An improved two-archive artificial bee colony algorithm for many-objective optimization
EXPERT SYSTEMS WITH APPLICATIONS(2024)
摘要
Artificial bee colony (ABC) algorithm has shown good performance on many optimization problems. However, these problems mainly focus on single-objective and ordinary multi-objective optimization problems (MOPs). For many-objective optimization problems (MaOPs), ABC encounters some difficulties. The selection pressure based on Pareto-dominance degrades severely. It is hard to balance convergence and population diversity. To help ABC solve MaOPs, this paper proposes an improved two-archive many-objective ABC (called MaOABC-TA) algorithm. Inspired by the improved two-archive (Two_Arch2) method, MaOABC-TA uses two archives namely convergence archive (CA) and diversity archive (DA) to promote convergence and diversity. Based on CA and DA, three different search strategies are designed to strengthen convergence or diversity in different search stages. In addition, a new probability selection strategy is proposed to choose solutions with good diversity. To verify the performance of MaOABC-TA, it is compared with 10 many-objective evolutionary algorithms (MaOEAs) and 3 many-objective ABCs on DTLZ and MaF benchmark sets with 3, 5, 8, and 15 objectives. Two performance indicators including inverted generational distance (IGD) and hypervolume (HV) and utilized. Experimental results show that MaOABC-TA is more competitive than the compared algorithms in term of the IGD and HV values.
更多查看译文
关键词
Swarm intelligence,Artificial bee colony,Many-objective optimization,Two-archive,Multiple search strategies
AI 理解论文
溯源树
样例
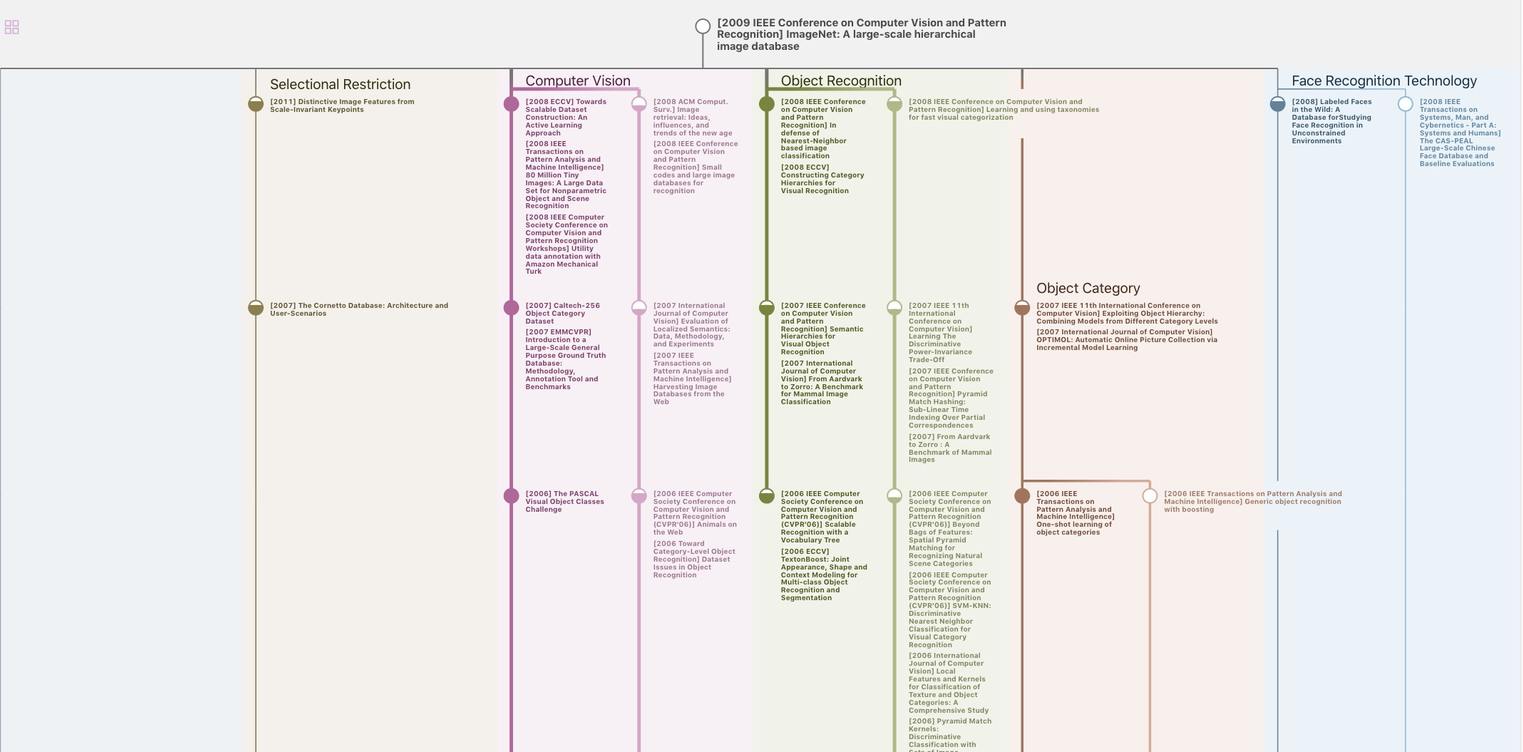
生成溯源树,研究论文发展脉络
Chat Paper
正在生成论文摘要