CNN and Transformer interaction network for hyperspectral image classification
INTERNATIONAL JOURNAL OF REMOTE SENSING(2023)
摘要
Convolutional Neural Network (CNN) has developed hyperspectral image (HSI) classification effectively. Although many CNN-based models can extract local features in HSI, it is difficult for them to extract global features. With its ability to capture long-range dependencies, Transformer is gradually gaining prominence in HSI classification, but it may overlook some local details when extracting features. To address these issues, we proposed a CNN and transformer interaction network (CTIN) for HSI classification. Firstly, A dual-branch structure was constructed in which CNN and Transformer are arranged in parallel to simultaneously extract global features and local features in HSI. Secondly, a feature interaction module has been imported between the two branches, thus facilitating a bi-directional flow of information between the global and local feature spaces. In this way, the network structure combines the advantages of CNN and Transformer in extracting features as much as possible. In addition, a token generation method is designed to harness abundant contextual information that is relevant to the centre pixel, and improve the accuracy of the final classification. Experiments were conducted on four hyperspectral datasets (two classical datasets - Indian Pines, Salinas Valley, a new satellite dataset - Yellow River, and an self-made UAV dataset-Yellow River Willow). Experimental results show that the proposed method outperforms the other state-of-the-art methods, with overall accuracies of 99.21%, 99.61%, 92.40%, and 98.17%, respectively.
更多查看译文
关键词
hyperspectral image classification,transformer interaction network,cnn
AI 理解论文
溯源树
样例
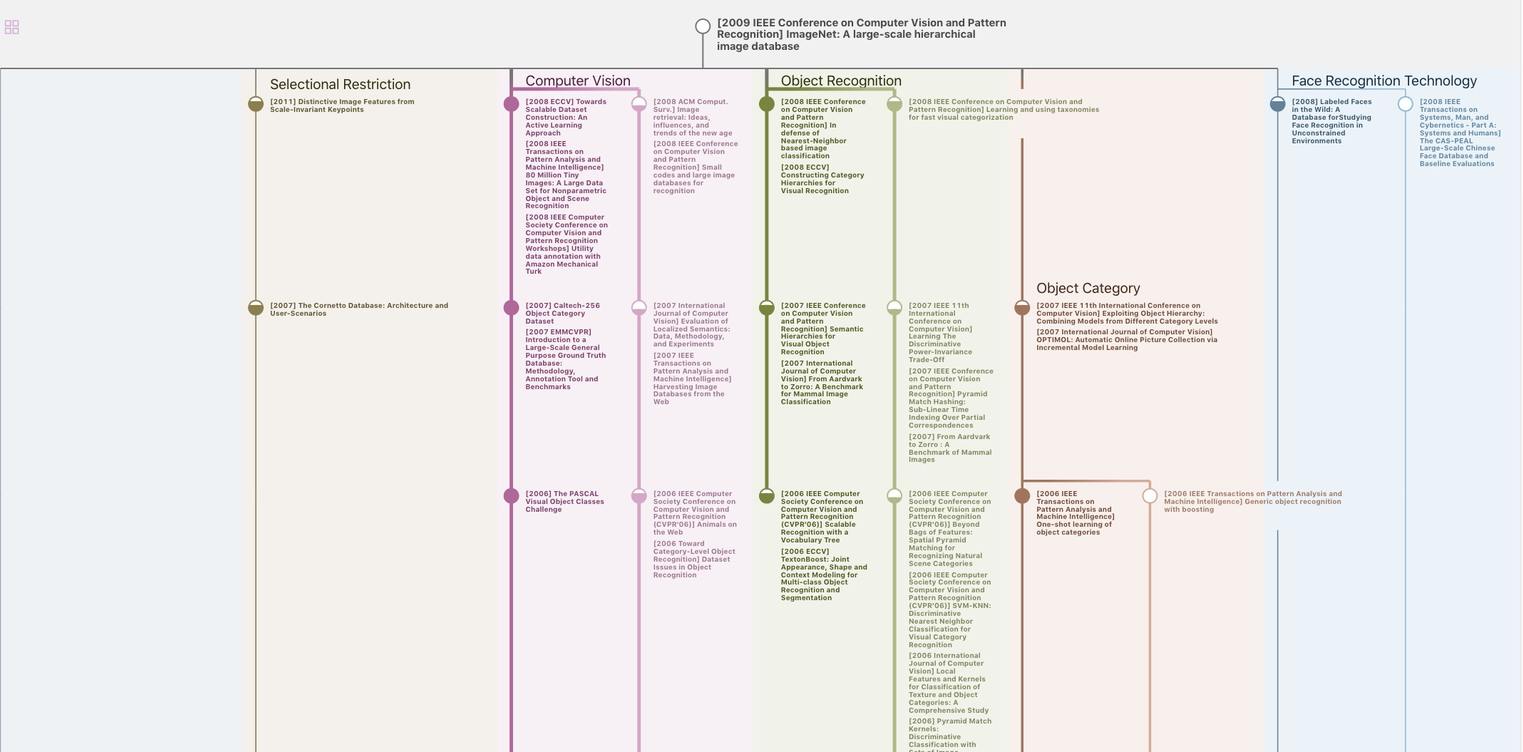
生成溯源树,研究论文发展脉络
Chat Paper
正在生成论文摘要