Visible-infrared person re-identification using high utilization mismatch amending triplet loss
IMAGE AND VISION COMPUTING(2023)
摘要
Visible-infrared person re-identification (VIPR) is a task of retrieving a specific pedestrian monitored by cameras in different spectra. A dilemma of VIPR is how to reasonably use intra-modal pairs. Fully discarding intra-modal pairs causes a low utilization of training data, while using intra-modal pairs brings a danger of distracting a VIPR model's concentration on handling cross-modal pairs, harming the cross-modal similarity metric learning. For that, a high utilization mismatch amending (HUMA) triplet loss function is proposed for VIPR. The key of HUMA is the multi-modal matching regularization (MMMR), which restricts variations of distance matrices calculated from cross- and intra-modal pairs to cohere cross- and intra-modal similarity metrics, allowing for a high utilization of training data and amending the adverse distractions of intra-modal pairs. In addition, to avoid the risk of harming feature discrimination caused by MMMR preferring coherence in similarity metrics, a novel separated loss function assignment (SLFA) strategy is designed to arrange MMMR well. Experimental results show that the proposed method is superior to state-of-the-art approaches.
更多查看译文
关键词
Modal-mismatch,Triplet loss,Visible-infrared person re-identification,Intelligent video surveillance
AI 理解论文
溯源树
样例
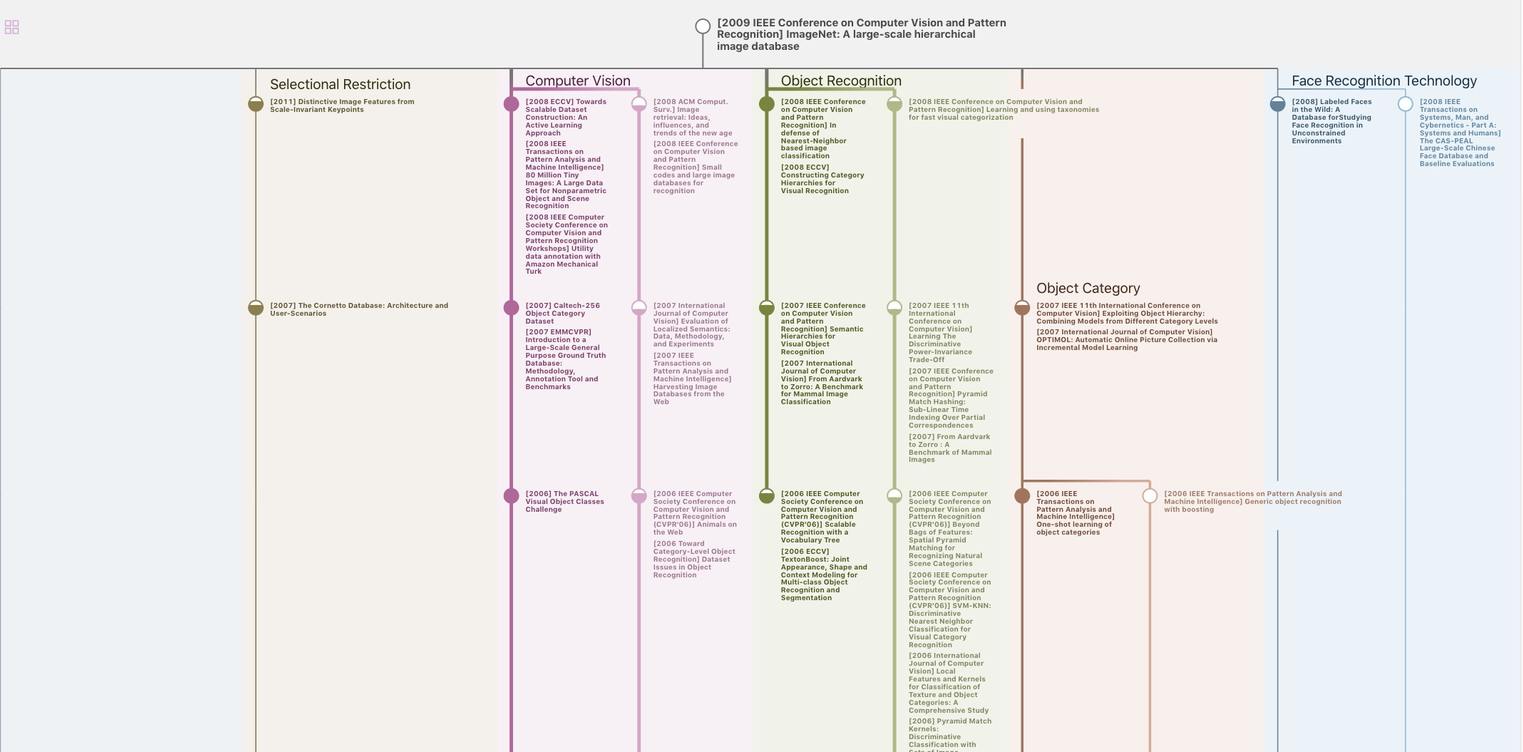
生成溯源树,研究论文发展脉络
Chat Paper
正在生成论文摘要