A Granger causality analysis method based on GRBF network
2023 IEEE 12TH DATA DRIVEN CONTROL AND LEARNING SYSTEMS CONFERENCE, DDCLS(2023)
摘要
Accurate and efficient fault root cause diagnosis is an effective means to prevent major accidents in industrial systems. Due to the difficulty of modeling complex systems, Granger causal analysis is widely used. Root cause diagnosis in the shortest possible time after a fault occurs can improve the accuracy of diagnostic results. Due to the strong nonlinear relationship in the short observation data, this paper introduces Generalized Radial Basis Function(GRBF) neural network of the nonlinear dimensionality reduction method into the Granger causal model to realize the root cause diagnosis of Granger faults based on the nonlinear short observation data. The effectiveness of the proposed method is verified by numerical simulation and fault diagnosis experimental study of Tennessee Eastman,(TE) chemical process. The results show that the proposed method improves the processing ability of Granger causal analysis for nonlinear causality, and can use a small amount of the fault data to complete accurate fault root cause diagnosis.
更多查看译文
关键词
Granger causality,Generalized Radial Basis Function,Root Cause Diagnosis.
AI 理解论文
溯源树
样例
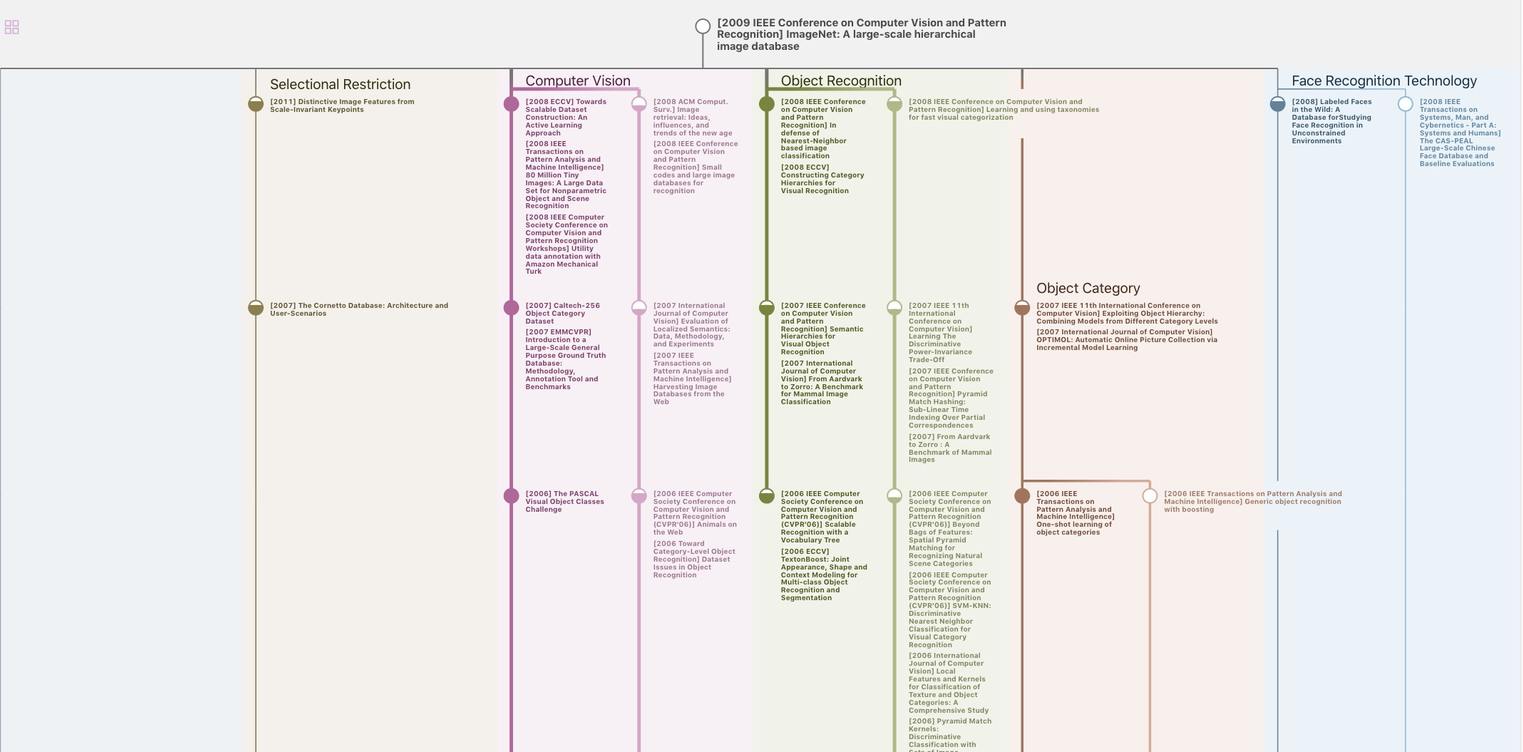
生成溯源树,研究论文发展脉络
Chat Paper
正在生成论文摘要