Multi-scale feature fusion kernel estimation with masked interpolation loss for real-world remote sensing images super-resolution
INTERNATIONAL JOURNAL OF REMOTE SENSING(2023)
摘要
In recent years, the application of deep learning to remote sensing image super-resolution has achieved promising results. However, most deep learning-based methods are often trained on remote sensing datasets constructed by bicubic downsampling, and their recovery effects on real-world remote sensing images are often unsatisfactory. This is because the process of generating low-resolution (LR) images from high-resolution (HR) images to construct training data pairs (LR-HR) using simple bicubic downsampling cannot reflect the degradation process of real-world remote sensing dataset images. In this paper, we propose a multi-scale feature fusion kernel estimation with masked interpolation loss for real-world remote sensing images super-resolution (MFFILSR) to address this problem. MFFILSR is divided into two stages: degenerate kernel estimation and SR network training. In the first stage, we propose a multi-scale feature fusion kernel estimation network that can effectively fuse multi-scale information, making the estimated downsampling kernel closer to the degradation patterns of real-world remote sensing images. In the second stage, we introduce a masked interpolation loss during generator training, by masking the interpolation loss, the artefacts of generated images can be effectively reduced. Extensive experiments show that MFFILSR has satisfactory super-resolution reconstruction performance for real-world remote sensing images.
更多查看译文
关键词
remote sensing images,remote sensing,interpolation loss,fusion,multi-scale,real-world,super-resolution
AI 理解论文
溯源树
样例
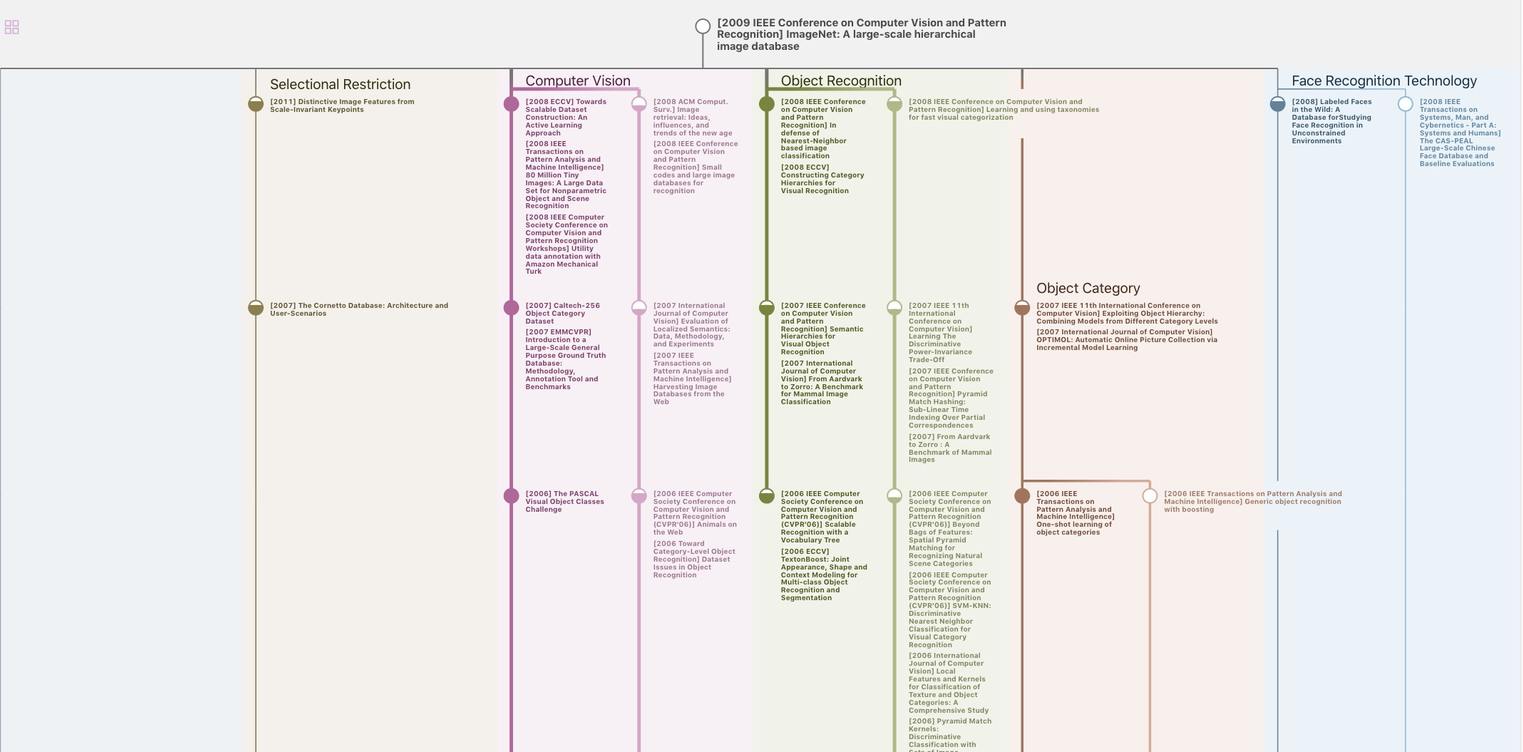
生成溯源树,研究论文发展脉络
Chat Paper
正在生成论文摘要