3D Human Pose Estimation Using Two-Stream Architecture with Joint Training
CMES-COMPUTER MODELING IN ENGINEERING & SCIENCES(2023)
摘要
With the advancement of image sensing technology, estimating 3D human pose from monocular video has become a hot research topic in computer vision. 3D human pose estimation is an essential prerequisite for subsequent action analysis and understanding. It empowers a wide spectrum of potential applications in various areas, such as intelligent transportation, human-computer interaction, and medical rehabilitation. Currently, some methods for 3D human pose estimation in monocular video employ temporal convolutional network (TCN) to extract inter-frame feature relationships, but the majority of them suffer from insufficient inter-frame feature relationship extractions. In this paper, we decompose the 3D joint location regression into the bone direction and length, we propose the TCG, a temporal convolutional network incorporating Gaussian error linear units (GELU), to solve bone direction. It enables more inter-frame features to be captured and makes the utmost of the feature relationships between data. Furthermore, we adopt kinematic structural information to solve bone length enhancing the use of intra-frame joint features. Finally, we design a loss function for joint training of the bone direction estimation network with the bone length estimation network. The proposed method has extensively experimented on the public benchmark dataset Human 3.6M. Both quantitative and qualitative experimental results showed that the proposed method can achieve more accurate 3D human pose estimations.
更多查看译文
关键词
3D human pose, improved TCN, GELU, kinematic structure
AI 理解论文
溯源树
样例
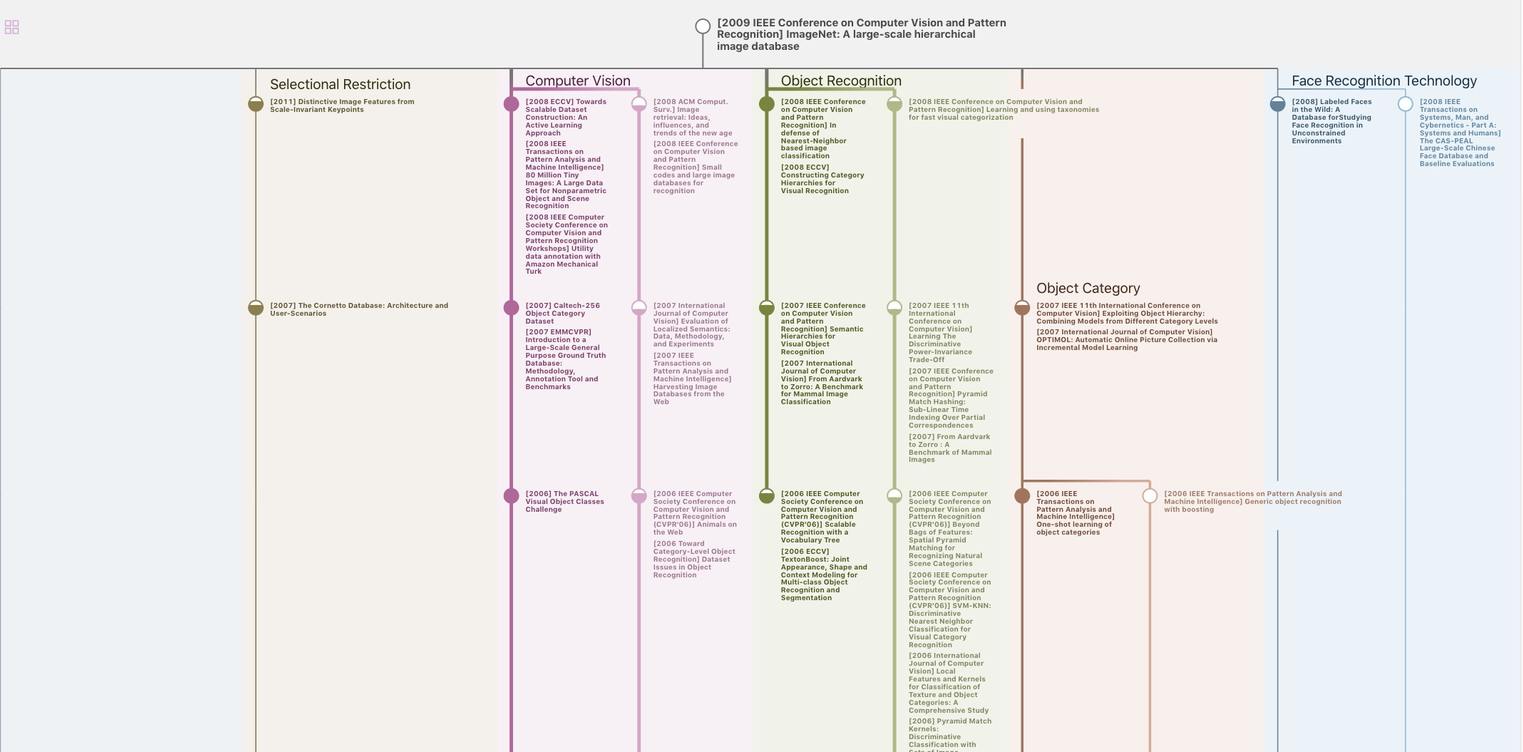
生成溯源树,研究论文发展脉络
Chat Paper
正在生成论文摘要