On Smartly Scanning of the Internet of Things
IEEE-ACM TRANSACTIONS ON NETWORKING(2023)
摘要
Cyber search engines, such as Shodan and Censys, have gained popularity due to their strong capability of indexing the Internet of Things (IoT). They actively scan and fingerprint IoT devices for unearthing IP-device mapping. Because of the large address space of the Internet and the mapping's mutative nature, efficiently tracking the evolution of IP-device mapping with a limited budget of scans is essential for building timely cyber search engines. An intuitive solution is to use reinforcement learning to schedule more scans to networks with high churn rates of IP-device mapping. However, such an intuitive solution has never been systematically studied. In this paper, we take the first step toward demystifying this problem based on our experiences in maintaining a global IoT scanning platform. Inspired by the measurement study of large-scale real-world IoT scan records, we land reinforcement learning onto a system capable of smartly scanning IoT devices in a principled way. We disclose key parameters affecting the effectiveness of different scanning strategies, and real-world experiments demonstrate that our system can scan up to around 40 times as many IP-device mapping mutations as random/sequential scanning.
更多查看译文
关键词
Internet of Things (IoT),Adaptive algorithms,IP networks,Cyberspace
AI 理解论文
溯源树
样例
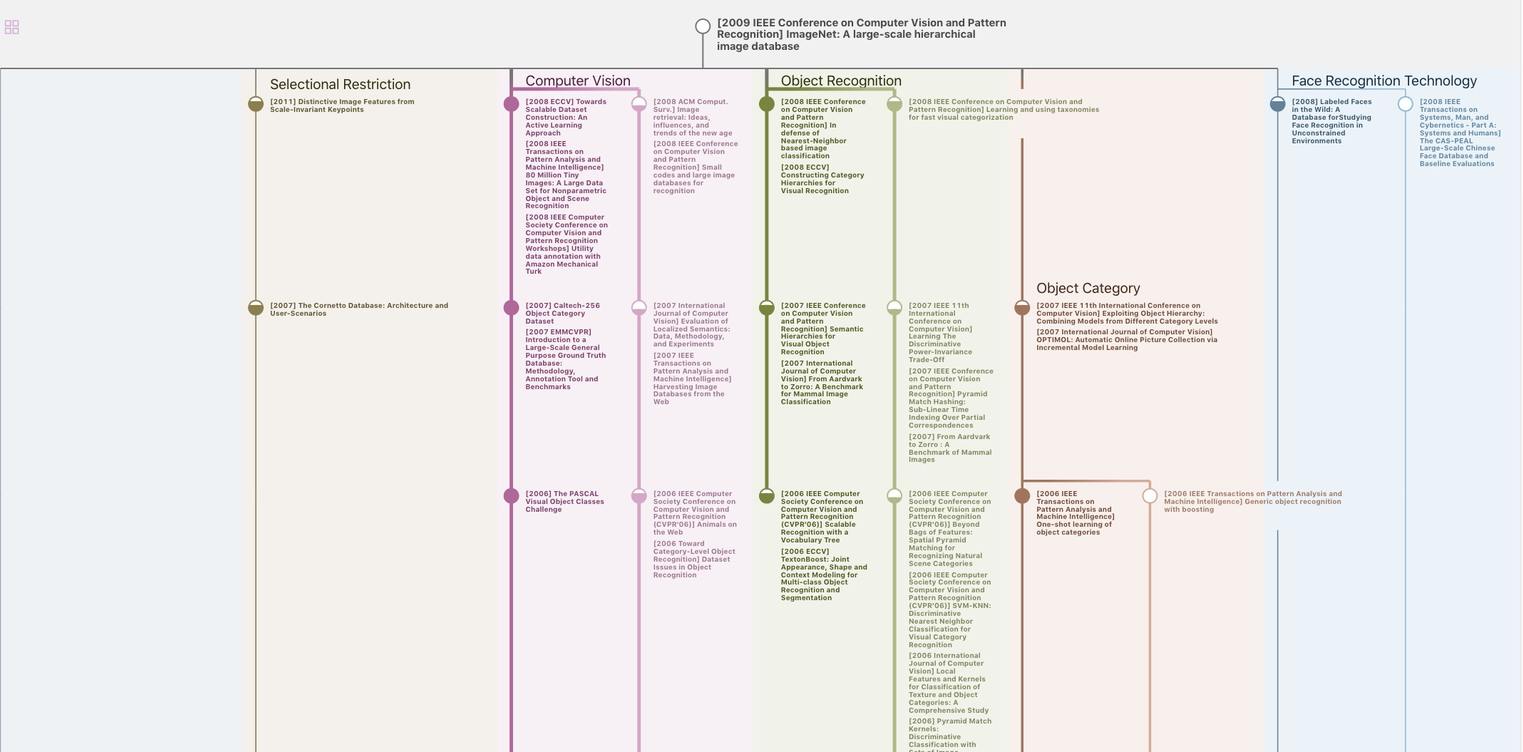
生成溯源树,研究论文发展脉络
Chat Paper
正在生成论文摘要