Machine learning models for chronic kidney disease diagnosis and prediction
BIOMEDICAL SIGNAL PROCESSING AND CONTROL(2024)
摘要
Background and objective: Chronic kidney disease is a severe health problem that affects people all over the world, particularly in South Asia. Therefore, proper diagnosis and treatment are required as early as possible. The main goal of this study is to detect the presence or absence of CKD in the human body utilizing a variety of features grasped from a few medical tests. Methods: This paper has focused on eight ensemble learning methods for diagnosing CKD on the UCI machine learning datasets. The datasets have been fixed by imputing the missing values using the MICE imputation method and handling the imbalance properties using the borderline SVMSMOTE method to improve the performance of classifiers. Moreover, recursive feature elimination and the boruta method have been used to find the most significant features and reduce the compilation time, while the hyperparameter tuning technique was used to raise the performance of classifiers and get optimal solutions. Results: Taking the most significant feature into consideration, RFE outperformed boruta and selected only 50% of the total features. Moreover, various performance matrices are used to find the best competent classifiers for detecting CKD. LightGBM outperformed state-of-the-art and other ensemble methods with the lowest compilation time and highest accuracy. Based on experimental findings, the proposed method achieved the highest average of 99.75% accuracy, 99.40% precision, 99.41% recall, 99.61% F-measure and 99.57% AUC-ROC. Moreover, our proposed method rises the average detection rate by 5.64%, 1%, 2.04%, 8.63%,1.99%, 2.84%, 2.42% and 4.76%, respectively, in comparison with different approaches performing on the same dataset. Conclusion: Experiments show that our suggested method can identify CKD more precisely than the most recent methods.
更多查看译文
关键词
Chronic kidney disease,Ensemble machine learning,Borderline-SMOTE,MICE,Prediction
AI 理解论文
溯源树
样例
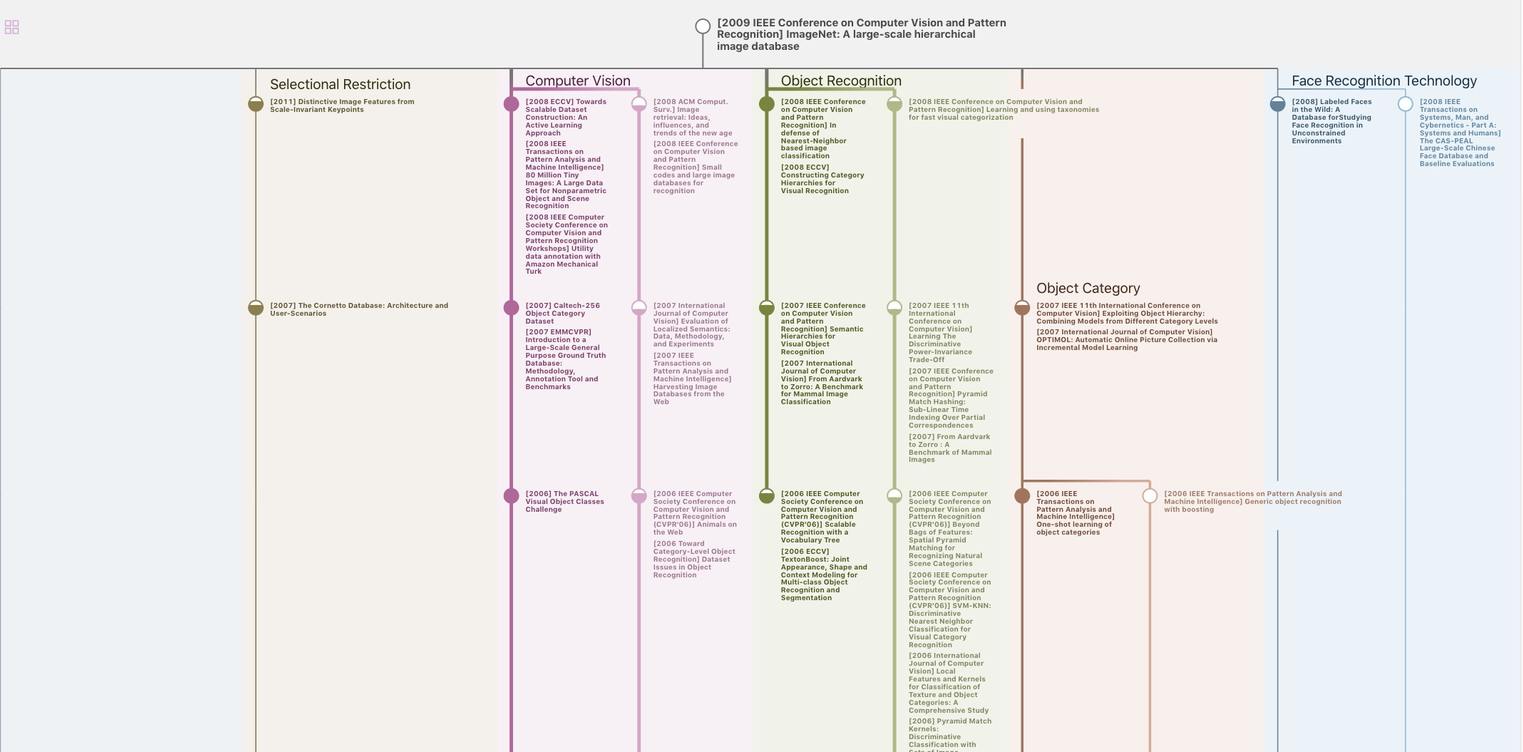
生成溯源树,研究论文发展脉络
Chat Paper
正在生成论文摘要