Attribute-sensitive community search over attributed heterogeneous information networks
EXPERT SYSTEMS WITH APPLICATIONS(2024)
摘要
Community search (CS) over attributed heterogeneous information networks (AHINs) takes both network structure and attributes of nodes into consideration, which can support personalized requirement and has gained great interest recently. Nevertheless, existing works are insensitive to the attributes that results from two limitations: (1) The structural cohesiveness metrics is insensitive to the slight quantity changes of nodes in a community. (2) The measurement adopted to measure the relevance between a community and given keywords is inappropriate. Additionally, the insensitivity may also lead to the free rider effect. To deal with these issues, in this paper, we first define structural cohesiveness by using the minimum number of neighbor nodes connected to each node in the community through a meta-path, then define keyword density with the path instances containing the given keywords, afterwards integrate both of them to propose an attribute-sensitive community model ASC that is sensitive to the attributes, and present the CS problem. In order to search community, we first propose a basic CS algorithm, then develop two index based CS algorithms to improve the efficiency, and theoretically prove that these two index based algorithms are more efficient than the basic algorithm. Comprehensive experiments conducted on five real-world datasets demonstrate the effectiveness of ASC and the efficiency of the developed algorithms.
更多查看译文
关键词
Attributed heterogeneous information networks,Attribute-sensitive community search,Structural cohesiveness,Keyword density,Node index,Attribute index
AI 理解论文
溯源树
样例
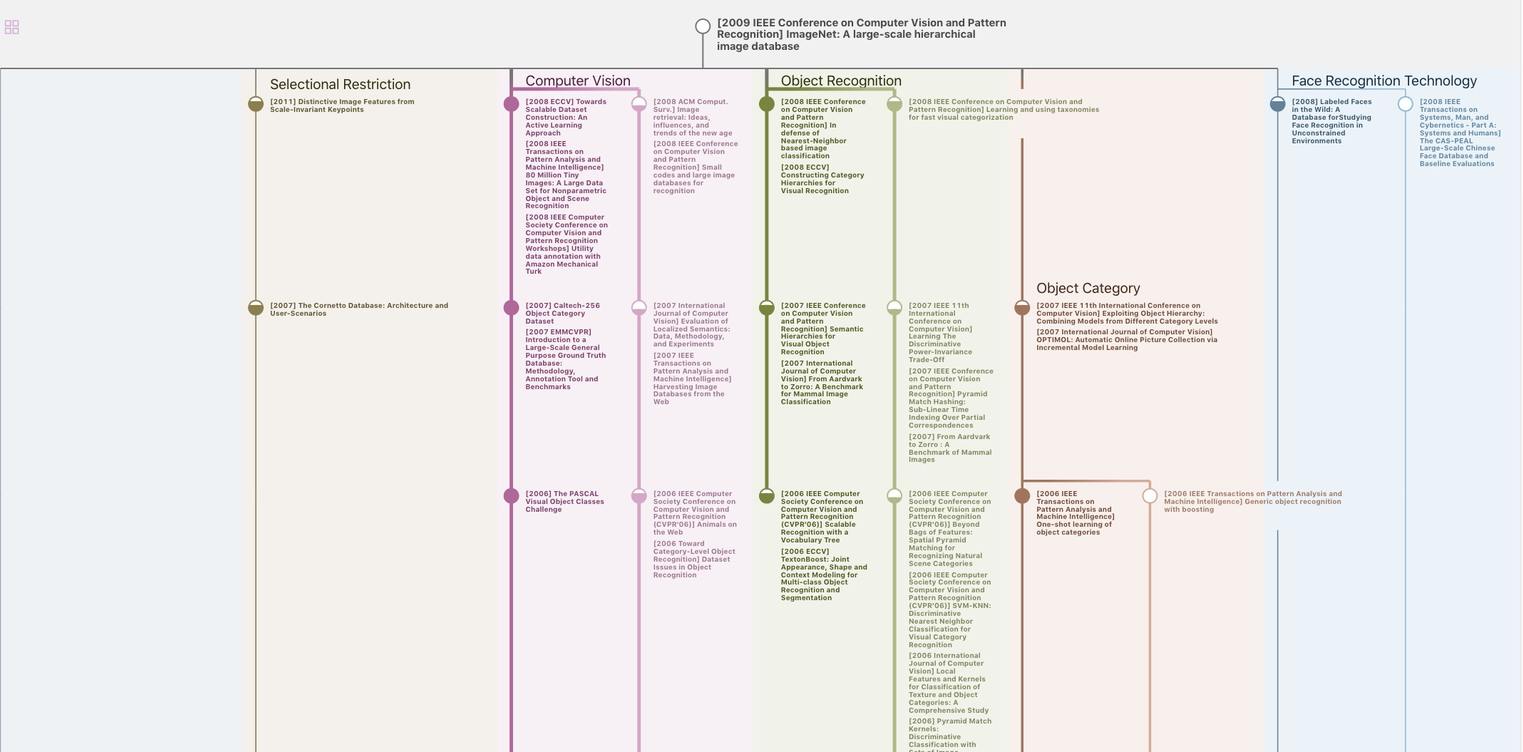
生成溯源树,研究论文发展脉络
Chat Paper
正在生成论文摘要