An ontological architecture for context data retrieval and ranking using SVM and DNN
JOURNAL OF INFORMATION & OPTIMIZATION SCIENCES(2023)
摘要
Context retrieval and ranking have always been an area of interest for researchers around the world. The ranking provides significance to the data that has to be presented in front of users but it also consumes time if the ranking architecture is not organized. The retrieval is dependent upon the co-relation among the data attributes that are supplied against a class label also referred to as ground truth and the ranking depends upon the sensing polarity that indicates the hold of the outcome towards asked information. This paper illustrates an ontological architecture that involves two phases namely context retrieval and ranking. The ranking phase is composed of three different algorithm architectures namely k-means, Support Vector Machines (SVM), and Deep Neural Networks (DNN). The DNN is tuned to fit and work as per the availability of a total number of samples. The proposed work has been evaluated for both quantitative and qualitative parameters in different sets and scenarios. The proposed work has also been compared with other state of art techniques and is illustrated in the paper itself.
更多查看译文
关键词
Context retrieval, Ranking, Ontology, Artificial intelligence, Trapdoor, Machine learning, Support vector machine, Deep neural network
AI 理解论文
溯源树
样例
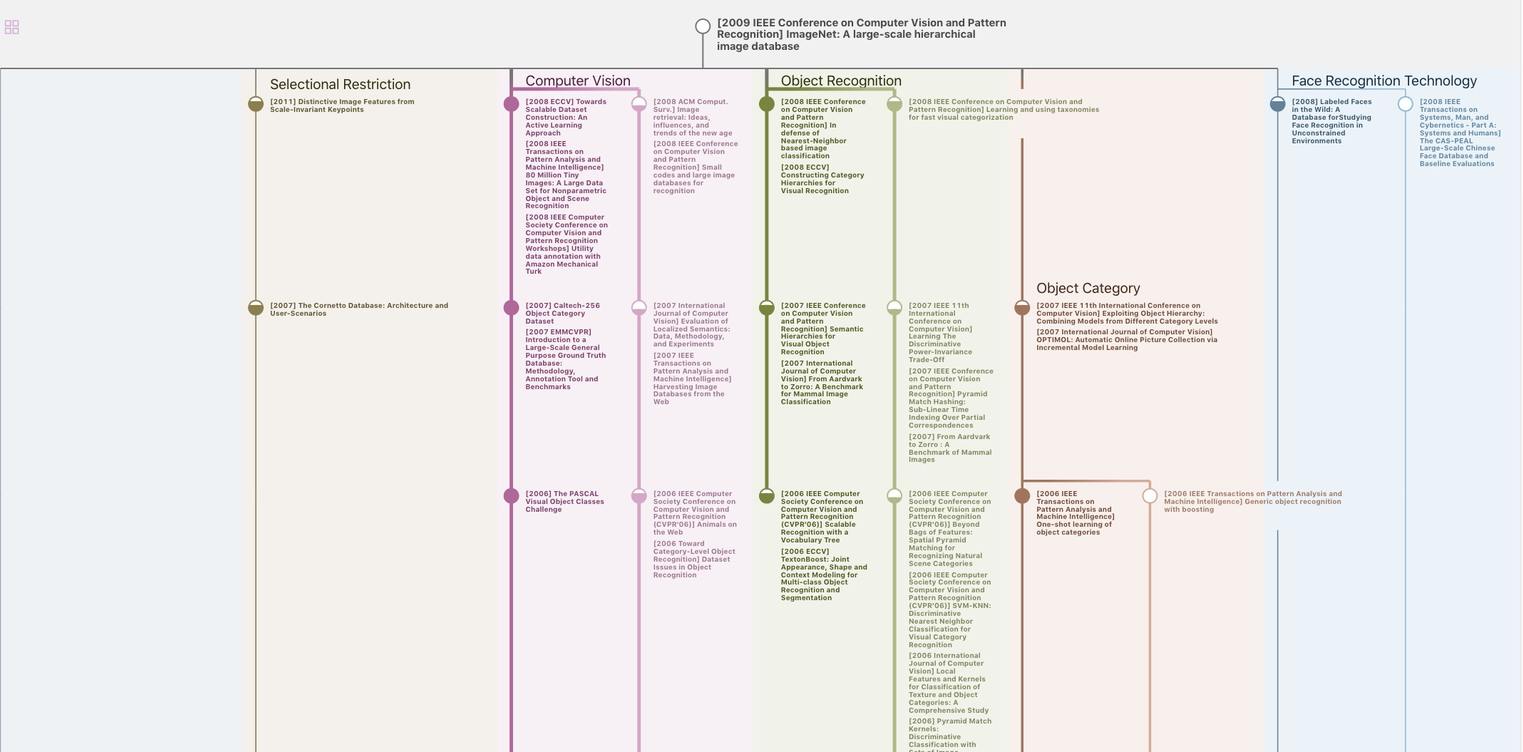
生成溯源树,研究论文发展脉络
Chat Paper
正在生成论文摘要