Optimizing intrusion detection in industrial cyber-physical systems through transfer learning approaches
COMPUTERS & ELECTRICAL ENGINEERING(2023)
摘要
Applications of Cyber-Physical Systems (CPSs) greatly influenceseveral industrial sectors. Treat-ing security-related concerns with utmost seriousness is necessary for the CPS to work correctly. Although CPS supervises the manufacturing process, the type and volume of cyberattacks that try to obtain data from CPS are significantly increasing. Since attacks on CPS can disrupt production, cause financial losses, and endanger national security, they must be prevented and detected. The general operation of the physical process can nevertheless be affected, and system failure is caused by specific traditional measures designed to anticipate CPS cyber-attacks. Also, as the system appears to be extremely complicated and no pertinent information about the item under investigation is available, the productive prediction of cyber-attacks in CPS remains a complex problem. This work will handle these issues using the proposed framework using Transfer Learning with the VGG16 model. The proposed TL-VGG16 achieves 96% accuracy, higher than existing CPS intrusion detection techniques.
更多查看译文
关键词
Deep learning,Attack detection,CPS,VGG16,Intrusion,Transfer learning,Detection accuracy
AI 理解论文
溯源树
样例
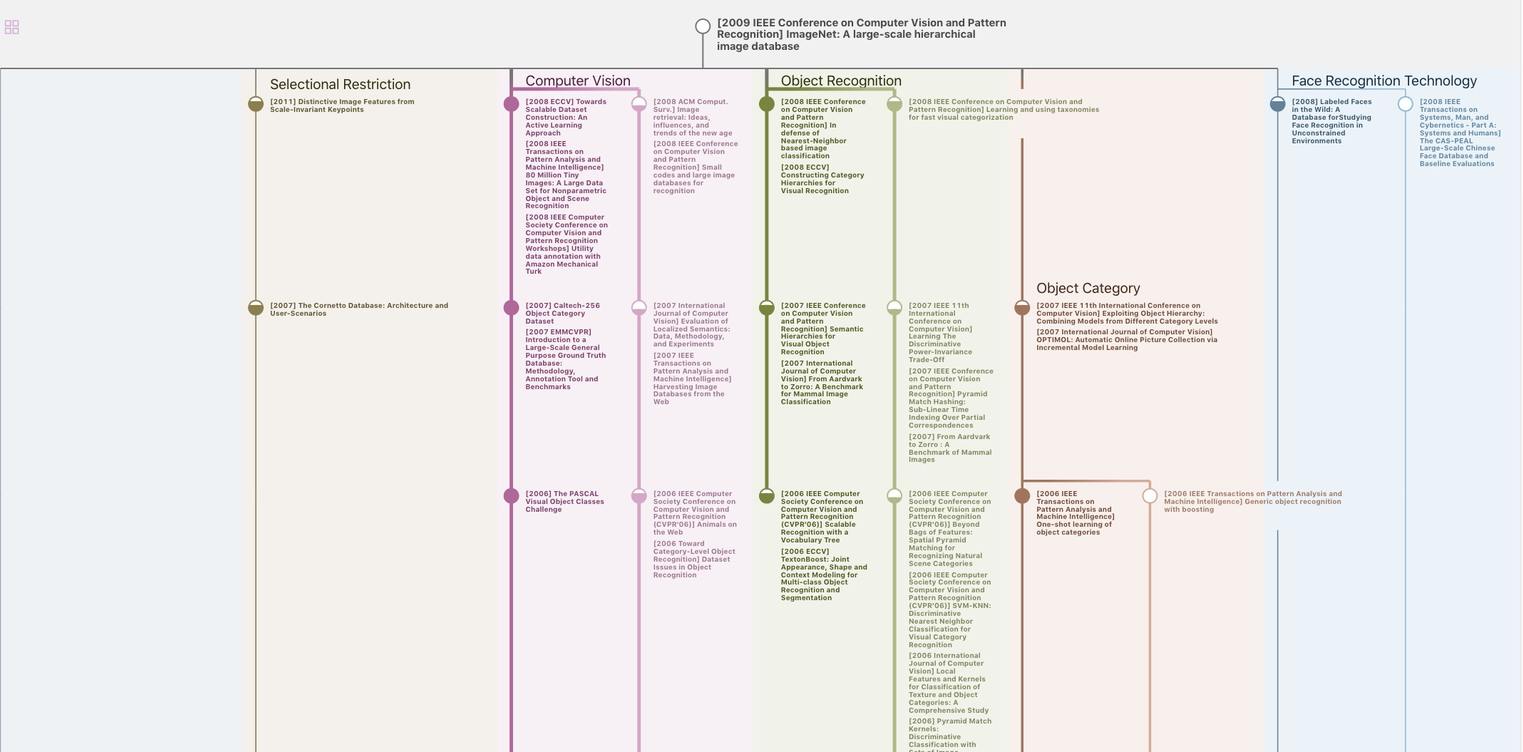
生成溯源树,研究论文发展脉络
Chat Paper
正在生成论文摘要