Gradient-Based Iterative Learning Control for Signal Quantization with Encoding-Decoding Mechanism
2023 IEEE 12TH DATA DRIVEN CONTROL AND LEARNING SYSTEMS CONFERENCE, DDCLS(2023)
摘要
This paper addresses the optimization problem of quantized iterative learning control (ILC) for networked control systems (NCSs) with limited bandwidth. For linear time-invariant systems with quantized input signals, a mathematical cost function is constructed to obtain a gradient-based ILC law that rests with the system model, and the learning gain is updated in the trial domain. By combining the infinite logarithmic quantizer with the encoding and decoding mechanism to encode and decode the signals, the quantization accuracy is enhanced and the system tracking capability is improved. Compared with the traditional gradient descent method with fixed learning gain, the gradient-based ILC law can obtain faster error convergence. Simulation based on industrial robot system is given to substantiate the suggested method.
更多查看译文
关键词
Quantized Iterative Learning Control, Networked Control Systems, Encoding-Decoding Mechanism, Gradient Descent Method
AI 理解论文
溯源树
样例
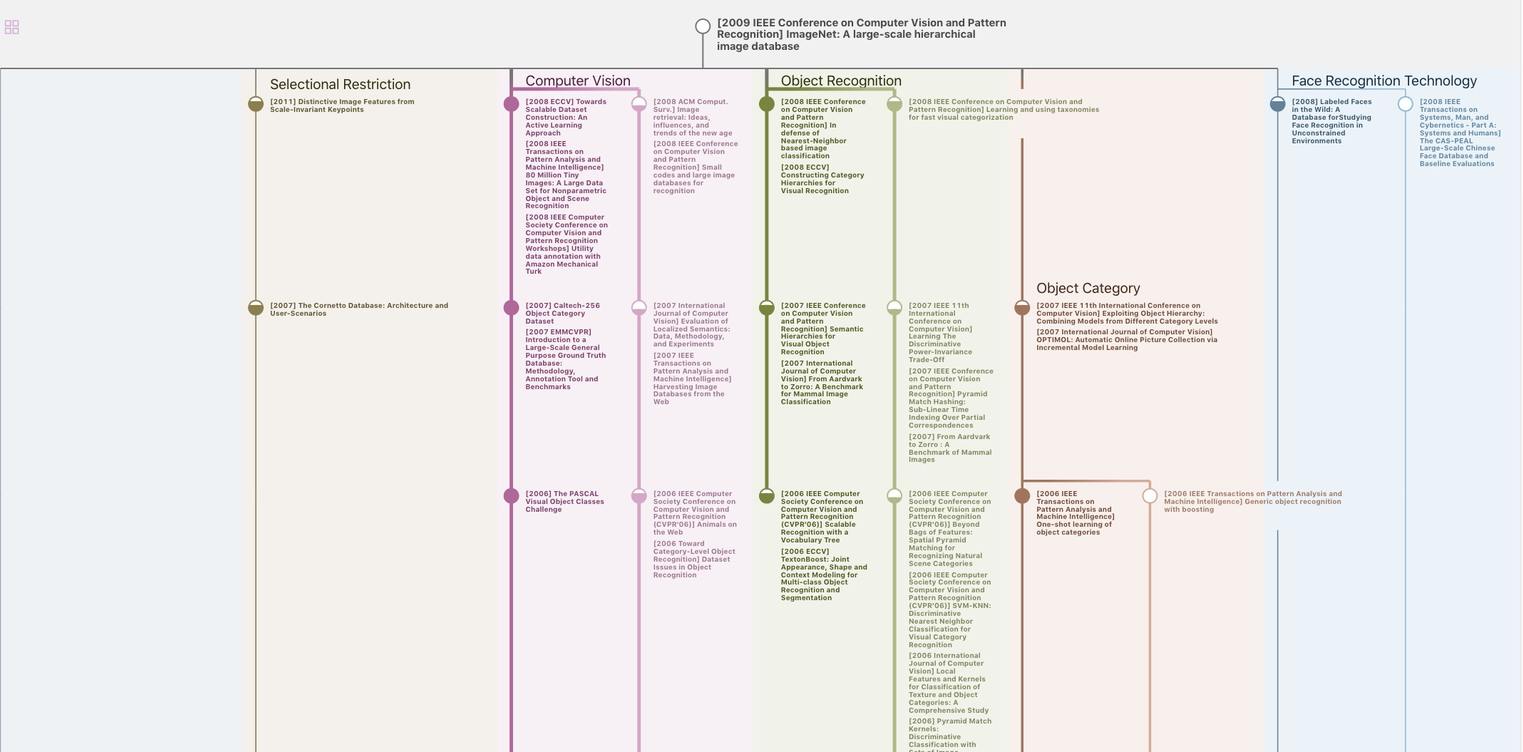
生成溯源树,研究论文发展脉络
Chat Paper
正在生成论文摘要