A Lightweight Pipeline Defect Detection Method via Structural Reparameterization Technique and Ghost Convolution
2023 IEEE 12TH DATA DRIVEN CONTROL AND LEARNING SYSTEMS CONFERENCE, DDCLS(2023)
摘要
To address the inference speed problem of pipeline defect detection, this paper proposes a single-stage lightweight pipeline defect detection method based on the reparameterization technique and ghost convolution. Initially, the baseline model selected for this study is You Only Look Once (YOLO)v5. To further enhance the learning ability without increasing model inference time, the reparameterization convolution (RepConv) is used instead of the 3x3 convolution of the original model. Subsequently, the ghost convolution (GhostConv) is utilized to build the ghost module, which substantially reduces the model's complexity to achieve the trade-off between accuracy and speed. Finally, an efficient channel attention (ECA) is employed in the backbone network and feature fusion stages to improve the model's feature extraction and aggregation capability. After actual experimental tests, the proposed improvement method reduces about 30% in parameters, reduces about 34% in floating point operations (FLOPs), and improves about 1% in accuracy.
更多查看译文
关键词
lightweight,defect detection,re-parameterization technique,ghost convolution,efficient channel attention
AI 理解论文
溯源树
样例
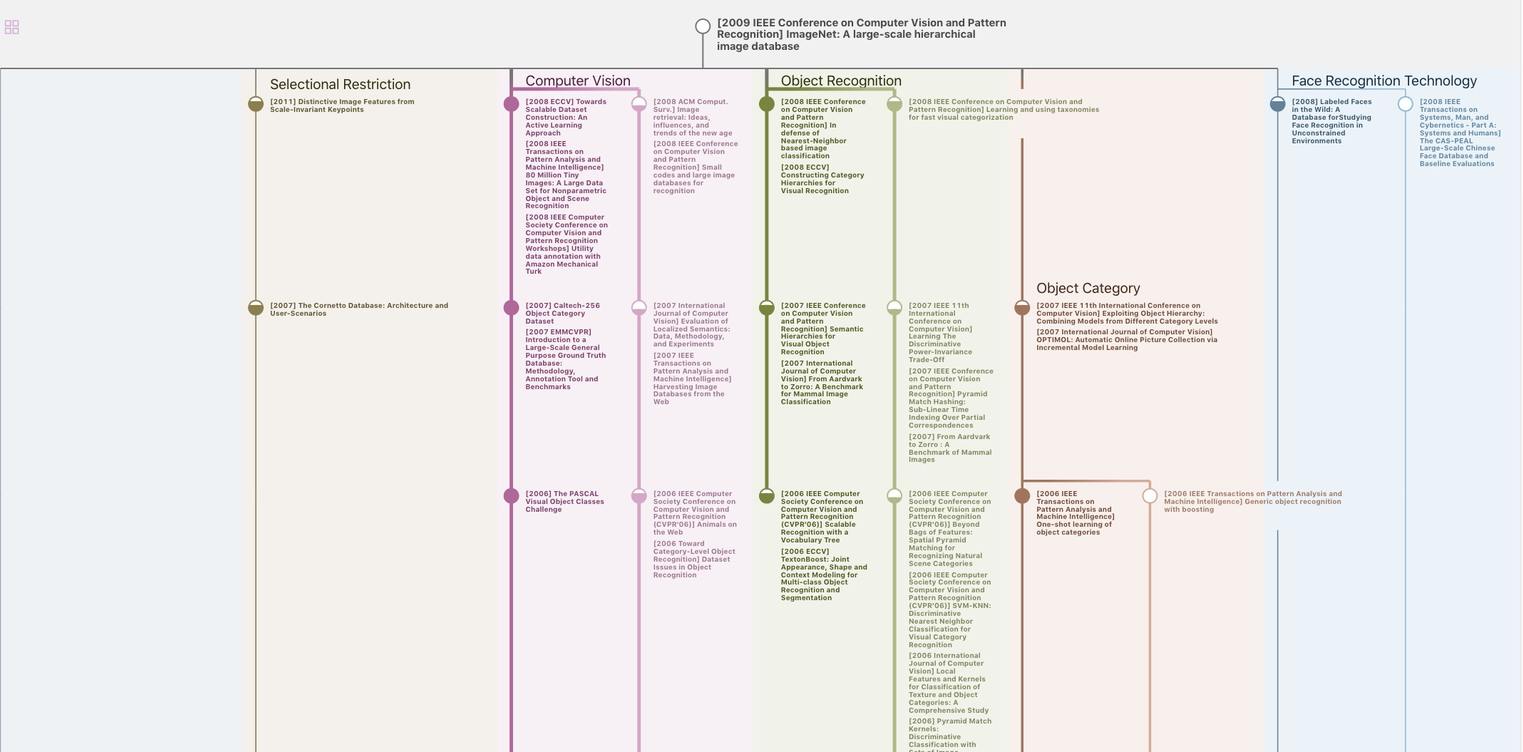
生成溯源树,研究论文发展脉络
Chat Paper
正在生成论文摘要