Parameter-Optimal-Gain-Arguable Iterative Learning Control for Linear Time-Invariant Systems with Quantized Error
APPLIED SCIENCES-BASEL(2023)
摘要
In this paper, a parameter optimal gain-arguable iterative learning control algorithm is proposed for a class of linear discrete-time systems with quantized error. Based on the lifting model description for ILC systems, the iteration time-variable derivative learning gain in the algorithm is optimized by resolving a minimization problem regarding the tracking error energy and the learning effort amplified by a weighting factor. Further, the tracking error can be monotonically convergent to zero when the condition is guaranteed and the rate of convergence can be adjusted by scaling the weighting factor of an optimization problem. This algorithm is more innovative when compared with the existing iterative learning control algorithm for quantization systems. The innovations of this algorithm are as follows: (i) this optimization-based strategy for selecting learning gains can improve the active learning ability of the control mechanism and avoid the passivity of existing selective learning gains; (ii) the algorithm of POGAILC with data quantization can improve the convergence performance of tracking errors and reduce the negative effects of data quantization on the control performance of the logarithmic quantizer; and (iii) we provide a rigorous algorithm convergence analysis by deriving the existence of the unique solution for the optimal learning-gain vector under a singular and nonsingular tracking-error diagonalized matrix. Finally, numerical simulations are used to demonstrate the effectiveness of the algorithm.
更多查看译文
关键词
iterative learning control,parameter-optimal-gain-arguable,time-invariant
AI 理解论文
溯源树
样例
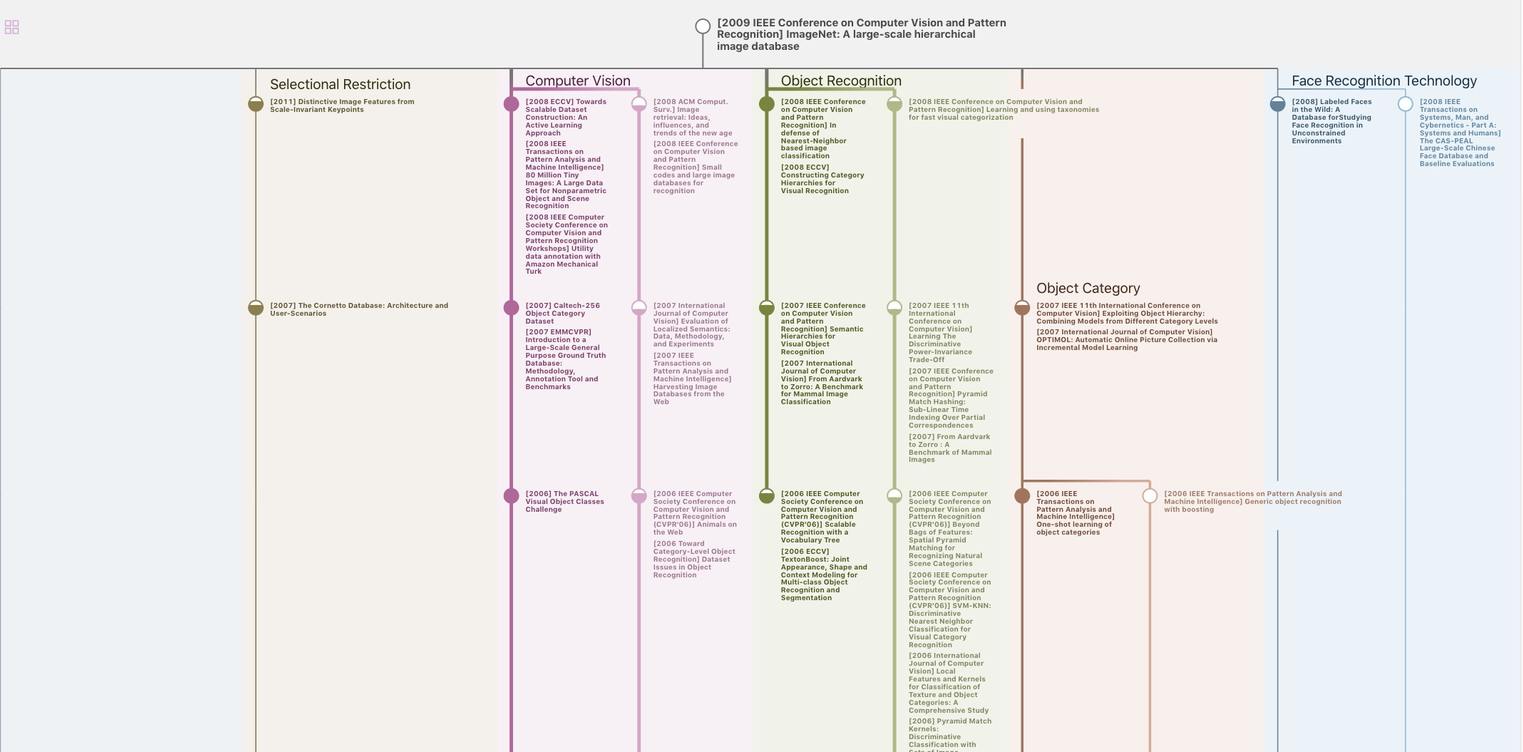
生成溯源树,研究论文发展脉络
Chat Paper
正在生成论文摘要