A Remaining Useful Life Prediction Method With Degradation Model Calibration
2023 IEEE 12TH DATA DRIVEN CONTROL AND LEARNING SYSTEMS CONFERENCE, DDCLS(2023)
摘要
Remaining life prediction (RUL) plays a key role in equipment reliability and safety. Statistical data-driven methods, as typical approaches in the field of remaining life prediction, generally use stochastic models to model the evolution of equipment performance degradation variables and give expressions for the remaining life distribution in the form of probability distributions, which provide great convenience for quantifying the uncertainty of remaining life prediction.In the existing studies on statistical data-driven remaining useful life prediction, degradation modeling is generally performed in the form of a fixed degradation model function. In this case, it is difficult to accurately predict the RUL of equipment by only updating the model parameters when the model function form is not selected properly. This paper proposes a method for predicting the RUL of equipment based on the degradation model calibration. First, a widely used linear Wiener proces based degradation model is initially constructed and the model parameters are updated based on the degradation monitoring data to predict the future degradation trend of equipment. Then, a data-driven parametric error model is established to compensate for the initial degradation model using the prediction error data to calibrate the degradation model function. Concurrently, the parameters of the calibrated degradation model are estimated and updated by using the Bayesian method. As such, the functional form of the degradation model and the associated parameters can be calibrated at the same time. Finally, the superiority of the proposed method is verified by lithium battery data.
更多查看译文
关键词
Remaining useful life,degradation model,model calibration,Bayesian method
AI 理解论文
溯源树
样例
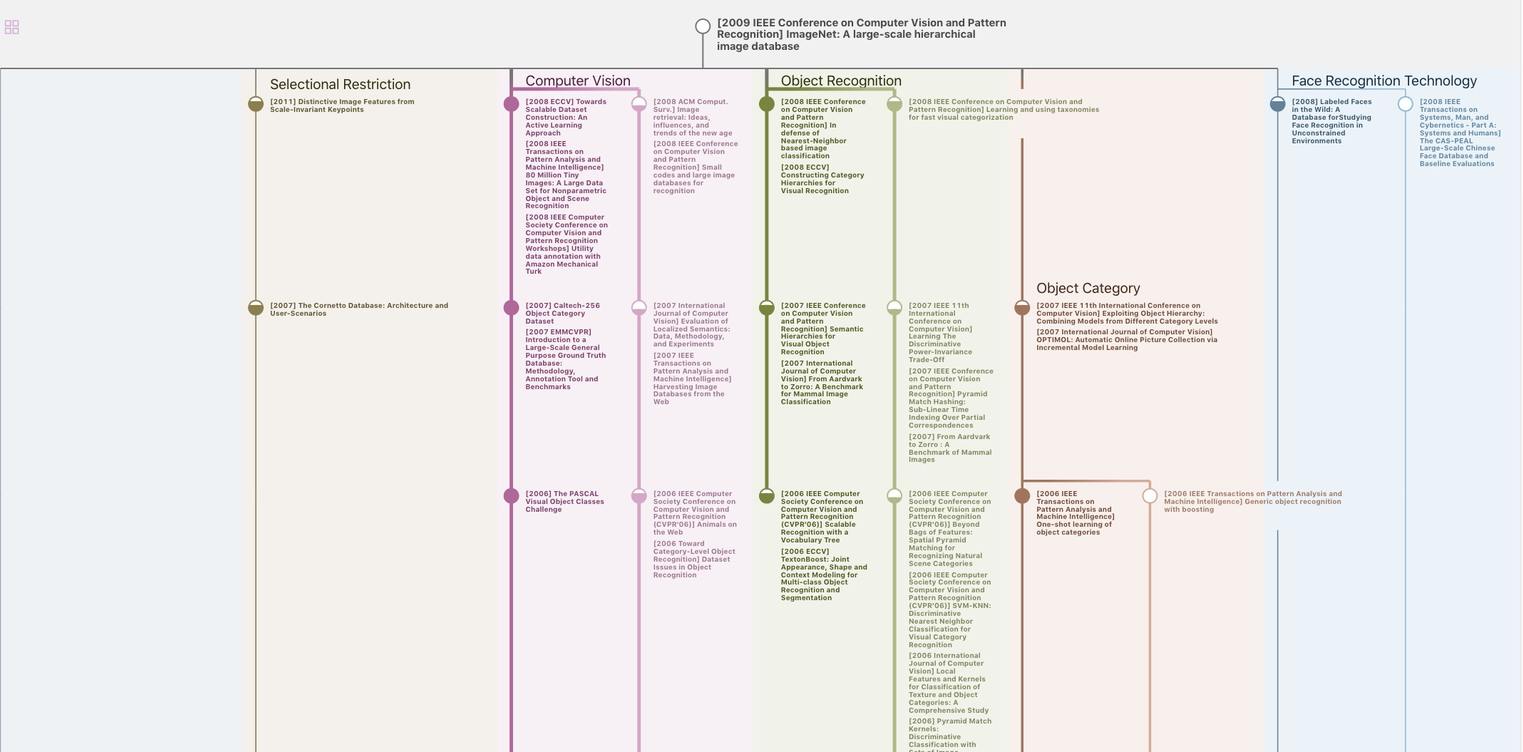
生成溯源树,研究论文发展脉络
Chat Paper
正在生成论文摘要