Machine Learning Guided Interpretation of Point-of-Care Ultrasound to Diagnose Lipohypertrophy
DIABETES(2023)
摘要
Objective: Using a supervised machine learning convolutional neural network (CNN) we developed a model to detect lipohypertrophy (LH) using point-of-care ultrasound (PoCUS) images. Research Designs and Methods: Using a PoCUS ultrasound machine (GE LOGIQe, 5-18 MHz probe), images were obtained by a single, blinded technician. We then split the data set into training (70%), validation (15%) and testing (15%) splits. To improve the generalizability of the model, we used image augmentation techniques (randomly changing contrast, brightness, etc). We considered recall and accuracy of our models when testing the various architectures on our testing dataset. An YOLOv5m object detection model was used to place bounding boxes around LH lesions. Results: The learning architectures investigated were VGG16, ResNet50, DenseNet169, and InceptionV3. The highest recall (76%) and accuracy (76%) was found using the DenseNet CNN architecture. As shown in the figure, YOLOv5m was able to identify LH locations with a F1 score of 0.78 and a confidence value of 0.41. Conclusions: We were able to use a DenseNet CNN architecture to automatically detect the presence of LH lesions on PoCUS images obtained at the bedside. Disclosure K.M.Madden: None. E.Bandari: None. L.Habashy: None. J.Raza: None. J.E.Kapeluto: None. G.S.Meneilly: None. Funding Allan M. McGavin Foundation
更多查看译文
关键词
ultrasound,machine learning guided interpretation,machine learning,point-of-care
AI 理解论文
溯源树
样例
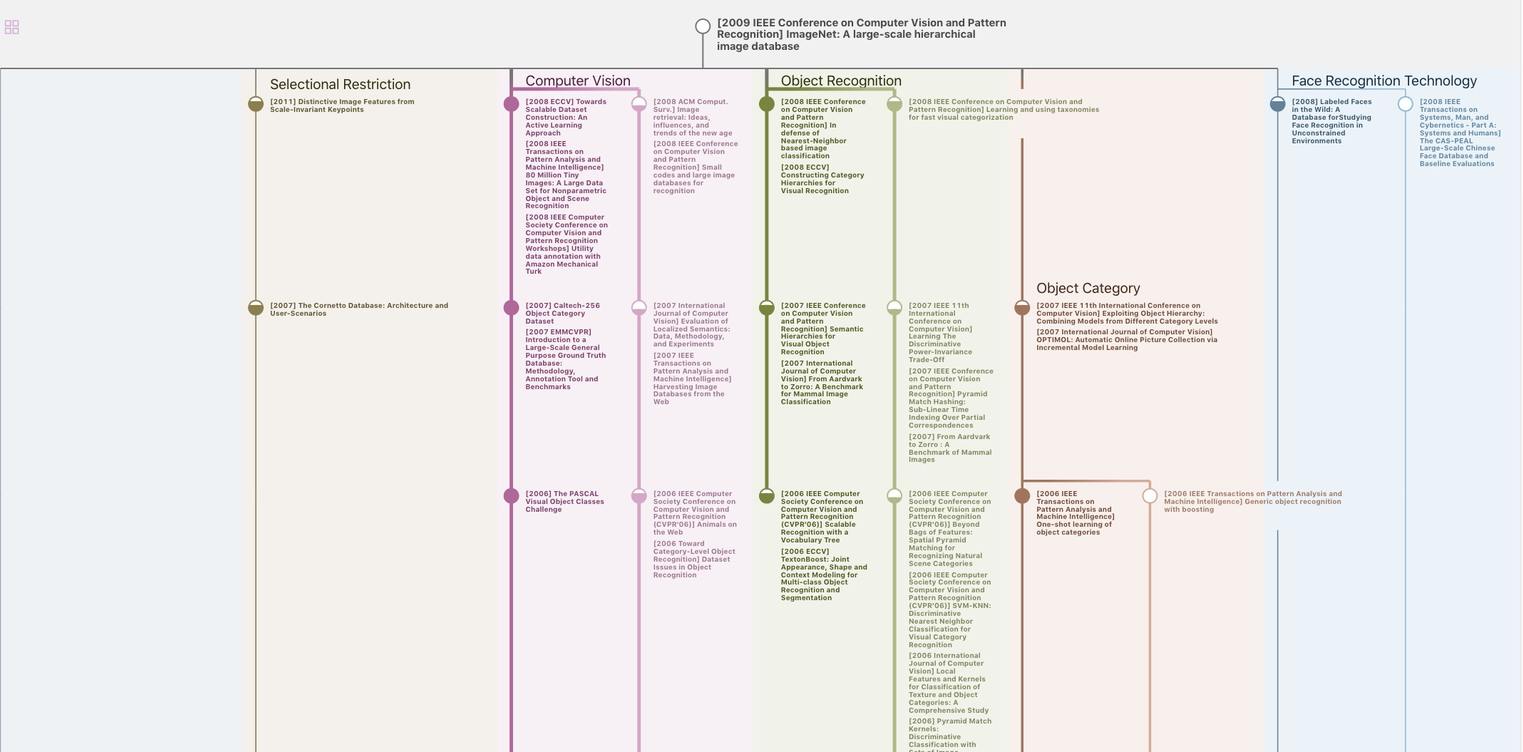
生成溯源树,研究论文发展脉络
Chat Paper
正在生成论文摘要