A Data-Driven Cluster Analysis of Renal Complications in Patients with Type 2 Diabetes-From Phenotypic Observation to Genetic Discovery
DIABETES(2023)
摘要
Diabetic kidney disease (DKD) has a strong genetic component. The marked phenotypic heterogeneity in type 2 diabetes (T2D) limits the power of cross-sectional genome-wide association studies (GWAS) on DKD. We performed a data-driven cluster analysis using the longitudinal electronic health records (EHR) of a prospective cohort to identify subgroups of patients with different patterns of progression of kidney functions. These included 29,416 Chinese patients with T2D recruited in 1995-2018 observed until 2019 (median [IQR] follow-up: 9 [5-12] years). We curated 211 variables including baseline data collected during structured assessment and follow-up EHR data. We applied generalized low rank models (GLRM) to resolve the inter-correlations among variables and reduce the high dimension of the raw dataset. We identified 6 clusters featured by distinct combinations of the 211 variables: 1) DKD-free characterized by stable estimated glomerular filtration rate (eGFR decline <1 ml/min per 1.73 m2 per year) and absence of macroalbuminuria (19.4%); 2) eGFR decline without macroalbuminuria and good glycemic and BP control (26.0%); 3) eGFR decline with albuminuria and high BP (20.1%); 4) eGFR decline with albuminuria and poor glycemic control (14.2%); 5) rapid eGFR decline, albuminuria, high BP and low glucose burden (10.5%); and 6) rapid eGFR decline, macroalbuminuria, high BP and high glucose burden (9.8%). We performed cluster-wise GWAS in 12,358 patients with available genotype data. We found diverse patterns of genetic associations for the 6 DKD clusters reaching genome-wide significance (P GWAS <5×10−8) for cluster 3 and 6. Polygenic risk scores (PRS) using top GWAS loci discriminated risk of end-stage kidney disease with AUC of 0.62 (PRS only) and 0.93 (PRS plus baseline clinical variables). Our novel cluster-GWAS using more precise phenotyping combining baseline and follow-up clinical variables had led to novel genetic discoveries. Disclosure M.Shi: None. J.C.Chan: Board Member; Asia Diabetes Foundation, Consultant; Bayer Inc., Celltrion, Boehringer Ingelheim and Eli Lilly Alliance, Sanofi, Research Support; AstraZeneca, Servier Laboratories, Viatris Inc., Hua Medicine, Merck KGaA, Applied Therapeutics Inc., Lee Powder, Pfizer Inc., Speaker's Bureau; Novartis, Stock/Shareholder; GemVCare Ltd. R.C.Ma: Advisory Panel; AstraZeneca, Merck & Co., Inc., Other Relationship; Bayer Inc., Boehringer-Ingelheim, Research Support; Tricida, Inc., Roche Diagnostics, Novo Nordisk. C.H.Tam: None. E.S.H.Lau: None. A.Yang: None. H.Wu: None. B.Fan: None. A.P.Kong: Advisory Panel; Abbott, Kyowa Kirin Co., Ltd., Speaker's Bureau; Abbott, AstraZeneca, Lilly, Bayer Inc., Boehringer Ingelheim Inc. A.Luk: Research Support; Novo Nordisk, Boehringer-Ingelheim, Bayer Inc., Speaker's Bureau; Eli Lilly and Company. E.Chow: Research Support; Medtronic, Merck KGaA, Speaker's Bureau; Novartis, Bayer Inc., Sanofi. Funding Research Grants Council of the Hong Kong Special Administrative Region (R4012-18); Theme-Based Research Scheme (T12–402/13N)
更多查看译文
关键词
diabetes—from,renal complications,cluster analysis,discovery,data-driven
AI 理解论文
溯源树
样例
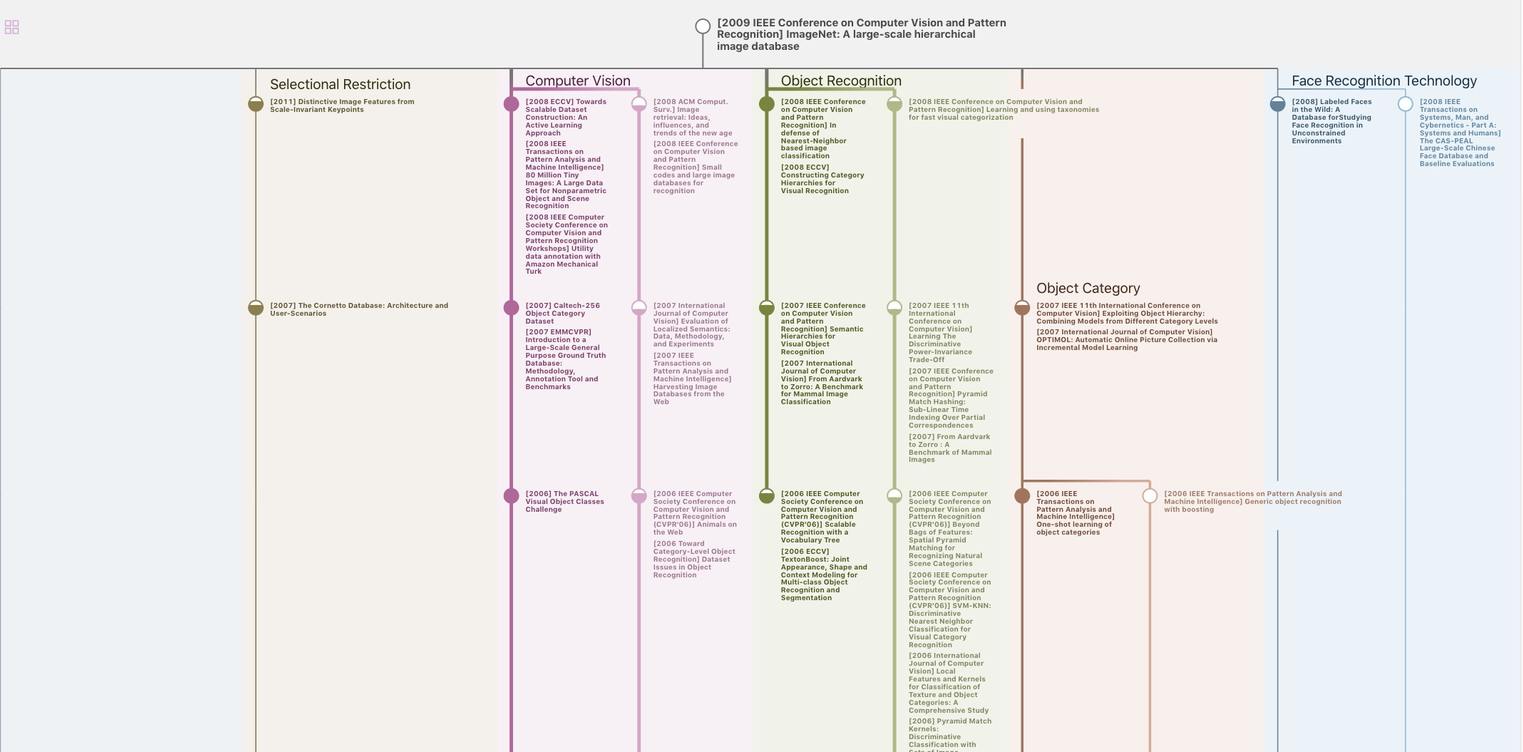
生成溯源树,研究论文发展脉络
Chat Paper
正在生成论文摘要