Adaptive error bounded piecewise linear approximation for time-series representation
ENGINEERING APPLICATIONS OF ARTIFICIAL INTELLIGENCE(2023)
摘要
Error-bounded piecewise linear approximation (l∞-PLA) has been proven effective in addressing challenges in data management and analytics. It works by approximating the original time series with linear segments such that the approximation error on each data point does not exceed a pre-defined threshold. To achieve this goal, most prior works on l∞-PLA use a fixed error threshold during the entire approximation process, assuming a stable time series. However, in many real-world applications, the distribution of values on the time series undergoes substantial changes in different temporal stages. This introduces a need to adaptively tune the error threshold based on the properties of the time series while considering the trade-off between representation error and storage resources. In this work, we propose a general framework for constructing l∞-PLA with adaptive error bounds (AEPLA). It works by dividing the original time series into a set of intervals and assigns adaptive error bounds to these sub-sequences based on their fluctuation levels. Next, these sub-sequences are approximated using a user-defined l∞-PLA method. We implement this framework by employing three different types of l∞-PLA methods and evaluate the performance of our approach on an extensive set of real-world time-series datasets from industrial and scientific domains. Our experiments show that constructing l∞-PLA using the AEPLA framework can provide better a trade-off between representation error and storage resources and achieves lower time and space costs than applying the original l∞-PLA methods.
更多查看译文
关键词
Time series,Data representation,Piecewise linear approximation,Adaptive error bounds
AI 理解论文
溯源树
样例
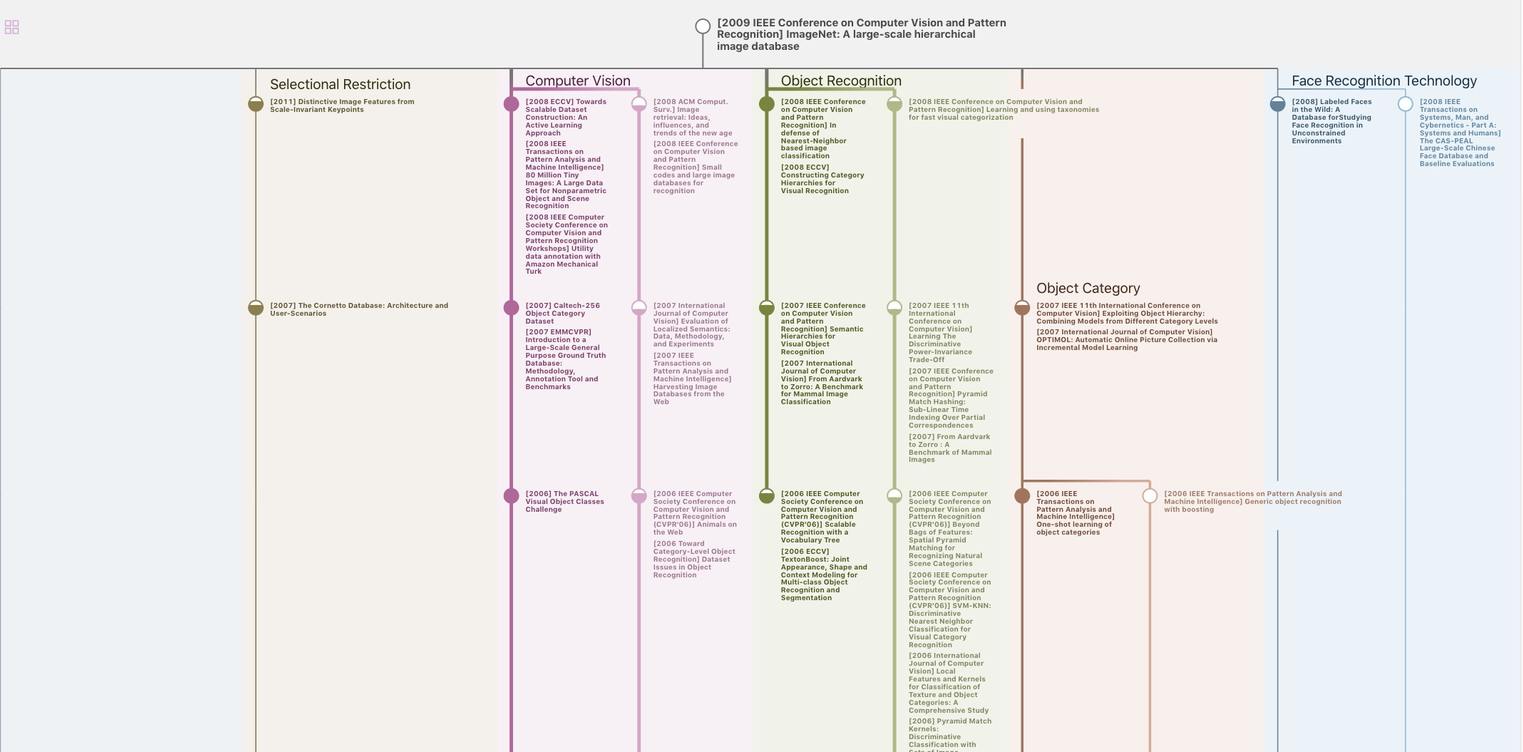
生成溯源树,研究论文发展脉络
Chat Paper
正在生成论文摘要