A fusion-attention swin transformer for cardiac MRI image segmentation
IET IMAGE PROCESSING(2024)
摘要
For semantic segmentation of cardiac magnetic resonance image (MRI) with low recognition and high background noise, a fusion-attention Swin Transformer is proposed based on cognitive science and deep learning methods. It has a U-shaped symmetric encoding-decoding structure with an attention-based skip connection. The encoder realizes self-attention for deep feature representation and the decoder up-samples global features to the corresponding input resolution for pixel-level segmentation. By introducing a skip connection between the encoder and decoder based on fusion attention, the remote interaction of global information is realized, and the attention to local features and specific channels is enhanced. A public ACDC cardiac MRI image dataset is used for experiments. The segmentation of the left ventricle, right ventricle, and myocardial layer is realized. The method performs well on a small sample dataset, for example, the pixel accuracy obtained by the proposed model is 93.68%, the Dice coefficient is 92.28%, and HD coefficient is 11.18. Compared with the state-of-the-art models, the segmentation precision has been significantly improved, especially for the low recognition and heavily occluded targets. For semantic segmentation of cardiac magnetic resonance imaging images, a fusion-attention Swin Transformer is proposed based on cognitive science and deep learning methods. It has a U-shaped symmetric encoding-decoding structure with an attention-based skip connection.image
更多查看译文
关键词
biomedical magnetic resonance imaging,image segmentation,medical image processing
AI 理解论文
溯源树
样例
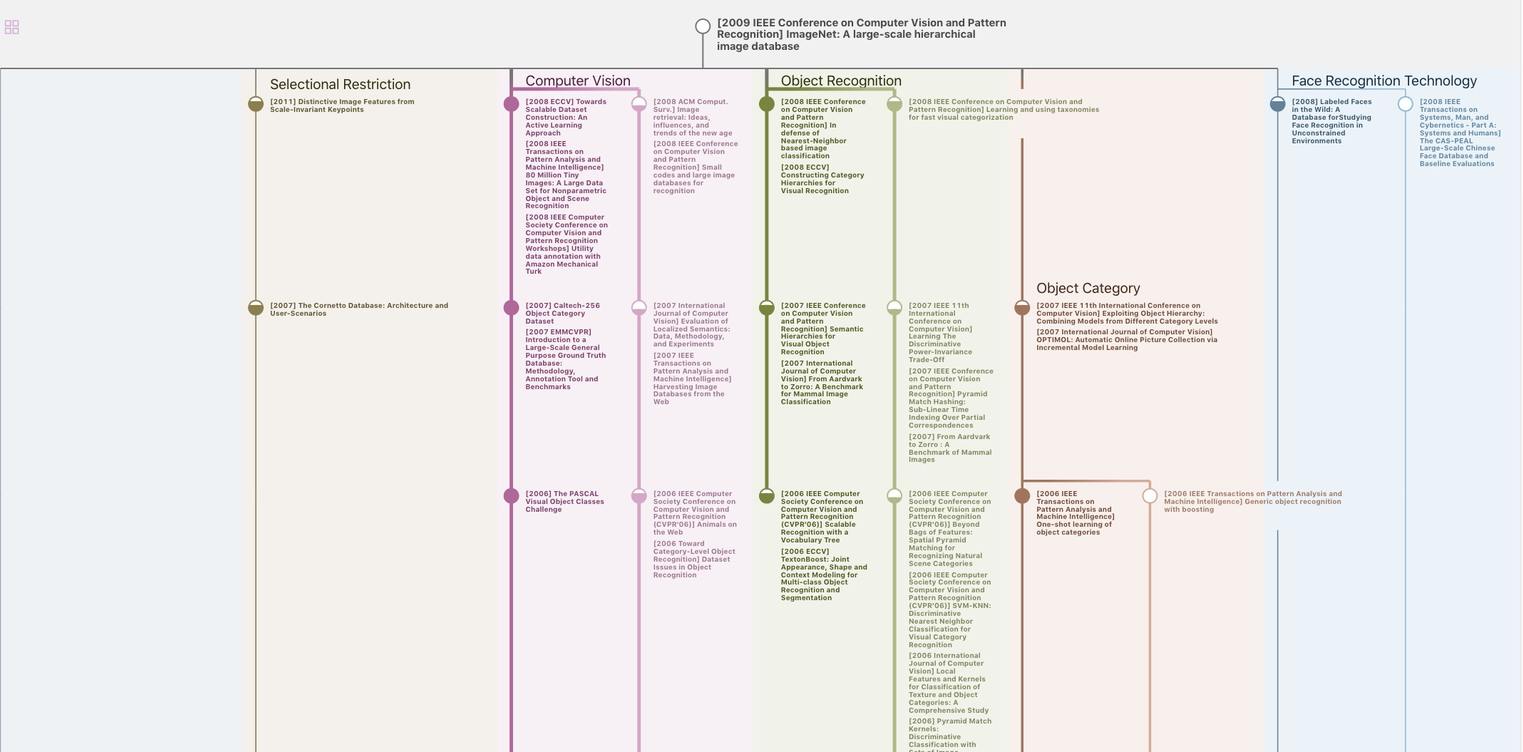
生成溯源树,研究论文发展脉络
Chat Paper
正在生成论文摘要