Attention-Based Personalized Compatibility Learning for Fashion Matching
APPLIED SCIENCES-BASEL(2023)
摘要
The fashion industry has a critical need for fashion compatibility. Modeling compatibility is a challenging task that involves extracting (in)compatible features of pairs, obtaining compatible relationships between matching items, and applying them to personalized recommendation tasks. Measuring compatibility is a complex and subjective concept in general. The complexity is reflected in the fact that relationships between fashion items are determined by multiple matching rules, such as color, shape, and material. Each personal aesthetic style and fashion preference differs, adding subjectivity to the compatibility concept. As a result, personalized factors must be considered. Previous works mainly utilize a convolutional neural network to measure compatibility by extracting general features, but they ignore fine-grained compatibility features and only model overall compatibility. We propose a novel neural network framework called the Attention-based Personalized Compatibility Embedding Network (PCE-Net). It comprises two components: attention-based compatibility embedding modeling and attention-based personal preference modeling. In the second part, we utilize matrix factorization and content-based features to obtain user preferences. Both pieces are jointly trained using the BPR framework in an end-to-end method. Extensive experiments on the IQON3000 dataset demonstrate that PCE-Net significantly outperforms most baseline methods.
更多查看译文
关键词
fashion analysis,personalized compatibility embedding modeling,attention mechanism,multi-modal
AI 理解论文
溯源树
样例
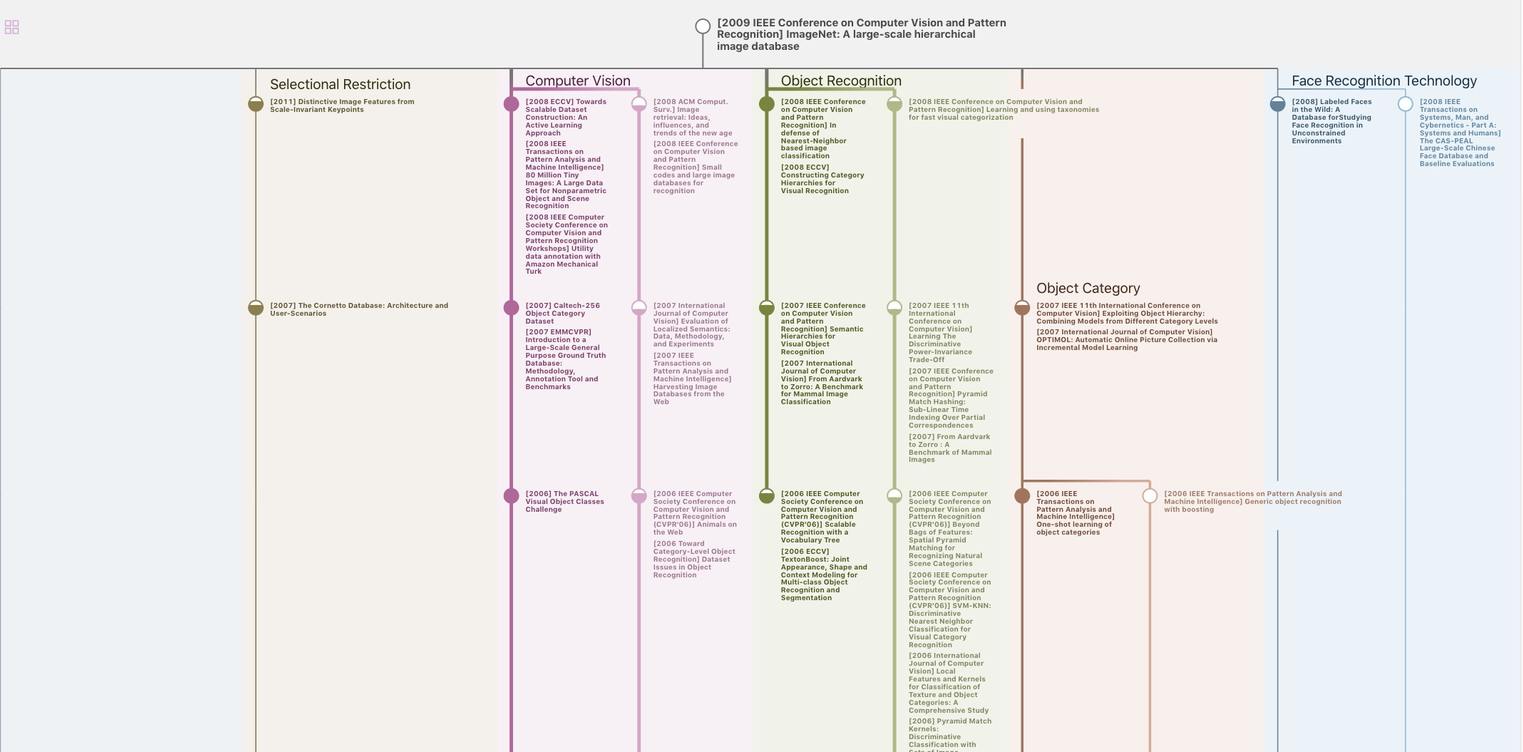
生成溯源树,研究论文发展脉络
Chat Paper
正在生成论文摘要