Rolling Bearing Fault Diagnosis Based on Time-frequency Transform-assisted CNN: A Comparison Study
2023 IEEE 12TH DATA DRIVEN CONTROL AND LEARNING SYSTEMS CONFERENCE, DDCLS(2023)
摘要
This paper is concerned with a comparison study on three time-frequency transform methods for CNN-based rolling bearing fault diagnosis, including short-time Fourier transform (STFT), continuous wavelet transform (CWT), and S-transform. The time-frequency transforms are exploited to transform the bearing fault data from 1D vibration signals to 2D time-frequency images, which are then fed into a dedicatedly designed 2D-CNN for fair performance comparison. To evaluate the performance of the time-frequency transform-assisted CNNs, several experiments are implemented based on the designed CNN and the bearing fault data. The superiority of S-transform assisted CNN is confirmed through the evaluation indicators calculated by the fault diagnostic results.
更多查看译文
关键词
Rolling bearing,Fault diagnosis,Time-frequency transform,Convolutional neural network,CNN
AI 理解论文
溯源树
样例
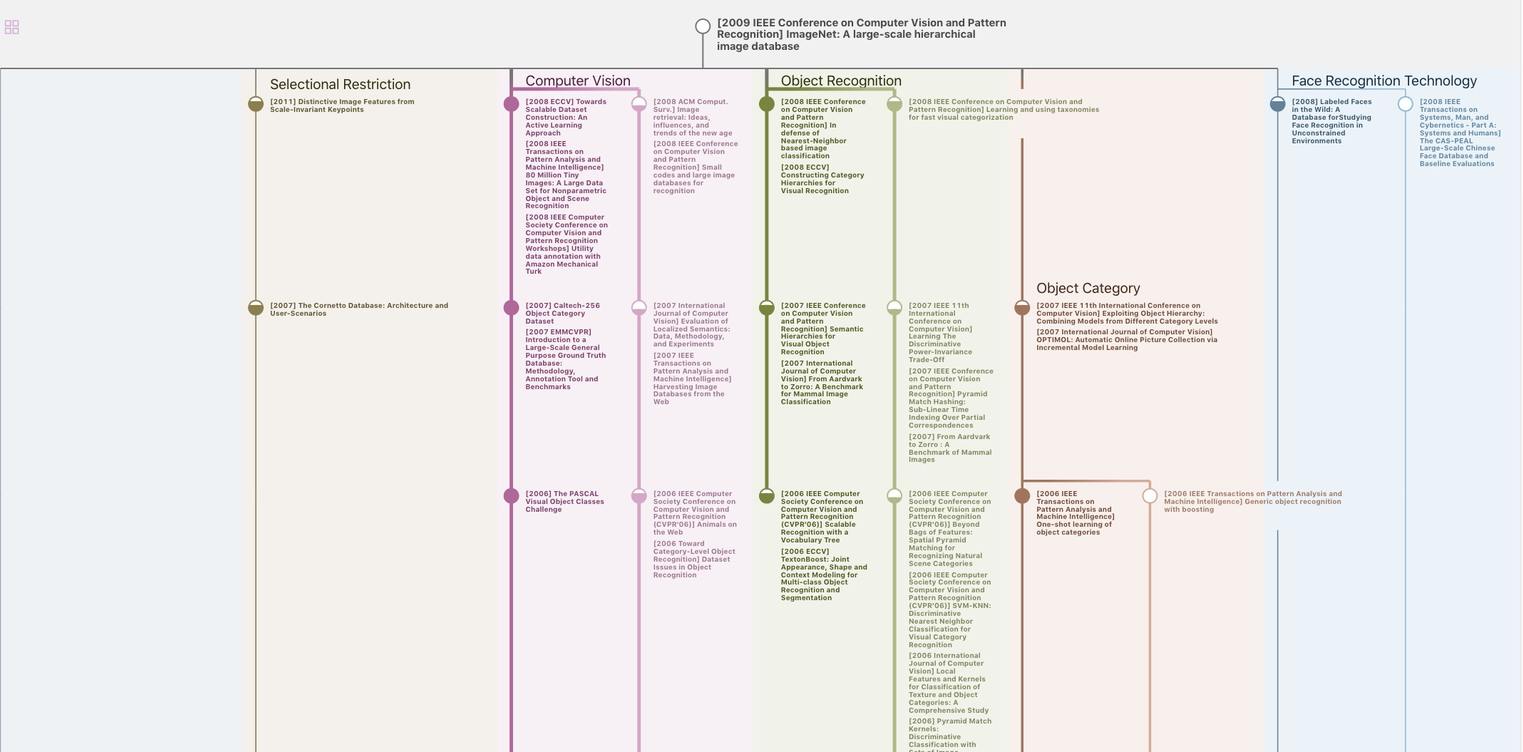
生成溯源树,研究论文发展脉络
Chat Paper
正在生成论文摘要