An Adaptive Soft Sensor Method based on Online Deep Evolving Fuzzy System for Industrial Process Data Streams
2023 IEEE 12TH DATA DRIVEN CONTROL AND LEARNING SYSTEMS CONFERENCE, DDCLS(2023)
摘要
In recent years, deep learning techniques have been widely applied in soft sensor modeling. Stacked autoencoder (SAE) networks are particularly effective at discovering complex data patterns due to their hierarchical structures. However, process data are typically generated as data streams, which poses a great challenge to capture the time-varying characteristics of the process for traditional soft sensor models based on SAE. Furthermore, the insufficiency of offline pre-training data further limits the feature representation capability of SAE. To address these problems, an online deep evolving fuzzy system (ODEFS) based adaptive soft sensor method for process data streams is proposed. In the offline modeling phase, quality-related stacked autoencoder (QSAE) is pre-trained as representation layer to mine quality-related feature representations, while an evolving fuzzy system with self-organization capability is built as the prediction layer. In the online implementation phase, the topology-preserving loss is added to the learning process of QSAE feature network to enable continuous learning of feature representations and alleviate the catastrophic forgetting problem. Meanwhile, the shallow EFS network handles concept drift in data patterns by self-adjusting the structure and parameters. The proposed ODEFS method can improve the feature representation capability of SAE in a data streaming environment and the ability to handle time-varying characteristics, thus ensuring better prediction accuracy. The effectiveness and superiority of the proposed method are verified on TE process.
更多查看译文
关键词
Industrial process data streams,Adaptive soft sensor,Evolving fuzzy system,Stacked autoencoder,Topology preserving loss
AI 理解论文
溯源树
样例
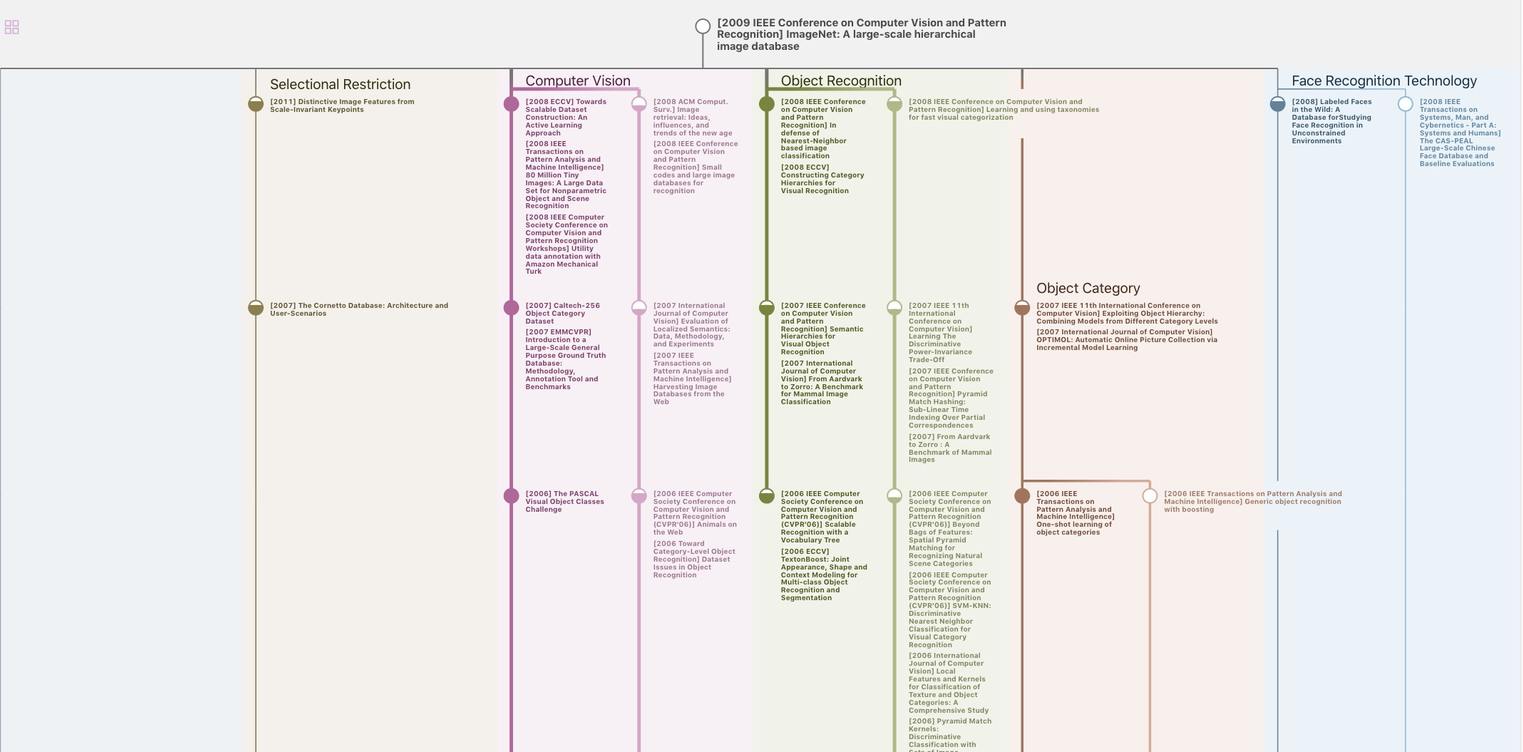
生成溯源树,研究论文发展脉络
Chat Paper
正在生成论文摘要