Fast fractional-order polar linear canonical transform: Theory and application
ENGINEERING APPLICATIONS OF ARTIFICIAL INTELLIGENCE(2023)
摘要
Zero-watermarking is a promising technology for image copyright protection due to its excellent imperceptibility and robustness. Compared with deep learning (DL)-based zero-watermarking schemes which are time-consuming and unable to respond in real-time, the moment-based zero-watermarking schemes do not have the aforementioned drawbacks and have drawn great attention with their strong description ability and geometric invariance. However, most existing moment-based zero-watermarking methods bear a number of drawbacks, in particular: (i) Direct computation of image moments is inefficient and inaccurate; (ii) They have difficulty obtaining the favorable trade-off between robustness and discriminability; (iii) Generating pseudo-random numbers based on traditional logistic map results in their lower security performance. To deal with those issues, this paper introduces a new image zero-watermarking method that relies on fast fractional-order polar linear canonical transform (FFrPLCT) features and asymmetric tent map. Firstly, we propose a novel computation strategy called fast polar linear Canonical transform (FPLCT), to save the calculation time of moments. Secondly, we define the fast fractional-order polar linear Canonical transform (FFrPLCT), which is characterized by the generic nature and time-frequency analysis capability. Thirdly, we present the FFrPLCT mixed low-order features by fully exploiting the time-frequency property of FFrPLCT, to improve both the robustness and discriminability of image representation. Finally, we develop a new image zero-watermarking method using FFrPLCT domain mixed low order features and asymmetric tent map. Experimental results and comparison with well-known existing methods demonstrate the efficacy and superiority of the proposed image zero-watermarking scheme.
更多查看译文
关键词
Zero-watermarking,FFrPLCT,Time-frequency analysis,Mixed low-order features,Asymmetric tent map,Robustness,Discriminability
AI 理解论文
溯源树
样例
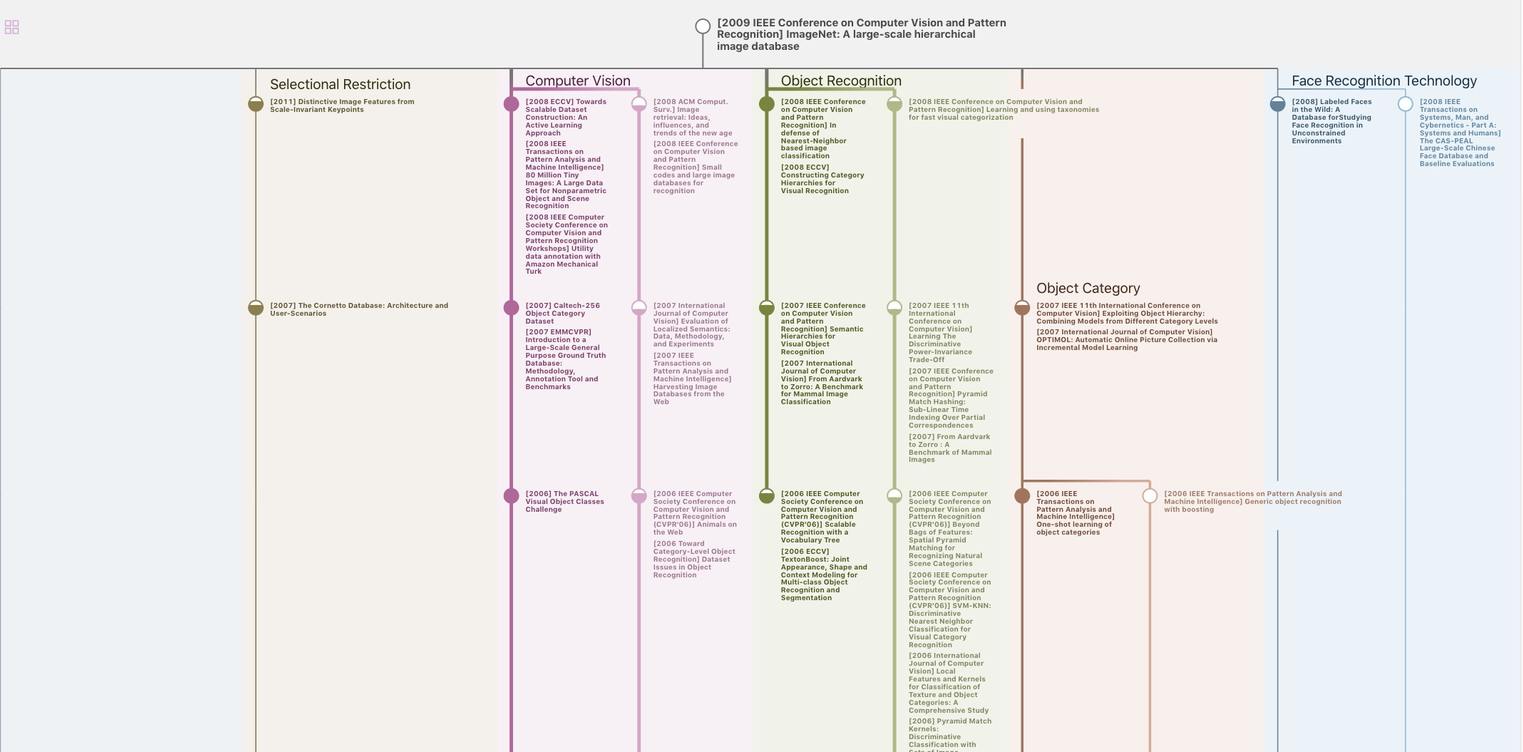
生成溯源树,研究论文发展脉络
Chat Paper
正在生成论文摘要