Multiairport Departure Scheduling via Multiagent Reinforcement Learning
IEEE INTELLIGENT TRANSPORTATION SYSTEMS MAGAZINE(2024)
摘要
With the sharp increase in air traffic demand, the terminal area near airports encounters severe saturation, leading to unprecedented safety concerns. Departure scheduling is the paramount commitment to reduce terminal area congestion. To meet the excessive traffic flow, collaborative departure scheduling by several cooperative airports of a multiairport system has become a new trend for future air transportation. In this article, a multiobjective collaborative departure scheduling model is formulated, in which the objectives are to simultaneously reduce flight delays and scheduling chaos, subject to safety separation constraints. To solve the large-scale multiobjective combinatorial optimization problem in real time, a decomposition-based multiagent reinforcement learning approach (MARL/D) is developed. The fundamental motivation is to extract scheduling knowledge by training the MARL/D on historical scheduling data. First, the action is decomposed along the time dimension to promote the exploration ability. Second, a novel multiobjective reward function is developed, where the aggregation weights are generated by decomposing two conflicting objective functions. Extensive simulation experiments are conducted on real air traffic datasets in the Beijing-Tianjin-Hebei region of northern China. The results demonstrate that the proposed algorithm can effectively reduce flight delays and departure sequence chaos. Compared with mathematical solutions, the proposed algorithm can achieve similar performance with a considerably faster speed.
更多查看译文
关键词
Airports,Collaboration,Delays,Safety,Linear programming,Atmospheric modeling,Chaos
AI 理解论文
溯源树
样例
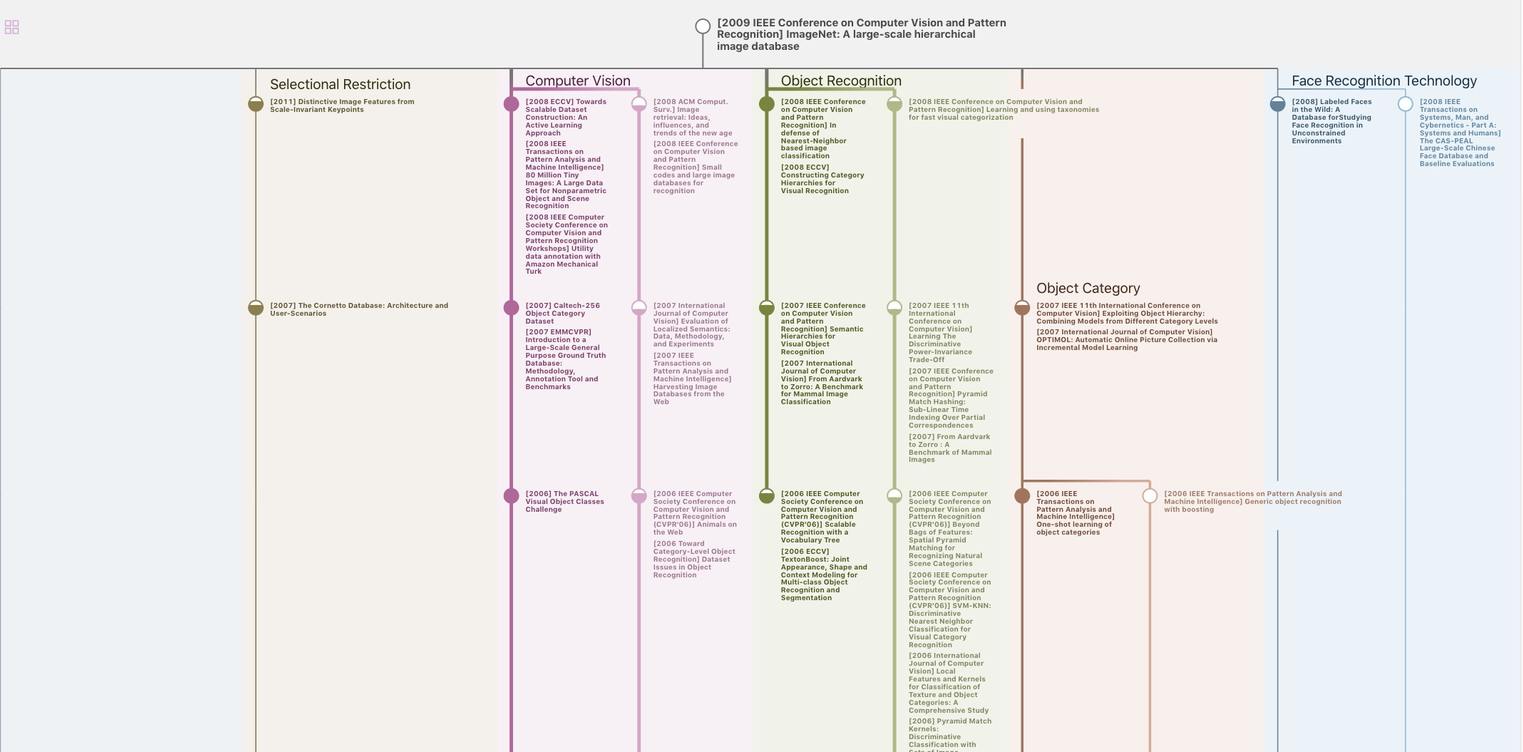
生成溯源树,研究论文发展脉络
Chat Paper
正在生成论文摘要