Reinforcement Learning based Data-driven Optimal Control Strategy for Systems with Disturbance
2023 IEEE 12TH DATA DRIVEN CONTROL AND LEARNING SYSTEMS CONFERENCE, DDCLS(2023)
摘要
This paper proposes a partially model-free optimal control strategy for a class of continuous-time systems in a datadriven way. Although a series of optimal control have achieving superior performance, the following challenges still exist: (i) The controller designed based on the nominal system is difficult to cope with sudden disturbances. (ii) Feedback control is highly dependent on system dynamics and generally requires full state information. A novel composite control method combining output feedback reinforcement learning and input-output disturbance observer for these two challenges is concluded in this paper. Firstly, an output feedback policy iteration (PI) algorithm is given to acquire the feedback gain iteratively. Simultaneously, the observer continuously provides estimates of the disturbance. System dynamic information and states information are not needed to be known in advance in our approach, thus offering a higher degree of robustness and practical implementation prospects. Finally, an example is given to show the effectiveness of the proposed controller.
更多查看译文
关键词
Reinforcement learning,data-driven,input-output data,disturbance observer,adaptive dynamic programming,output feedback
AI 理解论文
溯源树
样例
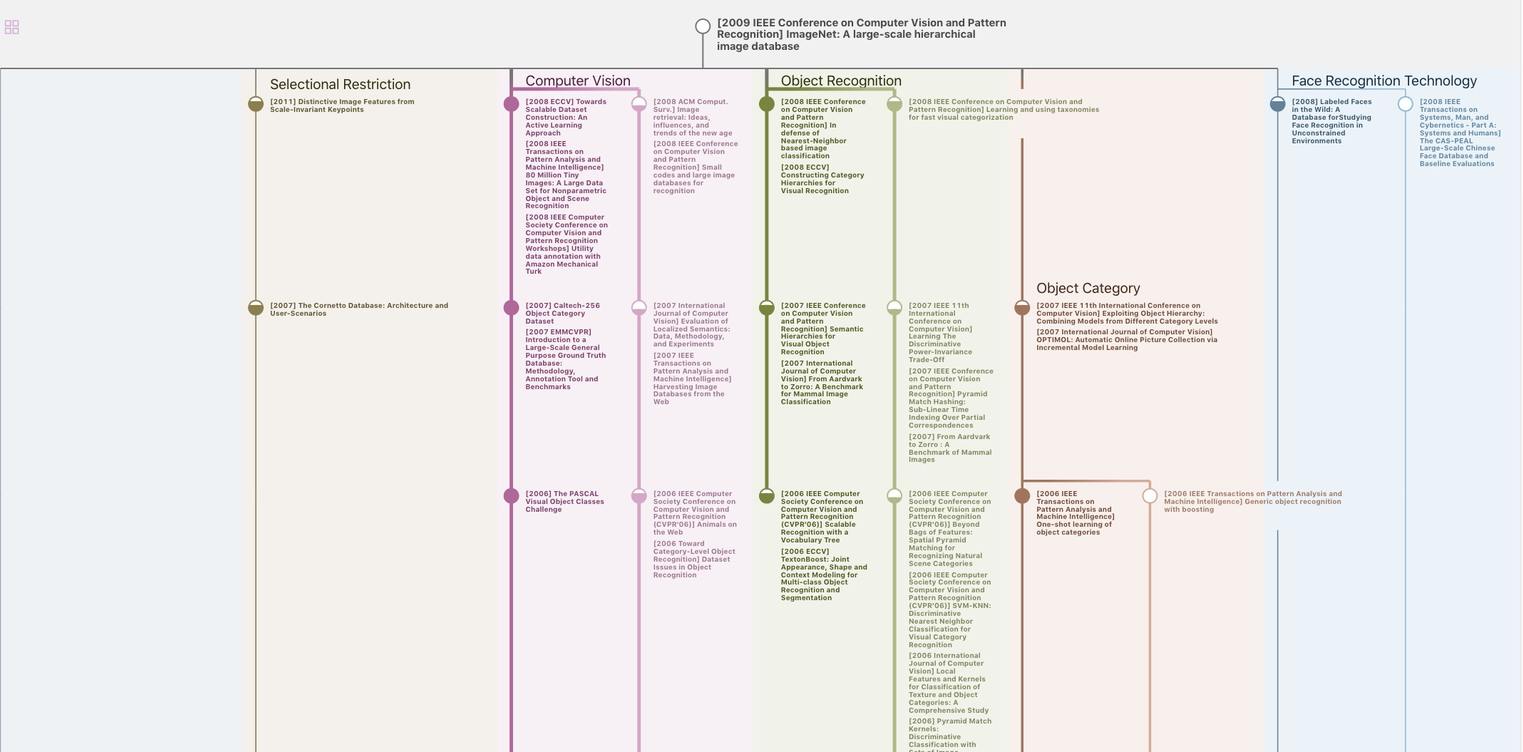
生成溯源树,研究论文发展脉络
Chat Paper
正在生成论文摘要