Optimal operational self-learning control for multi-time scale industrial with
ENGINEERING APPLICATIONS OF ARTIFICIAL INTELLIGENCE(2023)
摘要
Since the multi-time scales and strong nonlinearity are objectively existed in the practical industrial processes, it is extremely challenging to design control policy for optimizing the industrial operation. Therefore, this paper focuses on the multi-time scale optimal operational control (OOC) problem with unmodeled dynamics. Under the framework of reinforcement learning (RL) technology, a self-learning model-free method by integrating zero-sum game and singular perturbation (SP) theories is proposed in this paper, such that composite controllers with signal compensation can be found under which the suboptimal operation of the whole process can be achieved using only measured data. The remarkable highlight in this paper is the novel design method of the composite controllers with signal compensations for unknown nonlinear systems with multi-rate operations. Finally, the effectiveness of the proposed method is verified by a practical example and a numerical example.
更多查看译文
关键词
Optimal operational control, Reinforcement learning, Zero-sum game, Singular perturbation theory, Signal compensation
AI 理解论文
溯源树
样例
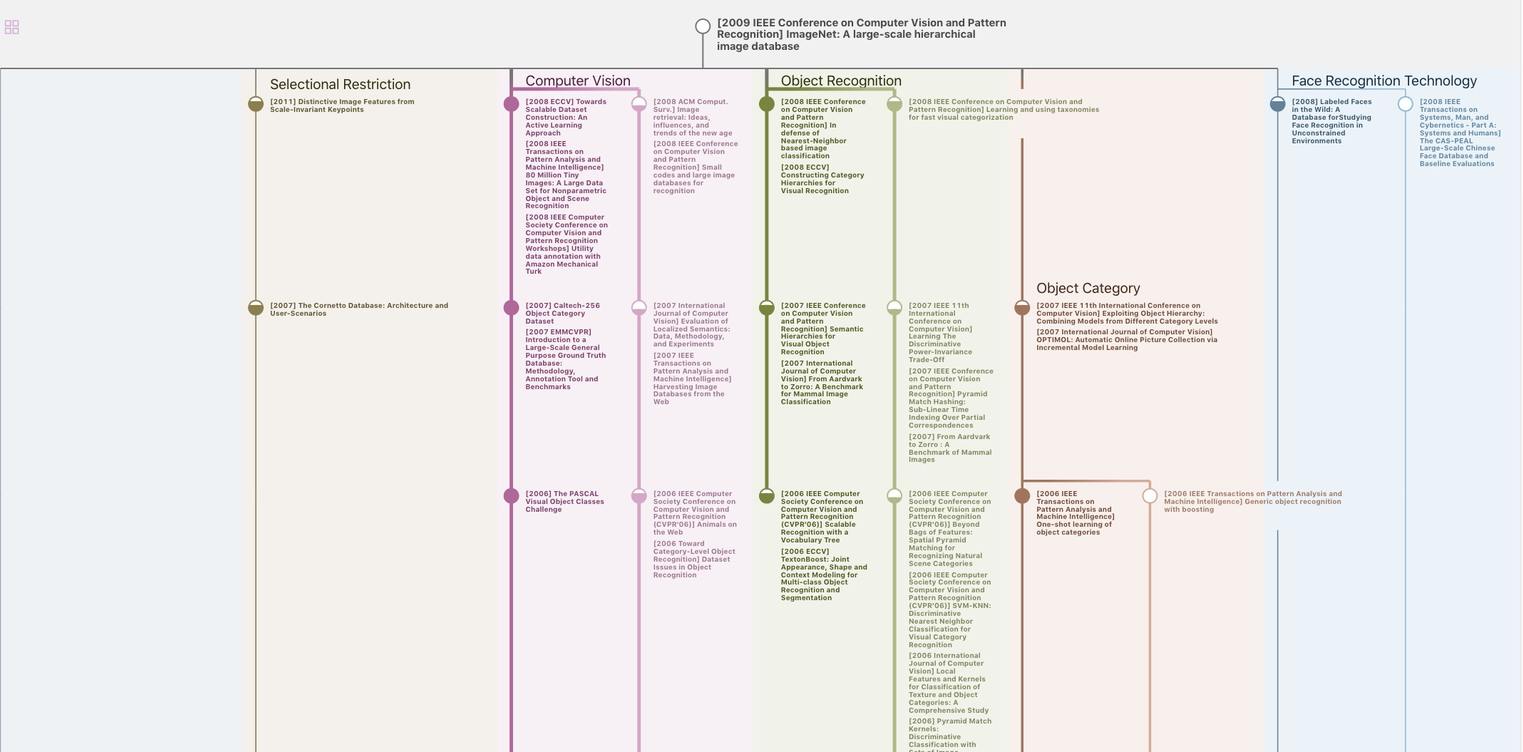
生成溯源树,研究论文发展脉络
Chat Paper
正在生成论文摘要