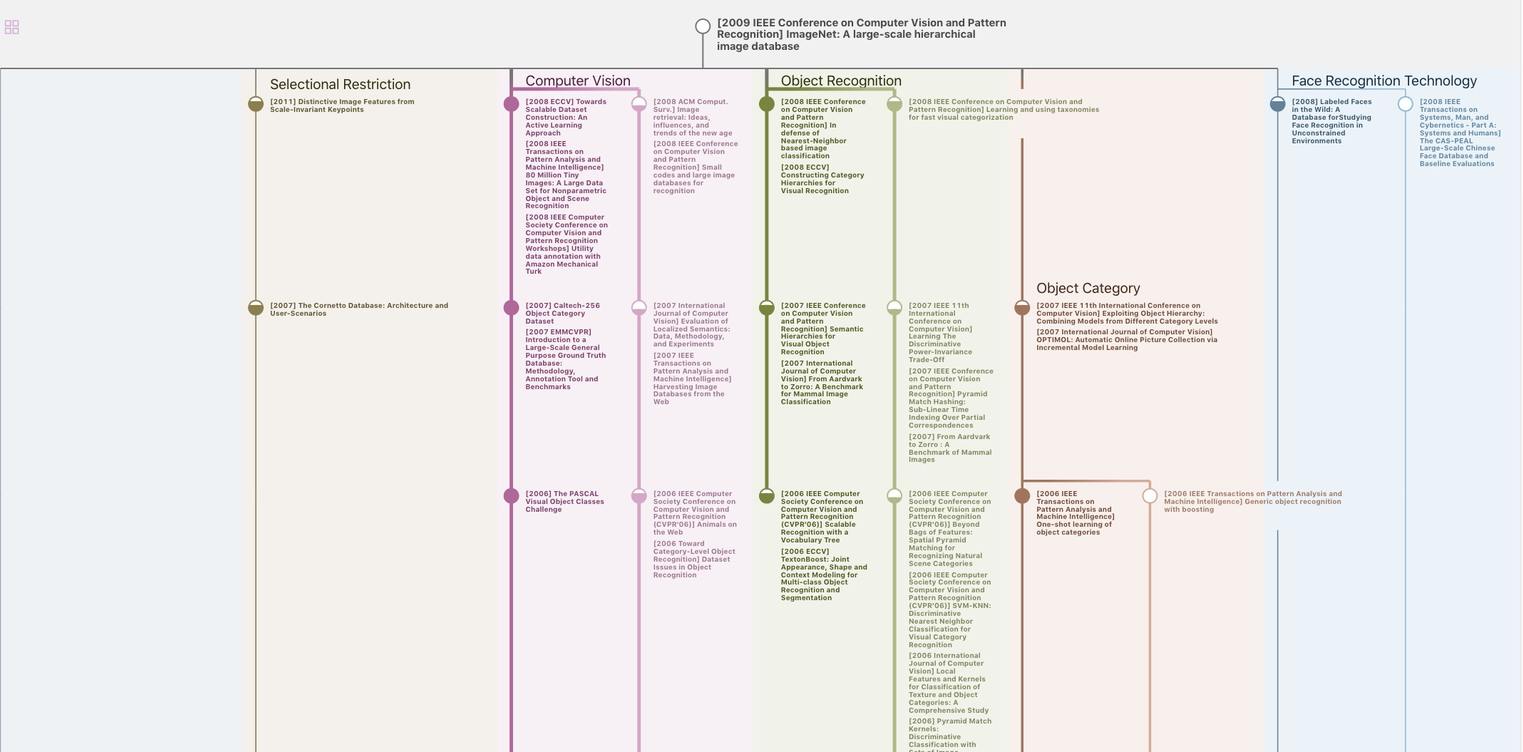
生成溯源树,研究论文发展脉络
Chat Paper
正在生成论文摘要